Open Access
ARTICLE
Ontological Model for Cohesive Smart Health Services Management
1 Le Laboratoire Génie de Production de l’École Nationale d’Ingénieurs de Tarbes (LGP-INP-ENIT), Université de Toulouse, CEDEX, Tarbes, 65016, France
2 Department of Software Engineering, Superior University, Lahore, 53700, Pakistan
3 Department of Informatics & Systems, University of Management & Technology, Lahore, 54000, Pakistan
4 Department of Computer Science, University of Management and Technology, Sialkot, 51310, Pakistan
5 Department of Computer Science, King Abdul-Aziz University, Jeddah, 21577, Saudi Arabia
6 Faculty of Computing and Information Technology, King Abdulaziz University, Jeddah, 21577, Saudi Arabia
* Corresponding Author: Muhammad Waseem Iqbal. Email:
Computers, Materials & Continua 2023, 74(2), 3679-3695. https://doi.org/10.32604/cmc.2023.030340
Received 24 March 2022; Accepted 29 May 2022; Issue published 31 October 2022
Abstract
Health care has become an essential social-economic concern for all stakeholders (e.g., patients, doctors, hospitals etc.), health needs, private care and the elderly class of society. The massive increase in the usage of health care Internet of things (IoT) applications has great technological evolvement in human life. There are various smart health care services like remote patient monitoring, diagnostic, disease-specific remote treatments and telemedicine. These applications are available in a split fashion and provide solutions for variant diseases, medical resources and remote service management. The main objective of this research is to provide a management platform where all these services work as a single unit to facilitate the users. The ontological model of integrated healthcare services is proposed by getting requirements from various existing healthcare services. There were 26 smart health care services and 26 smart health care services to classify the knowledge-based ontological model. The proposed ontological model is derived from different classes, relationships, and constraints to integrate health care services. This model is developed using Protégé based on each interrelated/correlated health care service having different values. Semantic querying SPARQL protocol and RDF query language (SPARQL) were used for knowledge acquisition. The Pellet Reasoner is used to check the validity and relations coherency of the proposed ontology model. Comparative to other smart health care services integration systems, the proposed ontological model provides more cohesiveness.Keywords
IoT is a concept of a vast network composed of any object that can be inserted into any location to some other place for storage and analysis. The sensors and things in IoT intelligently gather essential information processed and examined for enhanced decision-making. This permits the intelligent objects to link with each other and with nearby networks to send calculation-based performance [1]. Nowadays, IoT technology is primarily seen in industries and commercial sectors. The interconnected divergent kinds of intelligent gadgets vary from simple wearable devices, household devices, and appliances to large machines. These objects contain chips that are used to inspect and pursue facts. This technology is used in many intelligent projects, i.e., intelligent cities projects, innovative farming projects, and smart homes and this technology is also used in health care [2,3].
With the increase in diverse smart innovative applications, IoT has a changed our lives. IoT enabled health care is such a vital IoT application that links intelligent devices, and different stakeholders to the internet. IoT in health care systems robust has a critical role in serving comfort to doctors and patients. The input data from patients can be gathered with the help of sensors [4]. This data is processed by applications made for a user terminal, such as computers, smartphones or even a particular embedded device. Such a solution empowers sensors to gather detailed bodily information and use kind-collect physical the cloud to examine and store the data and then transmit the processed sensor’s data to cloud storage and analyses data interoperable to caregivers for further review [5]. Robust wireless solutions linked with the IoT. This type of application assists in monitoring the person suffering from chronic disease [6].
For especially people living in rural areas, IoT health care can be of much assistance as they do not have adequate medical services side assistance to help adequate good. Because they have to take a trip of miles to visit the doctor, they may start overlooking their health issues [7]. Remotely personal monitoring of the patients lessens reliance on the doctors and sustains quality of life-preserving privacy of patient data. Due to this ease of communication, one can save himself from the wastage of time, especially in situations and emergencies. A medical expert can also help in the hours of need as much as possible. The revolution of health care, progressing from conventional health management to supplementary custom-made smart health care systems, benefits personal health.
