Open Access
ARTICLE
A U-Net-Based CNN Model for Detection and Segmentation of Brain Tumor
1 Department of Computer Science, COMSATS University Islamabad, Sahiwal Campus, Sahiwal, 57000, Pakistan
2 Radiological Sciences Department, College of applied medical sciences, Najran University, Najran, 61441, Saudi Arabia
3 Department of Information Systems, College of Computer Science and Information Systems, Najran University, Najran, 61441, Saudi Arabia
4 Anatomy Department, Medicine College, Najran University, Najran, 61441, Saudi Arabia
* Corresponding Author: Hassan A. Alshamrani. Email:
Computers, Materials & Continua 2023, 74(1), 1333-1349. https://doi.org/10.32604/cmc.2023.031695
Received 25 April 2022; Accepted 17 June 2022; Issue published 22 September 2022
Abstract
Human brain consists of millions of cells to control the overall structure of the human body. When these cells start behaving abnormally, then brain tumors occurred. Precise and initial stage brain tumor detection has always been an issue in the field of medicines for medical experts. To handle this issue, various deep learning techniques for brain tumor detection and segmentation techniques have been developed, which worked on different datasets to obtain fruitful results, but the problem still exists for the initial stage of detection of brain tumors to save human lives. For this purpose, we proposed a novel U-Net-based Convolutional Neural Network (CNN) technique to detect and segmentizes the brain tumor for Magnetic Resonance Imaging (MRI). Moreover, a 2-dimensional publicly available Multimodal Brain Tumor Image Segmentation (BRATS2020) dataset with 1840 MRI images of brain tumors has been used having an image size of 240 × 240 pixels. After initial dataset preprocessing the proposed model is trained by dividing the dataset into three parts i.e., testing, training, and validation process. Our model attained an accuracy value of 0.98 % on the BRATS2020 dataset, which is the highest one as compared to the already existing techniques.Keywords
Cite This Article
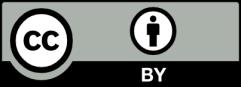
This work is licensed under a Creative Commons Attribution 4.0 International License , which permits unrestricted use, distribution, and reproduction in any medium, provided the original work is properly cited.