Open Access
ARTICLE
Fuzzy-HLSTM (Hierarchical Long Short-Term Memory) for Agricultural Based Information Mining
1 Department of Computer Science, College of Science and Arts, Sharurah, Najran University, Najran, Saudi Arabia
2 Department of Computer Science, College of Computer Science & Information Systems, Najran University, Najran, Saudi Arabia
3 Computer Skills Department, Deanship of Preparatory Year, Najran University, Najran, Saudi Arabia
* Corresponding Author: Ahmed Abdu Alattab. Email:
Computers, Materials & Continua 2023, 74(2), 2397-2413. https://doi.org/10.32604/cmc.2023.030924
Received 06 April 2022; Accepted 26 May 2022; Issue published 31 October 2022
Abstract
This research proposes a machine learning approach using fuzzy logic to build an information retrieval system for the next crop rotation. In case-based reasoning systems, case representation is critical, and thus, researchers have thoroughly investigated textual, attribute-value pair, and ontological representations. As big databases result in slow case retrieval, this research suggests a fast case retrieval strategy based on an associated representation, so that, cases are interrelated in both either similar or dissimilar cases. As soon as a new case is recorded, it is compared to prior data to find a relative match. The proposed method is worked on the number of cases and retrieval accuracy between the related case representation and conventional approaches. Hierarchical Long Short-Term Memory (HLSTM) is used to evaluate the efficiency, similarity of the models, and fuzzy rules are applied to predict the environmental condition and soil quality during a particular time of the year. Based on the results, the proposed approaches allows for rapid case retrieval with high accuracy.Keywords
Cite This Article
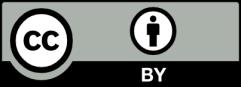
This work is licensed under a Creative Commons Attribution 4.0 International License , which permits unrestricted use, distribution, and reproduction in any medium, provided the original work is properly cited.