Open Access
ARTICLE
A Deep Learning for Alzheimer’s Stages Detection Using Brain Images
1 Department of Information Systems, Faculty of Computing and Information Technology, King Abdulaziz University, Jeddah, Saudi Arabia
2 Department of Computer Sciences, College of Computer and Information Sciences, Princess Nourah bint Abdulrahman University, Riyadh, Saudi Arabia
* Corresponding Author: Zahid Ullah. Email:
Computers, Materials & Continua 2023, 74(1), 1457-1473. https://doi.org/10.32604/cmc.2023.032752
Received 28 May 2022; Accepted 01 July 2022; Issue published 22 September 2022
Abstract
Alzheimer’s disease (AD) is a chronic and common form of dementia that mainly affects elderly individuals. The disease is dangerous because it causes damage to brain cells and tissues before the symptoms appear, and there is no medicinal or surgical treatment available yet for AD. AD causes loss of memory and functionality control in multiple degrees according to AD’s progression level. However, early diagnosis of AD can hinder its progression. Brain imaging tools such as magnetic resonance imaging (MRI), computed tomography (CT) scans, positron emission tomography (PET), etc. can help in medical diagnosis of AD. Recently, computer-aided diagnosis (CAD) such as deep learning applied to brain images obtained with these tools, has been an established strategic methodology that is widely used for clinical assistance in prognosis of AD. In this study, we proposed an intelligent methodology for building a convolutional neural network (CNN) from scratch to detect AD stages from the brain MRI images dataset and to improve patient care. It is worth mentioning that training a deep-learning model requires a large amount of data to produce accurate results and prevent the model from overfitting problems. Therefore, for better understanding of classifiers and to overcome the model overfitting problem, we applied data augmentation to the minority classes in order to increase the number of MRI images in the dataset. All experiments were conducted using Alzheimer’s MRI dataset consisting of brain MRI scanned images. The performance of the proposed model determines detection of the four stages of AD. Experimental results show high performance of the proposed model in that the model achieved a 99.38% accuracy rate, which is the highest so far. Moreover, the proposed model performance in terms of accuracy, precision, sensitivity, specificity, and f-measures is promising when compared to the very recent state-of-the-art domain-specific models existing in the literature.Keywords
Cite This Article
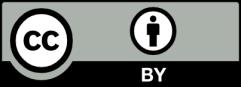