Open Access
ARTICLE
Data Mining with Comprehensive Oppositional Based Learning for Rainfall Prediction
1 Department of Information Systems, College of Science & Art at Mahayil, King Khalid University, Abha,62529, Saudi Arabia
2 Department of Information Systems, College of Computer and Information Sciences, Princess Nourah bint Abdulrahman University, Riyadh, 11671, Saudi Arabia
3 Department of Computer Science, College of Computing and Information System, Mecca, 24382, Saudi Arabia
4 Department of Computer and Self Development, Preparatory Year Deanship, Prince Sattam bin Abdulaziz University, AlKharj, 16278, Saudi Arabia
5 Department of Information System, College of Computer Engineering and Sciences, Prince Sattam bin Abdulaziz University, AlKharj, 16278, Saudi Arabia
* Corresponding Author: Manar Ahmed Hamza. Email:
Computers, Materials & Continua 2023, 74(2), 2725-2738. https://doi.org/10.32604/cmc.2023.029163
Received 26 February 2022; Accepted 30 March 2022; Issue published 31 October 2022
Abstract
Data mining process involves a number of steps from data collection to visualization to identify useful data from massive data set. the same time, the recent advances of machine learning (ML) and deep learning (DL) models can be utilized for effectual rainfall prediction. With this motivation, this article develops a novel comprehensive oppositional moth flame optimization with deep learning for rainfall prediction (COMFO-DLRP) Technique. The proposed CMFO-DLRP model mainly intends to predict the rainfall and thereby determine the environmental changes. Primarily, data pre-processing and correlation matrix (CM) based feature selection processes are carried out. In addition, deep belief network (DBN) model is applied for the effective prediction of rainfall data. Moreover, COMFO algorithm was derived by integrating the concepts of comprehensive oppositional based learning (COBL) with traditional MFO algorithm. Finally, the COMFO algorithm is employed for the optimal hyperparameter selection of the DBN model. For demonstrating the improved outcomes of the COMFO-DLRP approach, a sequence of simulations were carried out and the outcomes are assessed under distinct measures. The simulation outcome highlighted the enhanced outcomes of the COMFO-DLRP method on the other techniques.Keywords
Cite This Article
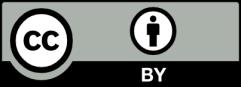
This work is licensed under a Creative Commons Attribution 4.0 International License , which permits unrestricted use, distribution, and reproduction in any medium, provided the original work is properly cited.