Open Access
ARTICLE
Sigmoidal Particle Swarm Optimization for Twitter Sentiment Analysis
1 Department of Computer Science and Engineering, CHRIST (Deemed to be University), Bangalore, 560074, India
2 Information Systems Department, College of Computer and Information Sciences, Imam Mohammad Ibn Saud Islamic University (IMSIU), Riyadh, 11432, Saudi Arabia
* Corresponding Author: Abdul Khader Jilani Saudagar. Email:
Computers, Materials & Continua 2023, 74(1), 897-914. https://doi.org/10.32604/cmc.2023.031867
Received 28 April 2022; Accepted 06 June 2022; Issue published 22 September 2022
Abstract
Social media, like Twitter, is a data repository, and people exchange views on global issues like the COVID-19 pandemic. Social media has been shown to influence the low acceptance of vaccines. This work aims to identify public sentiments concerning the COVID-19 vaccines and better understand the individual’s sensitivities and feelings that lead to achievement. This work proposes a method to analyze the opinion of an individual’s tweet about the COVID-19 vaccines. This paper introduces a sigmoidal particle swarm optimization (SPSO) algorithm. First, the performance of SPSO is measured on a set of 12 benchmark problems, and later it is deployed for selecting optimal text features and categorizing sentiment. The proposed method uses TextBlob and VADER for sentiment analysis, CountVectorizer, and term frequency-inverse document frequency (TF-IDF) vectorizer for feature extraction, followed by SPSO-based feature selection. The Covid-19 vaccination tweets dataset was created and used for training, validating, and testing. The proposed approach outperformed considered algorithms in terms of accuracy. Additionally, we augmented the newly created dataset to make it balanced to increase performance. A classical support vector machine (SVM) gives better accuracy for the augmented dataset without a feature selection algorithm. It shows that augmentation improves the overall accuracy of tweet analysis. After the augmentation performance of PSO and SPSO is improved by almost 7% and 5%, respectively, it is observed that simple SVM with 10-fold cross-validation significantly improved compared to the primary dataset.Keywords
Cite This Article
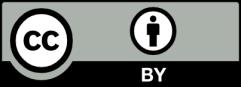
This work is licensed under a Creative Commons Attribution 4.0 International License , which permits unrestricted use, distribution, and reproduction in any medium, provided the original work is properly cited.