Open Access
ARTICLE
Deep Attention Network for Pneumonia Detection Using Chest X-Ray Images
1 Department of Information Technology, JSS Academy of Technical Education, Noida, India
2 JSS Academy of Technical Education, Noida, India
3 Department of Electronics and Communication Engineering, JECRC University Jaipur, Rajasthan, India
4 Harcourt Butler Technological University Kanpur, India
5 Department of Electrical Engineering, Prince Sattam Bin Abdulaziz University, College of Engineering, Al Kharj, 16278, Saudi Arabia
6 Faculty of Engineering, Imperial College London, London, SW7 2AZ, UK
7 College of Arts, Media and Technology, Chiang Mai University, Chiang Mai, 50200, Thailand
* Corresponding Author: Orawit Thinnukool. Email:
Computers, Materials & Continua 2023, 74(1), 1673-1691. https://doi.org/10.32604/cmc.2023.032364
Received 15 May 2022; Accepted 22 June 2022; Issue published 22 September 2022
Abstract
In computer vision, object recognition and image categorization have proven to be difficult challenges. They have, nevertheless, generated responses to a wide range of difficult issues from a variety of fields. Convolution Neural Networks (CNNs) have recently been identified as the most widely proposed deep learning (DL) algorithms in the literature. CNNs have unquestionably delivered cutting-edge achievements, particularly in the areas of image classification, speech recognition, and video processing. However, it has been noticed that the CNN-training assignment demands a large amount of data, which is in low supply, especially in the medical industry, and as a result, the training process takes longer. In this paper, we describe an attention-aware CNN architecture for classifying chest X-ray images to diagnose Pneumonia in order to address the aforementioned difficulties. Attention Modules provide attention-aware properties to the Attention Network. The attention-aware features of various modules alter as the layers become deeper. Using a bottom-up top-down feedforward structure, the feedforward and feedback attention processes are integrated into a single feedforward process inside each attention module. In the present work, a deep neural network (DNN) is combined with an attention mechanism to test the prediction of Pneumonia disease using chest X-ray pictures. To produce attention-aware features, the suggested network was built by merging channel and spatial attention modules in DNN architecture. With this network, we worked on a publicly available Kaggle chest X-ray dataset. Extensive testing was carried out to validate the suggested model. In the experimental results, we attained an accuracy of 95.47% and an F- score of 0.92, indicating that the suggested model outperformed against the baseline models.Keywords
Cite This Article
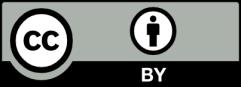
This work is licensed under a Creative Commons Attribution 4.0 International License , which permits unrestricted use, distribution, and reproduction in any medium, provided the original work is properly cited.