Open Access
ARTICLE
Effective Return Rate Prediction of Blockchain Financial Products Using Machine Learning
1 PG and Research Department of Computer Science, Marudupandiyar College (Affiliated to Bharathidasan University, Tiruchirappalli), Thanjavur, 613403, India
2 Department of Electronics and Communication Engineering, Kalasalingam Academy of Research and Education, Krishnankoil, 626126, Tamilnadu, India
3 Department of Computer Science, Faculty of Information Technology, Applied Science Private University, Amman, Jordan
4 Department of Information Technology, Chaitanya Bharathi Institute of Technology, Hyderabad, Telangana, 500075, India
5 Department of Convergence Science, Kongju National University, Gongju, 32588, Korea
6 Department of Computer Science and Engineering, Sejong University, Seoul, 05006, Korea
7 Department of Information and Communication Engineering, Yeungnam University, Gyeongsan-si, Gyeongbuk-do, 38541, Korea
* Corresponding Author: Sung Won Kim. Email:
Computers, Materials & Continua 2023, 74(1), 2303-2316. https://doi.org/10.32604/cmc.2023.033162
Received 09 June 2022; Accepted 12 July 2022; Issue published 22 September 2022
Abstract
In recent times, financial globalization has drastically increased in different ways to improve the quality of services with advanced resources. The successful applications of bitcoin Blockchain (BC) techniques enable the stockholders to worry about the return and risk of financial products. The stockholders focused on the prediction of return rate and risk rate of financial products. Therefore, an automatic return rate bitcoin prediction model becomes essential for BC financial products. The newly designed machine learning (ML) and deep learning (DL) approaches pave the way for return rate predictive method. This study introduces a novel Jellyfish search optimization based extreme learning machine with autoencoder (JSO-ELMAE) for return rate prediction of BC financial products. The presented JSO-ELMAE model designs a new ELMAE model for predicting the return rate of financial products. Besides, the JSO algorithm is exploited to tune the parameters related to the ELMAE model which in turn boosts the classification results. The application of JSO technique assists in optimal parameter adjustment of the ELMAE model to predict the bitcoin return rates. The experimental validation of the JSO-ELMAE model was executed and the outcomes are inspected in many aspects. The experimental values demonstrated the enhanced performance of the JSO-ELMAE model over recent state of art approaches with minimal RMSE of 0.1562.Keywords
Cite This Article
K. Kalyani, V. S. Parvathy, H. A. M. Abdeljaber, T. S. Murthy, S. Acharya et al., "Effective return rate prediction of blockchain financial products using machine learning," Computers, Materials & Continua, vol. 74, no.1, pp. 2303–2316, 2023. https://doi.org/10.32604/cmc.2023.033162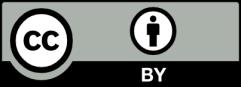