1.1 Lacking in Smart Health Services Standardization
Smart health care Systems are hard to define formally because most systems lack standardization of architecture by doing a literature review of different IoT based healthcare systems. We have known shared concepts in other innovative health systems working in isolation. These shared concepts can be represented using ontologies, the help of which are not only able to define smart health care systems formally but also find the commonalities and differences among these systems. By finding the shared concepts between these systems and ontologies, we merge them into a cohesive health care management system. That leads us to the standardization of smart health care systems.
Recently, a variety of smart health care services have been available to facilitate the users in a diversity of interaction styles and modes on a separate individual basis. These distinct units of health care services create frustration by providing inadequate modules, specific remote patient features, limited diagnostic structures, poor treatment of diseases and high costs. These types lack the motivation to create a management platform of smart health care services where they work as a single unit to facilitate the users. Although, in the last few years, a lot of research has been done to reduce users in smart health care services. This research proposes an ontological model for cohesive smart healthcare services management smart innovation presented. This model is provided smart health care survives management platform in a single unit and is part of the continuation of the author’s previous work [8]. It is to keep all the context in terms of health care services properly to facilitate the users.
Ontologies arrange the items or concepts of data in such a way that it creates meaning among them. The vocabularies and taxonomies become more meaningful by using the relations and restrictions among the data terms and concepts. Therefore, by using ontologies, we can formally also represent particular domain’s concepts and allow automated reasoning among those concepts. Worldwide ontologies are used for defining terms in the field and relationships among them because ontologies are shared and ideas and provide a standardized solution. These defined concepts in the field are termed classes and arranged in a hierarchy in the form of sub-classes. Object properties and data properties are assigned to these classes.
Following is an overview of the previously done work in the domain of IoT health care applications as we categorized it into three parts such as i) disease based, ii) service based, and iii) resource based.
2.1 Disease Based IOHT Applications
2.1.1 Concurrent Heart Attack Mobile Recognition Service
Mobile edge computing (MEC) is an advanced architecture that uses cloud computing and mobile networking specifically for more minor non-functionality applications [9]. Software-defined networking (SDN) is a development groundwater model for network-driven programming, ensuring broad network flexibility and significantly saving, which provides overall costs. The SDN does not make restrictions on the physical structure, but to a specific extent, it can be programmed in an application [10]. Trustworthy testing, road traffic safety & monitoring, and better social connectivity are some of the system’s features. For organizations such networks, the Vehicular ad hoc network (VANET) framework is introduced [11]. VANET is a framework in which smart cards are shown as mobile car nodes in the vehicle’s network. This framework is not only used for communication between two vehicles cars but also for communication with Restricted stock units (RSU) and intelligent vehicles. Cardiovascular disease is a chronic illness that is one of the world’s most important health problems and is a significant cause of death across the globe. A proficient algorithm for heart attack detection is devised [12].
Elderly patients can be marked safe if Electrocardiogram (ECG) signal patterns are processed in time and action is taken whenever there seems to be any problem in the ECG signal. This system also uses machine learning methods for evaluating sensor data for heart attacks and predicts heart attacks. This system is helpful for the elderly patient monitoring and is also likely to detect heart disease in patients with adults, adolescents and heart disease patients or physical restoration patients.
2.1.2 Chronic Metabolic Disorders
Health IoT for personalized monitoring of patients with chronic metabolic illness is presented. Two types of patients are targeted in this system, i.e., diabetic patients and patients going through house dialysis. To analyze the patient’s health condition, Health IoT uses the disease manager, which applies and regulates various rules after processing data. Such laws have been developed under doctors’ instructions, and these rules should change according to each patient’s health records [13]. A novel framework based on IoT has been presented for blood glucose level tests in the body. This system has a non-obtrusive glucometer, a show framework that uses an Arduino microcontroller and mobile application (android) [14]. This system uses operational intensifiers, an Infrared light-emitting diode (IR LED), a photodiode and a Liquid crystal display (LCD) display. The glucose estimation kit and the circuit has been accumulated, and an application was expected to show the results.
