Open Access
ARTICLE
A Novel Siamese Network for Few/Zero-Shot Handwritten Character Recognition Tasks
Department of Information System, Faculty of Computers and Information, Mansoura University, Mansoura, P.O.35516, Egypt
* Corresponding Author: Nagwa Elaraby. Email:
Computers, Materials & Continua 2023, 74(1), 1837-1854. https://doi.org/10.32604/cmc.2023.032288
Received 13 May 2022; Accepted 12 July 2022; Issue published 22 September 2022
Abstract
Deep metric learning is one of the recommended methods for the challenge of supporting few/zero-shot learning by deep networks. It depends on building a Siamese architecture of two homogeneous Convolutional Neural Networks (CNNs) for learning a distance function that can map input data from the input space to the feature space. Instead of determining the class of each sample, the Siamese architecture deals with the existence of a few training samples by deciding if the samples share the same class identity or not. The traditional structure for the Siamese architecture was built by forming two CNNs from scratch with randomly initialized weights and trained by binary cross-entropy loss. Building two CNNs from scratch is a trial and error and time-consuming phase. In addition, training with binary cross-entropy loss sometimes leads to poor margins. In this paper, a novel Siamese network is proposed and applied to few/zero-shot Handwritten Character Recognition (HCR) tasks. The novelties of the proposed network are in. 1) Utilizing transfer learning and using the pre-trained AlexNet as a feature extractor in the Siamese architecture. Fine-tuning a pre-trained network is typically faster and easier than building from scratch. 2) Training the Siamese architecture with contrastive loss instead of the binary cross-entropy. Contrastive loss helps the network to learn a nonlinear mapping function that enables it to map the extracted features in the vector space with an optimal way. The proposed network is evaluated on the challenging Chars74K datasets by conducting two experiments. One is for testing the proposed network in few-shot learning while the other is for testing it in zero-shot learning. The recognition accuracy of the proposed network reaches to 85.6% and 82% in few- and zero-shot learning respectively. In addition, a comparison between the performance of the proposed Siamese network and the traditional Siamese CNNs is conducted. The comparison results show that the proposed network achieves higher recognition results in less time. The proposed network reduces the training time from days to hours in both experiments.Keywords
Cite This Article
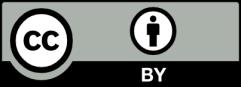
This work is licensed under a Creative Commons Attribution 4.0 International License , which permits unrestricted use, distribution, and reproduction in any medium, provided the original work is properly cited.