Open Access
ARTICLE
A Multi-Level Circulant Cross-Modal Transformer for Multimodal Speech Emotion Recognition
1 College of Information Engineering, Shanghai Maritime University, Shanghai, 201306, China
2 Shanghai Ship and Shipping Research Institute, Shanghai, 200135, China
3 Division of Management and Education, University of Pittsburgh, Bradford, USA
4 College of Early Childhood Education, Shanghai Normal University, Shanghai, 200234, China
* Corresponding Author: Huihua He. Email:
Computers, Materials & Continua 2023, 74(2), 4203-4220. https://doi.org/10.32604/cmc.2023.028291
Received 07 February 2022; Accepted 14 March 2022; Issue published 31 October 2022
Abstract
Speech emotion recognition, as an important component of human-computer interaction technology, has received increasing attention. Recent studies have treated emotion recognition of speech signals as a multimodal task, due to its inclusion of the semantic features of two different modalities, i.e., audio and text. However, existing methods often fail in effectively represent features and capture correlations. This paper presents a multi-level circulant cross-modal Transformer (MLCCT) for multimodal speech emotion recognition. The proposed model can be divided into three steps, feature extraction, interaction and fusion. Self-supervised embedding models are introduced for feature extraction, which give a more powerful representation of the original data than those using spectrograms or audio features such as Mel-frequency cepstral coefficients (MFCCs) and low-level descriptors (LLDs). In particular, MLCCT contains two types of feature interaction processes, where a bidirectional Long Short-term Memory (Bi-LSTM) with circulant interaction mechanism is proposed for low-level features, while a two-stream residual cross-modal Transformer block is applied when high-level features are involved. Finally, we choose self-attention blocks for fusion and a fully connected layer to make predictions. To evaluate the performance of our proposed model, comprehensive experiments are conducted on three widely used benchmark datasets including IEMOCAP, MELD and CMU-MOSEI. The competitive results verify the effectiveness of our approach.Keywords
Cite This Article
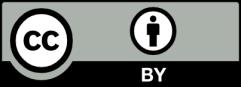
This work is licensed under a Creative Commons Attribution 4.0 International License , which permits unrestricted use, distribution, and reproduction in any medium, provided the original work is properly cited.