Open Access
ARTICLE
Forecasting Future Trajectories with an Improved Transformer Network
1 School of Instrument Science and Engineering, Southeast University, Nanjing, 210000, China
2 Department of Mechanical Aerospace & Engineering, North Carolina State University, Raleigh, 27695, NC, USA
3 School of Electronic Engineering, Nanjing Xiaozhuang University, Nanjing, 211171, China
* Corresponding Author: Weigong Zhang. Email:
Computers, Materials & Continua 2023, 74(2), 3811-3828. https://doi.org/10.32604/cmc.2023.029787
Received 11 March 2022; Accepted 26 April 2022; Issue published 31 October 2022
Abstract
An increase in car ownership brings convenience to people’s life. However, it also leads to frequent traffic accidents. Precisely forecasting surrounding agents’ future trajectories could effectively decrease vehicle-vehicle and vehicle-pedestrian collisions. Long-short-term memory (LSTM) network is often used for vehicle trajectory prediction, but it has some shortages such as gradient explosion and low efficiency. A trajectory prediction method based on an improved Transformer network is proposed to forecast agents’ future trajectories in a complex traffic environment. It realizes the transformation from sequential step processing of LSTM to parallel processing of Transformer based on attention mechanism. To perform trajectory prediction more efficiently, a probabilistic sparse self-attention mechanism is introduced to reduce attention complexity by reducing the number of queried values in the attention mechanism. Activate or not (ACON) activation function is adopted to select whether to activate or not, hence improving model flexibility. The proposed method is evaluated on the publicly available benchmarks next-generation simulation (NGSIM) and ETH/UCY. The experimental results indicate that the proposed method can accurately and efficiently predict agents’ trajectories.Keywords
Cite This Article
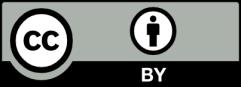
This work is licensed under a Creative Commons Attribution 4.0 International License , which permits unrestricted use, distribution, and reproduction in any medium, provided the original work is properly cited.