Open Access
ARTICLE
Regulatory Genes Through Robust-SNR for Binary Classification Within Functional Genomics Experiments
1 Department of Statistics, Abdul Wali Khan University, Mardan, 23200, Pakistan
2 Industrial Engineering Department, College of Engineering, King Saud University, P.O. Box 800, Riyadh, 11421, Saudi Arabia
3 Department of Computer Science, COMSATS University Islamabad, Islamabad, 45550, Pakistan
4 Department of Information and Communication Engineering, Yeungnam University, Gyeongsan, 38541, Korea
* Corresponding Author: Muhammad Shafiq. Email:
Computers, Materials & Continua 2023, 74(2), 3663-3677. https://doi.org/10.32604/cmc.2023.030064
Received 17 March 2022; Accepted 10 June 2022; Issue published 31 October 2022
Abstract
The current study proposes a novel technique for feature selection by inculcating robustness in the conventional Signal to noise Ratio (SNR). The proposed method utilizes the robust measures of location i.e., the “Median” as well as the measures of variation i.e., “Median absolute deviation (MAD) and Interquartile range (IQR)” in the SNR. By this way, two independent robust signal-to-noise ratios have been proposed. The proposed method selects the most informative genes/features by combining the minimum subset of genes or features obtained via the greedy search approach with top-ranked genes selected through the robust signal-to-noise ratio (RSNR). The results obtained via the proposed method are compared with well-known gene/feature selection methods on the basis of performance metric i.e., classification error rate. A total of 5 gene expression datasets have been used in this study. Different subsets of informative genes are selected by the proposed and all the other methods included in the study, and their efficacy in terms of classification is investigated by using the classifier models such as support vector machine (SVM), Random forest (RF) and k-nearest neighbors (k-NN). The results of the analysis reveal that the proposed method (RSNR) produces minimum error rates than all the other competing feature selection methods in majority of the cases. For further assessment of the method, a detailed simulation study is also conducted.Keywords
Cite This Article
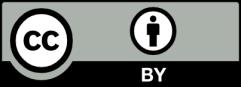