Open Access
ARTICLE
Optimal Deep Transfer Learning Based Colorectal Cancer Detection and Classification Model
1 Information Technology Department, Faculty of Computing and Information Technology, King Abdulaziz University, Jeddah, 21589, Saudi Arabia
2 Center for Artificial Intelligence in Precision Medicines, King Abdulaziz University, Jeddah, 21589, Saudi Arabia
3 Department of Mathematics, Faculty of Science, Al-Azhar University, Naser City, 11884, Cairo, Egypt
4 Cancer Biology Unit, King Fahd Medical Research Center, King Abdulaziz University, Jeddah, 21589, Saudi Arabia
5 Department of Medical Laboratory Sciences, Faculty of Applied Medical Sciences, King Abdulaziz University, Jeddah, 22252, Saudi Arabia
6 Department of Molecular Genetics and Enzymology, Human Genetics and Genome Research Institute, National Research Centre, Cairo, 12622, Egypt
7 Biochemistry Department, Faculty of Science, King Abdulaziz University, Jeddah, 21589, Saudi Arabia
8 Cell Biology Department, Biotechnology Research Institute, National Research Centre, Giza, 12622, Egypt
* Corresponding Author: Mahmoud Ragab. Email:
Computers, Materials & Continua 2023, 74(2), 3279-3295. https://doi.org/10.32604/cmc.2023.031037
Received 08 April 2022; Accepted 20 May 2022; Issue published 31 October 2022
Abstract
Colorectal carcinoma (CRC) is one such dispersed cancer globally and also prominent one in causing cancer-based death. Conventionally, pathologists execute CRC diagnosis through visible scrutinizing under the microscope the resected tissue samples, stained and fixed through Haematoxylin and Eosin (H&E). The advancement of graphical processing systems has resulted in high potentiality for deep learning (DL) techniques in interpretating visual anatomy from high resolution medical images. This study develops a slime mould algorithm with deep transfer learning enabled colorectal cancer detection and classification (SMADTL-CCDC) algorithm. The presented SMADTL-CCDC technique intends to appropriately recognize the occurrence of colorectal cancer. To accomplish this, the SMADTL-CCDC model initially undergoes pre-processing to improve the input image quality. In addition, a dense-EfficientNet technique was employed to extract feature vectors from the pre-processed images. Moreover, SMA with Discrete Hopfield neural network (DHNN) method was applied for the recognition and classification of colorectal cancer. The utilization of SMA assists in appropriately selecting the parameters involved in the DHNN approach. A wide range of experiments was implemented on benchmark datasets to assess the classification performance. A comprehensive comparative study highlighted the better performance of the SMADTL-CDC model over the recent approaches.Keywords
Cite This Article
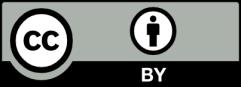
This work is licensed under a Creative Commons Attribution 4.0 International License , which permits unrestricted use, distribution, and reproduction in any medium, provided the original work is properly cited.