Open Access
ARTICLE
Data-Driven Models for Predicting Solar Radiation in Semi-Arid Regions
1 Engineering Faculty, Shohadaye Hoveizeh Campus of Technology, Shahid Chamran University of Ahvaz, Dashte Azadegan, Iran
2 Energies and Materials Research Laboratory, Department of Matter Sciences, Faculty of Sciences and Technology, University of Tamanghasset, Tamanghasset, Algeria
3 Unité de Recherche en Energies Renouvelables en Milieu Saharien (URERMS),Centre de Développement des Energies Renouvelables (CDER), 01000, Adrar, Algeria
4 Mechanical Power Engineering Department, Faculty of Engineering, Cairo University, Giza, 12613, Giza, Egypt
5 Agricultural Engineering Department, Faculty of Agriculture, Mansoura University, Mansoura, 35516, Egypt
6 CERIS, Instituto Superior Técnico,Universidade de Lisboa, Lisbon, Portugal
7 Department of Civil, Environmental and Natural Resources Engineering, Lulea University of Technology, 97187, Lulea, Sweden
8 Universidad Politécnica de Madrid,UPM, Avd., Puerta de Hierro, 28040, Madrid, Spain
9 Department of Communications and Electronics, Delta Higher Institute of Engineering and Technology, Mansoura, 35111, Egypt
10 Faculty of Artificial Intelligence, Delta University for Science and Technology, Mansoura, 35712, Egypt
* Corresponding Author: Nadjem Bailek. Email:
Computers, Materials & Continua 2023, 74(1), 1625-1640. https://doi.org/10.32604/cmc.2023.031406
Received 17 April 2022; Accepted 12 June 2022; Issue published 22 September 2022
Abstract
Solar energy represents one of the most important renewable energy sources contributing to the energy transition process. Considering that the observation of daily global solar radiation (GSR) is not affordable in some parts of the globe, there is an imperative need to develop alternative ways to predict it. Therefore, the main objective of this study is to evaluate the performance of different hybrid data-driven techniques in predicting daily GSR in semi-arid regions, such as the majority of Spanish territory. Here, four ensemble-based hybrid models were developed by hybridizing Additive Regression (AR) with Random Forest (RF), Locally Weighted Linear Regression (LWLR), Random Subspace (RS), and M5P. The base algorithms of the developed models are scarcely applied in previous studies to predict solar radiation. The testing phase outcomes demonstrated that the AR-RF models outperform all other hybrid models. The provided models were validated by statistical metrics, such as the correlation coefficient (R) and root mean square error (RMSE). The results proved that Scenario #6, utilizing extraterrestrial solar radiation, relative humidity, wind speed, and mean, maximum, and minimum ambient air temperatures as the model inputs, leads to the most accurate predictions among all scenarios (R = 0.968–0.988 and RMSE = 1.274–1.403 MJ/m2⋅d). Also, Scenario #3 stood in the next rank of accuracy for predicting the solar radiation in both validating stations. The AD-RF model was the best predictive, followed by AD-RS and AD-LWLR. Hence, this study recommends new effective methods to predict GSR in semi-arid regions.Keywords
Cite This Article
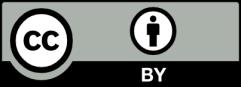