Open Access
ARTICLE
Optimization Scheme of Trusted Task Offloading in IIoT Scenario Based on DQN
1 Beijing University of Posts and Telecommunications, Beijing, 100876, China
2 China United Network Communications Corporation Beijing Branch, 100800, China
3 Beijing Information Science and Technology University, Beijing, 100192, China
4 University of Quebec at Montreal, Montreal, H2X 3X2, Canada
* Corresponding Author: Zikui Lu. Email:
Computers, Materials & Continua 2023, 74(1), 2055-2071. https://doi.org/10.32604/cmc.2023.031750
Received 26 April 2022; Accepted 29 June 2022; Issue published 22 September 2022
Abstract
With the development of the Industrial Internet of Things (IIoT), end devices (EDs) are equipped with more functions to capture information. Therefore, a large amount of data is generated at the edge of the network and needs to be processed. However, no matter whether these computing tasks are offloaded to traditional central clusters or mobile edge computing (MEC) devices, the data is short of security and may be changed during transmission. In view of this challenge, this paper proposes a trusted task offloading optimization scheme that can offer low latency and high bandwidth services for IIoT with data security. Blockchain technology is adopted to ensure data consistency. Meanwhile, to reduce the impact of low throughput of blockchain on task offloading performance, we design the processes of consensus and offloading as a Markov decision process (MDP) by defining states, actions, and rewards. Deep reinforcement learning (DRL) algorithm is introduced to dynamically select offloading actions. To accelerate the optimization, we design a novel reward function for the DRL algorithm according to the scale and computational complexity of the task. Experiments demonstrate that compared with methods without optimization, our mechanism performs better when it comes to the number of task offloading and throughput of blockchain.Keywords
Cite This Article
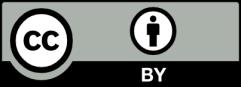
This work is licensed under a Creative Commons Attribution 4.0 International License , which permits unrestricted use, distribution, and reproduction in any medium, provided the original work is properly cited.