Glucometer with IoT is presented [15] as the purpose of this system is to use blood glucose levels, which is painful for patients, rather than tobacco prices by optical and IT technology using non-technology. To achieve blood glucose levels, a specific dimension is used by glucose spectroscopy.
In this particular system, the mobile application is responsible for receiving and starting the processing of ECG signals. This device supports ECG signals received as another EGG cloud processing center segment. And the other actor is a medical staff who will see the patient’s ECG in case of any medical problem [16]. Discrete wavelet transformation (DWT) is used for the ECG signal preparation and investigation, and a Support vector machine (SVM) classifier eliminates the commotion in the ECG signal. After experimentation, the best classification accuracy is 98.9% for a feature vector of size 18 and 2493 support vectors. Various executions of the algorithm on the Galileo board help demonstrate that the computational budget is such that the ECG analysis and classification are real-time systems [17].
2.1.4 Smart Asthma Patients Care Using IoT
A respiratory observing system is developed. According to this system, respiratory rate is estimated on the temperature value. The LM35 temperature sensor is utilized. The respiratory rate is calculated using an LM35 temperature sensor and is observed by the patient’s respiration constantly based on the based continuously rate of inhaled and exhaled air. These sensors sense temperature values in near real-time by using an Arduino microcontroller. NRF24l01 is utilized for communicating the sensor’s data from home to a nearby medical Centre [18]. Then the data is circulated in a webserver by Ethernet wired connection to know the patient’s present condition, which is valuable for the doctor. Data is useful for the doctor also data is also displayed on an LCD monitor. If any unusual situation is observed, an alarm is initiated and generated, showing an emergency alarm message in the web browser. Then the data is then analyzed and processed by utilizing different data mining ways like data clustering and data classification to recognize the health status of patients without any outside help [19].
2.2 Service Based IOT Applications
For providing a harmless and safe healthy living to the elderly in a smart home, various sensors ensure behavioral and environmental conditions; this information is employed in addition to the health devices, used for necessary sign measurement. The aftereffect of the investigation is served to the formal and casual guardians to give them a choice emotionally supportive network. These aids convey protected environmental factors for older patients [20].
Checking constant sickness at home utilizing associated gadgets is conceivable. The point is to screen patients during their everyday life schedules to save and gather information continually. This sort of checking needs to manage three angles: people, climate and sensors. The Centre is to gain proficiency with the various relations and connections between factors coming from those perspectives. A significant piece of this work contemplates the individual’s enthusiastic attitude (stress, bliss, pity) and considers them as utilizing the appropriate computerized reasoning devices [21]. People suffering from chronic diseases need to monitor their vital signs intermittently. This framework ensures that high danger patients are opportunely checked and observed occasionally. By utilizing this model, the cost is diminished, and patients are very much aware of their condition without anyone else without the guidance of the medical caretaker [22]. Small heart rate variability (HRV) monitoring system dependent on the IoT innovation for negligible hypertensive patients is introduced. This framework, HRV boundaries are acquired using a wireless Zonal intercommunication global-standard (ZigBee) heartbeat sensor in this framework. Arduino moves the patient’s information to the worker using the Message queuing telemetry transport (MQTT) convention. The framework effectively attempts to screen and discern the hypertension state of the patients [23].
2.2.2 Remote Prescription and Telemedicine
Real-time clock, Radio frequency identification (RFID) and sensor tags are associated with the Raspberry Pi. Raspberry Pi receives readings from the sensors, and they can also be shown on the monitor. If the patient’s rare heart condition is not improved in a specific time interval, an alarm will send a message using SMS service to the doctor [24]. This medication box is of high technology. It operates on wireless transmission units; I-Med-Box is a trained medical examiner who monitors the daily intake of medicines of patients. A reader of a specific frequency, Wi-Fi, a Zig bee receiver, and a tablet having additional ports are implanted into the closure. A weight bridge sensor having a high resolution is joined in the bottom of the Intelligent medicine box (iMedBox) to keep an eye on the weight changes of the medication contained in the iMedBox, and based on this, the dose; the medicine intake of the patient can be estimated. Wearable medical sensors, e.g., Bio Patch, intelligent medicine packages, and other sensors/devices, can be attached to iMedBox by wireless technologies [25].
