Open Access
ARTICLE
Liver Ailment Prediction Using Random Forest Model
1 Department of Electrical Engineering, University of Engineering and Technology, Mardan, 23200, Pakistan
2 City University of Science and Information Technology, Peshawar, Pakistan
3 Department of IT and Computer Science, Pak-Austria Fachhochschule Institute of Applied Sciences and Technology, Haripur, Pakistan
4 Radiological Sciences Department, College of Applied Medical Sciences, Najran University, Najran, Saudi Arabia
5 Department of Information Systems, College of Computer Science and Information Systems, Najran University, Najran, 61441, Saudi Arabia
6 Electrical Engineering Department, College of Engineering, Najran University Saudi Arabia, Najran, 61441, Saudi Arabia
7 Anatomy Department, Medicine College, Najran University, Najran, Saudi Arabia
8 Computer Science Department, College of Computer Science and Information Systems, Najran University, Najran, Saudi Arabia
* Corresponding Author: Fazal Muhammad. Email:
Computers, Materials & Continua 2023, 74(1), 1049-1067. https://doi.org/10.32604/cmc.2023.032698
Received 26 May 2022; Accepted 27 June 2022; Issue published 22 September 2022
Abstract
Today, liver disease, or any deterioration in one’s ability to survive, is extremely common all around the world. Previous research has indicated that liver disease is more frequent in younger people than in older ones. When the liver’s capability begins to deteriorate, life can be shortened to one or two days, and early prediction of such diseases is difficult. Using several machine learning (ML) approaches, researchers analyzed a variety of models for predicting liver disorders in their early stages. As a result, this research looks at using the Random Forest (RF) classifier to diagnose the liver disease early on. The dataset was picked from the University of California, Irvine repository. RF’s accomplishments are contrasted to those of Multi-Layer Perceptron (MLP), Average One Dependency Estimator (A1DE), Support Vector Machine (SVM), Credal Decision Tree (CDT), Composite Hypercube on Iterated Random Projection (CHIRP), K-nearest neighbor (KNN), Naïve Bayes (NB), J48-Decision Tree (J48), and Forest by Penalizing Attributes (Forest-PA). Some of the assessment measures used to evaluate each classifier include Root Relative Squared Error (RRSE), Root Mean Squared Error (RMSE), accuracy, recall, precision, specificity, Matthew’s Correlation Coefficient (MCC), F-measure, and G-measure. RF has an RRSE performance of 87.6766 and an RMSE performance of 0.4328, however, its percentage accuracy is 72.1739. The widely acknowledged result of this work can be used as a starting point for subsequent research. As a result, every claim that a new model, framework, or method enhances forecasting may be benchmarked and demonstrated.Keywords
Cite This Article
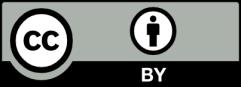
This work is licensed under a Creative Commons Attribution 4.0 International License , which permits unrestricted use, distribution, and reproduction in any medium, provided the original work is properly cited.