Open Access
ARTICLE
Android Malware Detection Using ResNet-50 Stacking
1 Department of Computer Science, Umm Al Qura University, P.O. Box 715, Mecca, Saudi Arabia
2 Curriculums and Teaching Department, Faculty of Education, Umm Al Qura University, P.O. Box 715, Mecca, Saudi Arabia
3 School of Computer Science, University College Dublin, Belfield, Dublin, Ireland
* Corresponding Author: Marwan Albahar. Email:
Computers, Materials & Continua 2023, 74(2), 3997-4014. https://doi.org/10.32604/cmc.2023.028316
Received 06 February 2022; Accepted 05 May 2022; Issue published 31 October 2022
Abstract
There has been an increase in attacks on mobile devices, such as smartphones and tablets, due to their growing popularity. Mobile malware is one of the most dangerous threats, causing both security breaches and financial losses. Mobile malware is likely to continue to evolve and proliferate to carry out a variety of cybercrimes on mobile devices. Mobile malware specifically targets Android operating system as it has grown in popularity. The rapid proliferation of Android malware apps poses a significant security risk to users, making static and manual analysis of malicious files difficult. Therefore, efficient identification and classification of Android malicious files is crucial. Several Convolutional Neural Network (CNN) based methods have been proposed in this regard; however, there is still room for performance improvement. In this work, we propose a transfer learning and stacking approach to efficiently detect the Android malware files by utilizing two well-known machine learning models, ResNet-50 and Support Vector Machine (SVM). The proposed model is trained on the DREBIN dataset by transforming malicious APK files into grayscale images. Our model yields higher performance measures than state-of-the-art works on the DREBIN dataset, where the reported measures are accuracy, recall, precision, and F1 measures of 97.8%, 95.8%, 95.7%, and 95.7%, respectively.Keywords
Cite This Article
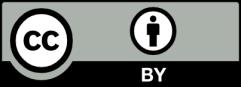