If the patients do not take their medicines regularly, they may potentially suffer from the diseases. There are some diseases like Blood Pressure, if not cured with treatments, which can become a cause of heart attack. Chronic illnesses like osteoporosis cannot be fixed by taking medicine once; if patients do not medico regularly, this might become harmful to their patient’s health. So, this issue needs to be addressed. For this purpose, an intelligent pillbox is introduced to help the patient follow their medicine intake as directed by the specialist. First, the smart pillbox is connected to the Internet by Wi-Fi. After this, a mobile application is launched for the patient and caregivers to check whether the patient has taken his daily dose of medicine or not [26]. A confirmed client can enter the patient’s data, the endorsed medication, time and time in every supper into the application and append headings about how every pill should be taken. For this reason, to be satisfied, the update and cautions are created. When the time has come to take their medication, a notice is set off on the portable application with a voice caution and vibrates, directing them to take medicine. If the patient takes their medication effectively, the framework doesn’t propel the alarm to work once more. The framework will regularly record the time and change the prescriptions’ state. Then again, if the weight of the jug isn’t changed, the framework will accept that the patient has not taken their medication and start the alarm work in the subsequent stage. In the wake of running the alarm work, if the weight changes are not spotted once more, the framework will change the status as not taken. However, if weight change is identified, the framework will record this weight and change the group as a pill taken [27].
The architecture is proposed for the telemedicine of diabetes management. This exhibits to collect of behavioral responses of patients with diabetes. This information is examined and refined to be presented to all the involved stakeholders. Thus, decisions are reinforced by the data. Visualization layer as mined data to patients and doctors. Based on this data, a prediction layer can be made to predict medicines depending upon behavioral data obtained randomly by different users. All stored information is maintained and examined to display and make suggestions to the doctors and patients [28].
2.3 Resource Based IOT Applications
2.3.1 Hospitalized Patient Monitoring
Bedridden patients under intense care must be checked very carefully, whether at the hospital or at home. Solutions based on IoT devices are devised, which help us save the costs of physical monitors by providing some Internet-connected services. This work describes the integration of IoT paradigms and resource ecosystems with a personalized cloud-oriented device-based environment, aiming at an e-Health scenario, highlighting monitoring and early treatment of hospitalized patients, and hospitalizes concentrating on cloud-enabled event detection joined with synchronized reaction. An IOT-based Intensive care unit (ICU) monitoring system is presented to help identify emergency communication and emergency and start communication with medical staff. It is also an active and quick treatment. In this way, help gets started without any delay [29].
2.3.2 Internet of Things (IoT) in Smart Ambulance
Patient monitoring and organization of mobile rescue setups can provide significant value in the sinking time of patients’ recovery and functioning expenditures. Before introducing the innovative intelligence when medical teams were inefficiently interacting, they were effectually aiding in an isolated system unable to connect with the hospital administrative staff [30]. IoT sensing networks assist in devising a solution for managing hazard response within an ambulance setting. This uses the bio sensing networks to observe and analyze the patient’s physical and vital signs. It will strengthen the use of traditional wireless sensor networks to monitor the patient and also increase the performance of the Emergency support system (ESS). Innovative ambulance, when paramedics inefficiently interacted, they were effectually aiding in a closed system unable to connect with the hospital network [31]. This system is interconnected to other equipment networks connected by health devices, sensors, and different parameters required by the intelligent ambulance and reach the patients’ health devices. The actual treatment starts when the patient gets to the hospital, but so much time is wasted, and the patient may lose his life.
To stop this incident, this framework investigates essential well-being boundaries of the patient like pulse, pulse, and internal heat level in the emergency vehicle in ceaseless stretches itself and guides it to the medical clinic’s information base while in transit to the medical clinic. Keeping in see this information, the emergency clinic specialists will understand what kind of treatment should be given to the patient. This can save the pre-activity season for doctors guaranteeing to keep the patient’s life [32].
