Open Access
ARTICLE
GrCol-PPFL: User-Based Group Collaborative Federated Learning Privacy Protection Framework
1 School of Compute Science and Technology, Hainan University, Haikou, 570228, China
2 Hainan Blockchain Technology Engineering Research Center, Hainan University, Haikou, 570228, China
3 Department of Computer Science Texas Tech University, TX, 79409, USA
* Corresponding Author: Zhenhao Liu. Email:
Computers, Materials & Continua 2023, 74(1), 1923-1939. https://doi.org/10.32604/cmc.2023.032758
Received 28 May 2022; Accepted 05 July 2022; Issue published 22 September 2022
Abstract
With the increasing number of smart devices and the development of machine learning technology, the value of users’ personal data is becoming more and more important. Based on the premise of protecting users’ personal privacy data, federated learning (FL) uses data stored on edge devices to realize training tasks by contributing training model parameters without revealing the original data. However, since FL can still leak the user's original data by exchanging gradient information. The existing privacy protection strategy will increase the uplink time due to encryption measures. It is a huge challenge in terms of communication. When there are a large number of devices, the privacy protection cost of the system is higher. Based on these issues, we propose a privacy-preserving scheme of user-based group collaborative federated learning (GrCol-PPFL). Our scheme primarily divides participants into several groups and each group communicates in a chained transmission mechanism. All groups work in parallel at the same time. The server distributes a random parameter with the same dimension as the model parameter for each participant as a mask for the model parameter. We use the public datasets of modified national institute of standards and technology database (MNIST) to test the model accuracy. The experimental results show that GrCol-PPFL not only ensures the accuracy of the model, but also ensures the security of the user’s original data when users collude with each other. Finally, through numerical experiments, we show that by changing the number of groups, we can find the optimal number of groups that reduces the uplink consumption time.Keywords
Cite This Article
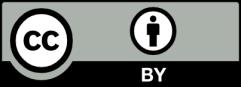
This work is licensed under a Creative Commons Attribution 4.0 International License , which permits unrestricted use, distribution, and reproduction in any medium, provided the original work is properly cited.