Open Access
ARTICLE
Robust and Reusable Fuzzy Extractors from Non-Uniform Learning with Errors Problem
1 Graduate School of Information Security, Korea University, Seoul, 02841, Korea
2 Department of Computer Science, Sangmyung University, Seoul, 03016, Korea
* Corresponding Author: Jong Hwan Park. Email:
Computers, Materials & Continua 2023, 74(1), 1985-2003. https://doi.org/10.32604/cmc.2023.033102
Received 07 June 2022; Accepted 12 July 2022; Issue published 22 September 2022
Abstract
A fuzzy extractor can extract an almost uniform random string from a noisy source with enough entropy such as biometric data. To reproduce an identical key from repeated readings of biometric data, the fuzzy extractor generates a helper data and a random string from biometric data and uses the helper data to reproduce the random string from the second reading. In 2013, Fuller et al. proposed a computational fuzzy extractor based on the learning with errors problem. Their construction, however, can tolerate a sub-linear fraction of errors and has an inefficient decoding algorithm, which causes the reproducing time to increase significantly. In 2016, Canetti et al. proposed a fuzzy extractor with inputs from low-entropy distributions based on a strong primitive, which is called digital locker. However, their construction necessitates an excessive amount of storage space for the helper data, which is stored in authentication server. Based on these observations, we propose a new efficient computational fuzzy extractor with small size of helper data. Our scheme supports reusability and robustness, which are security notions that must be satisfied in order to use a fuzzy extractor as a secure authentication method in real life. Also, it conceals no information about the biometric data and thanks to the new decoding algorithm can tolerate linear errors. Based on the non-uniform learning with errors problem, we present a formal security proof for the proposed fuzzy extractor. Furthermore, we analyze the performance of our fuzzy extractor scheme and provide parameter sets that meet the security requirements. As a result of our implementation and analysis, we show that our scheme outperforms previous fuzzy extractor schemes in terms of the efficiency of the generation and reproduction algorithms, as well as the size of helper data.Keywords
Cite This Article
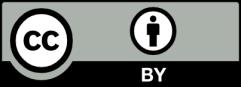