Ontologies arrange the items or concepts of data to create meaning among them using relations and restrictions among the data terms and ideas so that vocabularies and taxonomies become more meaningful. The use of ontologies provides machine-process able semantics to be shared among several other software and tools. Ontology is a clear explanation of common conceptual interests [33].
Ontological engineering is a combination of therapeutic development works for particular domains. Ontologies are used for defining terms in the field and relationships among them. Explaining concepts in the environment is termed classes. These concepts are arranged in a hierarchy in the form of sub-classes. Object properties and data properties are assigned to these classes [34].
Smart health care systems are hard to define formally because most systems lack standardization of architecture [35]. By doing a literature review of different, IoT-based healthcare systems, we know that there are concepts that have been shared and other systems working in remoteness. We use 101 methodology and already ideas given in OBO1 Foundry has been used. With the help of which, we will be able to define innovative health systems formally and find the commonalities and differences among these systems. By finding the shared concepts between these systems and describing them in ontologies, we can merge them into a cohesive system [36,37].
Some classes and subclasses are defined, and different stakeholders are available in the industry shown in Fig. 1.
Figure 1: Taxonomy of classes and subclasses of a cohesive system
Diverse relations are Shown in Fig. 2 is, representing class service and subclasses. These classes are derived from our literature survey.
Figure 2: Classification of service class
Fig. 3 represents the class of disease and subclasses in relation to subclasses diagnostic services and diagnostic activities and actors involved.
Figure 3: Taxonomy of disease class
Fig. 4 represents the class activities and subclasses, like data acquisition, diagnostic activities, communication activities, patient care, smart prescription & telemedicine, patient medication with IoT enabled pilled box, data processing and analysis.
Figure 4: Taxonomy of activities class
Fig. 5 represents class observation and subclasses like blood observation, heart observation, etc. and their relation with services, data acquisition, data processing and analysis.
Figure 5: Taxonomy of class observation and its subclasses
The linking of two classes by using some predicates represents the semantic relation. An ontology represents the metadata schemas used to integrate different services under one system. These semantic relations form with the help of vocabulary. The following Fig. 6 illustrates the Object property relations between other individuals and different concepts.
Figure 6: Object property hierarchy
In Tab. 1, semantic relations concerning Fig. 6 are discussed in detail with the domain range of each reference concerning object property by mentioning semantic relations.
Following relations have been created in the knowledge model by defining object properties between the classes and explaining the domain range between the types.
4.1 SPARQL Query for Validation and Retrieval
SPARQL is a query language like SQL, which is used with knowledge engineering and knowledge modelling to query data over different RDF triple stores and simultaneously with the ontology itself. It is also used for validation and query ontology using the SPARQL plugin via Protégé or Foskey server using Web interface because the Protégé Web interface did not support SPARQL. The basic theory is to make a query to get a reply from ontology. In Fig. 7, the SPARQL query for arrhythmia detection service is performed.
Figure 7: SPARQL query of arrhythmia detection service
Fig. 8 shows the query in which different properties of the arrhythmia detection system are used. This system belongs to the monitoring system and cardiac disease management system. The arrhythmia detection system has patients of type arrhythmia patient.
Figure 8: SPARQL query of arrhythmia detection
Fig. 9 shows the query in which the arrhythmia detection service possesses different services with corresponding object properties. The arrhythmia detection service is a diagnostic type service. It has the activity of heartbeat segmentation, SVM classification, ECG filtering and DWT.
Figure 9: SPARQL query of arrhythmia detection service
Fig. 10 shows the query in which heart disease monitoring services possess different services with corresponding object properties or data properties. It comes under the monitoring services category. A heart disease monitoring system provides a heart disease monitoring service. Heart disease monitoring service has the activity of heart rate detection, blood pressure detection and data storage on the cloud.
Figure 10: SPARQL query of heart disease monitoring service
Fig. 11 shows the query in which ECG signals have different data or object properties. This observation is processed by ECG filtering activity. DWT and SVM Classifications Activities also process this. Heart Rate Detection Activity acquires ECG signals.
