Open Access
ARTICLE
Epileptic Seizures Diagnosis Using Amalgamated Extremely Focused EEG Signals and Brain MRI
Department of Computer Science, King Saud University, Riyadh, 11543, Saudi Arabia
* Corresponding Author: Farah Mohammad. Email:
Computers, Materials & Continua 2023, 74(1), 623-639. https://doi.org/10.32604/cmc.2023.032552
Received 22 May 2022; Accepted 22 June 2022; Issue published 22 September 2022
Abstract
There exists various neurological disorder based diseases like tumor, sleep disorder, headache, dementia and Epilepsy. Among these, epilepsy is the most common neurological illness in humans, comparable to stroke. Epilepsy is a severe chronic neurological illness that can be discovered through analysis of the signals generated by brain neurons and brain Magnetic resonance imaging (MRI). Neurons are intricately coupled in order to communicate and generate signals from human organs. Due to the complex nature of electroencephalogram (EEG) signals and MRI’s the epileptic seizures detection and brain related problems diagnosis becomes a challenging task. Computer based techniques and machine learning models are continuously giving their contributions to diagnose all such diseases in a better way than the normal process of diagnosis. Their performance may sometime degrade due to missing information, selection of poor classification model and unavailability of quality data that are used to train the models for better prediction. This research work is an attempt to epileptic seizures detection by using a multi focus dataset based on EEG signals and brain MRI. The key steps of this work are: feature extraction having two different streams i.e., EEG using wavelet transformation along with SVD-Entropy, and MRI using convolutional neural network (CNN), after extracting features from both streams, feature fusion is applied to generate feature vector used by support vector machine (SVM) to diagnose the epileptic seizures. From the experimental evaluation and results comparison with the current state-of-the-art techniques, it has been concluded that the performance of the proposed scheme is better than the existing models.
Keywords
Cite This Article
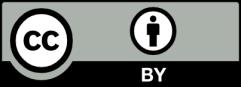