Open Access
ARTICLE
Deep Learning-based Environmental Sound Classification Using Feature Fusion and Data Enhancement
1 Department of Computer Science, COMSATS University Islamabad, Vehari Campus, Pakistan
2 Department of Computer Science, University of Engineering and Technology Lahore, Pakistan
3 Department of Computer Science, College of Computers and Information Technology, Taif University, Taif, 21974, Saudi Arabia
* Corresponding Author: Rashid Jahangir. Email:
Computers, Materials & Continua 2023, 74(1), 1069-1091. https://doi.org/10.32604/cmc.2023.032719
Received 27 May 2022; Accepted 29 June 2022; Issue published 22 September 2022
Abstract
Environmental sound classification (ESC) involves the process of distinguishing an audio stream associated with numerous environmental sounds. Some common aspects such as the framework difference, overlapping of different sound events, and the presence of various sound sources during recording make the ESC task much more complicated and complex. This research is to propose a deep learning model to improve the recognition rate of environmental sounds and reduce the model training time under limited computation resources. In this research, the performance of transformer and convolutional neural networks (CNN) are investigated. Seven audio features, chromagram, Mel-spectrogram, tonnetz, Mel-Frequency Cepstral Coefficients (MFCCs), delta MFCCs, delta-delta MFCCs and spectral contrast, are extracted from the UrbanSound8K, ESC-50, and ESC-10, databases. Moreover, this research also employed three data enhancement methods, namely, white noise, pitch tuning, and time stretch to reduce the risk of overfitting issue due to the limited audio clips. The evaluation of various experiments demonstrates that the best performance was achieved by the proposed transformer model using seven audio features on enhanced database. For UrbanSound8K, ESC-50, and ESC-10, the highest attained accuracies are 0.98, 0.94, and 0.97 respectively. The experimental results reveal that the proposed technique can achieve the best performance for ESC problems.Keywords
Cite This Article
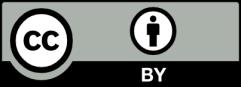
This work is licensed under a Creative Commons Attribution 4.0 International License , which permits unrestricted use, distribution, and reproduction in any medium, provided the original work is properly cited.