Figure 11: SPARQL query of ECG signals
Fig. 12 shows the query in which the heart rate value has different data or object properties. The heart rate value is of type heart rate. The heart rate value is processed by analyzing parameters against threshold values activity. The heart rate value is acquired by heart rate detection. The heart rate value is also processed by DWT and heartbeat segmentation activities.
Figure 12: SPARQL query of heart rate value
Fig. 13 shows the query in which the heart sound value has different data and object properties. Heart sound value belongs to the type of heart sound. Heart sound value is processed by DWT and heartbeat segmentation activities. Heart sound value is acquired by heart sound sensing activity. Analyses parameters against threshold values activity also process heart sound value.
Figure 13: SPARQL query of heart sound value
4.2 Using Reasoner for Validation
Protégé is only a tool, not an expert system, that helps researchers and ontologists to build ontologies. It uses plugins from different platforms like Pellet, Fact++, other, and Hermit to validate ontologies. Reasoner facilitates by providing proof of the ontology system that has been developed under Protégé by detecting the inconsistency between ontologies. Reasoner is often a mathematical model-based tool as it facilitates logical inferencing using a rule-based approach and semantic web rule language. Pellet Reasoner is a frequently used tool in research for validation. In our study, Pellet Reasoner is also used for verification through SPARQL work with the requirement analysis and has retrieved accordingly.
Diverse, innovative healthcare frameworks are contemplated, giving various smart healthcare services. These services are made out of a different number of activities. These services perform explicit assignments under the particular help. For instance, a demonstrative framework has diverse recognition and observing benefits. In these services, various exercises are underlined for performing the conclusion of the patient. Discovering the shared characteristics and distinctions among the administrations of the different framework is an enormous concerning issue for astute wellbeing.
These frameworks of various medical care applications are arranged in three kinds. These services work in a disengagement way to engage clients in the explicit issue. The harmony of these well-being applications is absent. Every one of these applications deals with its stream and design. This research remembers discovering particular distinctions and shared traits for the independent utilization of IoT health care. Contingent on these shared traits and contrasts, coordinated engineering of various IoT wellbeing applications can be created. This robust design will give clients an assortment of health services applications and a variety under one system.
Acknowledgement: Therefore, the authors gratefully acknowledge DSR’s technical and financial support.
Funding Statement: This work was partially funded by the Deanship of Scientific Research (DSR), King Abdul-Aziz University, Jeddah, Saudi Arabia under Grant No. (D-504-611-1443).
Conflicts of Interest: The authors declare that they have no conflicts of interest to report regarding the present study.
1https://obofoundry.org
References
1. H. Mshali, T. Lemlouma, M. Moloney and D. Magoni, “A survey on health monitoring systems for smart homes,” International Journal of Industrial Ergonomics, vol. 66, no. 1, pp. 26–56, 2018. [Google Scholar]
2. R. R. Pai and S. Alathur, “Bibliometric analysis and methodological review of mobile health services and applications in India,” International Journal of Medical Informatics, vol. 145, no. 1, pp. 104330, 2021. [Google Scholar]
