Open Access
ARTICLE
Automated Brain Tumor Diagnosis Using Deep Residual U-Net Segmentation Model
1 Department of Computer Science and Engineering, Periyar Maniammai Institute of Science and Technology, Thanjavur, 613403, India
2 Department of Computer Science, College of Computer Engineering and Sciences, Prince Sattam Bin Abdulaziz University, Alkharj, Saudi Arabia
3 Department of Computer Science and Engineering, Jain Deemed to-be University, Bangalore, 560069, India
4 Department of Neurology, Annapurna Neuro Hospital, Kathmandu, 44600, Nepal
5 Department of Computer Science and Engineering, Sejong University, Seoul, 05006, Korea
6 Department of Information and Communication Engineering, Yeungnam University, Gyeongsan-si, Gyeongbuk-do, 38541, Korea
* Corresponding Author: Sung Won Kim. Email:
Computers, Materials & Continua 2023, 74(1), 2179-2194. https://doi.org/10.32604/cmc.2023.032816
Received 30 May 2022; Accepted 12 July 2022; Issue published 22 September 2022
Abstract
Automated segmentation and classification of biomedical images act as a vital part of the diagnosis of brain tumors (BT). A primary tumor brain analysis suggests a quicker response from treatment that utilizes for improving patient survival rate. The location and classification of BTs from huge medicinal images database, obtained from routine medical tasks with manual processes are a higher cost together in effort and time. An automatic recognition, place, and classifier process was desired and useful. This study introduces an Automated Deep Residual U-Net Segmentation with Classification model (ADRU-SCM) for Brain Tumor Diagnosis. The presented ADRU-SCM model majorly focuses on the segmentation and classification of BT. To accomplish this, the presented ADRU-SCM model involves wiener filtering (WF) based preprocessing to eradicate the noise that exists in it. In addition, the ADRU-SCM model follows deep residual U-Net segmentation model to determine the affected brain regions. Moreover, VGG-19 model is exploited as a feature extractor. Finally, tunicate swarm optimization (TSO) with gated recurrent unit (GRU) model is applied as a classification model and the TSO algorithm effectually tunes the GRU hyperparameters. The performance validation of the ADRU-SCM model was tested utilizing FigShare dataset and the outcomes pointed out the better performance of the ADRU-SCM approach on recent approaches.Keywords
Cite This Article
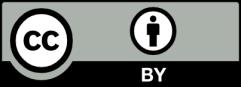
This work is licensed under a Creative Commons Attribution 4.0 International License , which permits unrestricted use, distribution, and reproduction in any medium, provided the original work is properly cited.