Open Access
ARTICLE
Jellyfish Search Optimization with Deep Learning Driven Autism Spectrum Disorder Classification
1 Department of Computer Science & Engineering, Aditya Engineering College, Surampalem, Andhra Pradesh, India
2 Department of Computer Science & Engineering, Ajay Kumar Garg Engineering College, Ghaziabad, Uttar Pradesh, India
3 Department of Human Resource Management, Moscow Aviation Institute, Moscow, Russia
4 Department of Computer Science and Engineering, Vignan’s Institute of Information Technology, Visakhapatnam, 530049, India
5 Computer Communication Engineering, Al-Rafidain University College, Baghdad, Iraq
6 Department of Medical Instrumentation Techniques Engineering, Al-Mustaqbal University College, Hillah, 51001, Iraq
7 Department of Computer Technical Engineering, Al-Hadba University College, Mosul, Iraq
8 College of Technical Engineering, The Islamic University, Najaf, Iraq
* Corresponding Author: Ahmed Alkhayyat. Email:
Computers, Materials & Continua 2023, 74(1), 2195-2209. https://doi.org/10.32604/cmc.2023.032586
Received 23 May 2022; Accepted 24 June 2022; Issue published 22 September 2022
Abstract
Autism spectrum disorder (ASD) is regarded as a neurological disorder well-defined by a specific set of problems associated with social skills, recurrent conduct, and communication. Identifying ASD as soon as possible is favourable due to prior identification of ASD permits prompt interferences in children with ASD. Recognition of ASD related to objective pathogenic mutation screening is the initial step against prior intervention and efficient treatment of children who were affected. Nowadays, healthcare and machine learning (ML) industries are combined for determining the existence of various diseases. This article devises a Jellyfish Search Optimization with Deep Learning Driven ASD Detection and Classification (JSODL-ASDDC) model. The goal of the JSODL-ASDDC algorithm is to identify the different stages of ASD with the help of biomedical data. The proposed JSODL-ASDDC model initially performs min-max data normalization approach to scale the data into uniform range. In addition, the JSODL-ASDDC model involves JSO based feature selection (JFSO-FS) process to choose optimal feature subsets. Moreover, Gated Recurrent Unit (GRU) based classification model is utilized for the recognition and classification of ASD. Furthermore, the Bacterial Foraging Optimization (BFO) assisted parameter tuning process gets executed to enhance the efficacy of the GRU system. The experimental assessment of the JSODL-ASDDC model is investigated against distinct datasets. The experimental outcomes highlighted the enhanced performances of the JSODL-ASDDC algorithm over recent approaches.Keywords
Cite This Article
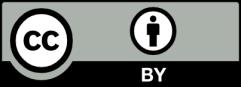