3. K. Hafdi, A. Kriouile and A. Kriouile, “Overview on internet of things architectures, enabling technologies and challenges,” Journal of Computers, vol. 14, no. 9, pp. 557–570, 2019. [Google Scholar]
4. V. G. Bangera and V. Dhiman, “IoT based smart health monitoring for old aged patients,” International Journal of Recent Trends in Engineering and Research, vol. 3, no. 5, pp. 431–437, 2017. [Google Scholar]
5. M. Taştan, “IoT based wearable smart health monitoring system,” Celal Bayar Üniversitesi Fen Bilimleri Dergisi, vol. 14, no. 3, pp. 343–350, 2018. [Google Scholar]
6. A. Gyrard and A. Sheth, “I am happy: Towards an IoT knowledge-based cross-domain well-being recommendation system for everyday happiness,” Smart Health, vol. 15, no. 1, pp. 100083, 2020. [Google Scholar]
7. R. Kumar, R. Mishra, H. P. Gupta and T. Dutta, “Smart sensing for agriculture: Applications, advancements, and challenges,” IEEE Consumer Electronics Magazine, vol. 10, no. 4, pp. 51–56, 2021. [Google Scholar]
8. S. K. Shahzad, D. Ahmed, M. R. Naqvi, M. T. Mushtaq, M. W. Iqbal et al., “Ontology driven smart health service integration,” Computer Methods and Programs in Biomedicine, vol. 207, pp. 106146, 2021. [Google Scholar]
9. V. Vassilakis, I. Moscholios, A. Bontozoglou and M. Logothetis, “Mobility-aware qos assurance in software-defined radio access networks: An analytical study,” in Proc. of Network Softwarization (NetSoft1st IEEE Conf., Tokyo, Japan, pp. 1–6, 2015. [Google Scholar]
10. T. Adbeb, W. Di and M. Ibrar, “Software-defined networking based vanet architecture: Mitigation of traffic congestion,” International Journal of Advanced Computer Science and Applications, vol. 11, no. 3, pp. 25, 2020. [Google Scholar]
11. G. Wu, X. Chen, M. S. Obaidat and C. Lin, “A high efficient node capture attack algorithm in wireless sensor network based on route minimum key set,” Security and Communication Networks, vol. 6, no. 2, pp. 230–238, 2013. [Google Scholar]
12. M. R. Naqvi, M. W. Iqbal, M. U. Ashraf, S. Ahmad, A. T. Soliman et al., “Ontology driven testing strategies for IoT applications,” Computers, Materials & Continua (CMC), vol. 70, no. 3, pp. 5855–5869, 2022. [Google Scholar]
13. M. Sun, E. V. Wijk, S. Koval, R. Van Wijk and M. He, “Measuring ultra-weak photon emission as a non-invasive diagnostic tool for detecting early-stage type 2 diabetes: A step toward personalized medicine,” Journal of Photochemistry and Photobiology B: Biology, vol. 166, no. 1, pp. 86–93, 2017. [Google Scholar]
14. G. M. Bhat and N. G. Bhat, “A novel IoT based framework for blood glucose examination,” in Proc. Int. Conf. on Electrical, Electronics, Communication, Computer and Optimization Techniques (ICEECCOT), Mysuru, India, pp. 205–207, 2017. [Google Scholar]
15. S. Sunny and S. S. Kumar, “Optical based non-invasive glucometer with IoT,” in Proc. Int. Conf. on Power, Signals, Control and Computation (EPSCICON), Thrissur, India, pp. 1–3, 2018. [Google Scholar]
16. A. M. Elmisery, S. Rho and D. Botvich, “A fog based middleware for automated compliance with oecd privacy principles in internet of healthcare things,” IEEE Access, vol. 4, no. 1, pp. 8418–8441, 2016. [Google Scholar]
17. M. Kothari, “Microcontroller based heart beat monitoring and alerting system,” IOSR Journal of Electronics and Communication Engineering (IOSR-JECE), vol. 9, no. 1, pp. 30–32, 2014. [Google Scholar]
18. H. R. Hossein, “SPHMS: Smart patient m-healthcare monitoring system with nfc and IoT,” International Journal of Computer Applications Technology and Research, vol. 4, no. 12, pp. 956–959, 2015. [Google Scholar]
19. D. McGlade and S. S. Hayward, “ML-based cyber incident detection for electronic medical record systems,” Smart Health, vol. 12, no. 1, pp. 3–23, 2019. [Google Scholar]
20. M. W. Iqbal, N. A. Ch, S. K. Shahzad, M. R. Naqvi, B. A. Khan et al., “User context ontology for adaptive mobile-phone interfaces,” IEEE Access, vol. 9, no. 1, pp. 96751–96762, 2021. [Google Scholar]
21. S. Begum and H. Parveen, “U-healthcare and IoT,” International Journal of Computer Science and Mobile Computing, vol. 5, no. 8, pp. 138–142, 2016. [Google Scholar]
22. B. S. Babu, K. Srikanth, T. Ramanjaneyulu and I. L. Narayana, “IoT for healthcare,” International Journal of Science and Research, vol. 5, no. 2, pp. 322–326, 2016. [Google Scholar]
23. L. M. Vaughn and K. Donohoe, “Dangers in the medicine cabinet: Appropriate management of expired and unused prescription drugs,” Home Health Care Management & Practice, vol. 25, no. 4, pp. 155–159, 2013. [Google Scholar]
24. G. Sharma and S. Kalra, “A lightweight user authentication scheme for cloud-IoT based healthcare services,” Iranian Journal of Science and Technology, Transactions of Electrical Engineering, vol. 43, no. 1, pp. 619–636, 2019. [Google Scholar]
25. D. DeMeo and M. Morena, “Medication adherence using a smart pill bottle,” in 11th Int. Conf. & Expo on Emerging Technologies for a Smarter World (CEWIT), New York, USA, pp. 1–4, 2014. [Google Scholar]
26. D. Sethia, D. Gupta and H. Saran, “Smart health record management with secure NFC-enabled mobile devices,” Smart Health, vol. 13, no. 1, pp. 100063, 2019. [Google Scholar]
27. Y. Shen, H. Zhang, Y. Fan, A. P. W. Lee and L. Xu, “Smart health of ultrasound telemedicine based on deeply represented semantic segmentation,” IEEE Internet of Things Journal, vol. 8, no. 23, pp. 16770–16778, 2020. [Google Scholar]
28. S. Distefano, D. Bruneo, F. Longo, G. Merlino and A. Puliafito, “Hospitalized patient monitoring and early treatment using IoT and cloud,” Bio Nano Science, vol. 7, no. 2, pp. 382–385, 2017. [Google Scholar]
29. H. Afreen and I. S. Bajwa, “An IoT-based real-time intelligent monitoring and notification system of cold storage,” IEEE Access, vol. 9, no. 1, pp. 38236–38253, 2021. [Google Scholar]
30. H. Fang, “Mifuzzy clustering for incomplete longitudinal data in smart health,” Smart Health, vol. 1, no. 2, pp. 50–65, 2017. [Google Scholar]
31. H. Samani and R. Zhu, “Robotic automated external defibrillator ambulance for emergency medical service in smart cities,” IEEE Access, vol. 4, no. 1, pp. 268–283, 2016. [Google Scholar]
32. O. Udawant, N. Thombare, D. Chauhan, A. Hadke and D. Waghole, “Smart ambulance system using IoT,” in Proc. 2017 Int. Conf. on Big Data, IoT and Data Science (BID), Pune, India, pp. 171–176, 2017. [Google Scholar]
33. N. Koutroumpouchos, C. Ntantogian, C. Xenakis, “Building trust for smart connected devices: the challenges and pitfalls of trust zone,” Sensors, vol. 21, no. 2, pp. 520, 2021. [Google Scholar]
34. S. Anand and A. Verma, “Development of ontology for smart hospital and implementation using uml and rdf,” International Journal of Computer Science Issues (IJCSI), vol. 7, no. 5, pp. 206, 2010. [Google Scholar]
35. A. Maryam, F. Sallabi, M. Awad, K. Shuaib, M. R. Naqvi et al., “A-SHIP: Ontology-based adaptive sustainable healthcare insurance policy,” Sustainability, vol. 14, no. 3, pp. 1917, 2022. [Google Scholar]
36. T. Naz, M. Akhtar, S. K. Shahzad, M. Fasli, M. W. Iqbal et al., “Ontology-driven advanced drug-drug interaction,” Computers & Electrical Engineering, vol. 86, no. 1, pp. 106695, 2020. [Google Scholar]
37. A. Khamparia and B. Pandey, “Comprehensive analysis of semantic web reasoners and tools: A survey,” Education and Information Technologies, vol. 22, no. 6, pp. 3121–3145, 2017. [Google Scholar]
Cite This Article
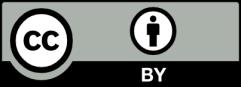