Open Access
ARTICLE
Deep Learned Singular Residual Network for Super Resolution Reconstruction
1 Department of Electronics and Communications, V R Siddhartha Engineering College, Vijayawada, 520007, India
2 Department of Electronics and Communication Engineering, Koneru Lakshmaiah Education Foundation, Vaddeswaram, Guntur, 522502, India
3 Department of CSE, Anil Neerukonda Institute of Technology & Sciences, Sangivalasa, Visakhapatnam, 531162, India
* Corresponding Author: Gunnam Suryanarayana. Email:
Computers, Materials & Continua 2023, 74(1), 1123-1137. https://doi.org/10.32604/cmc.2023.031227
Received 12 April 2022; Accepted 10 June 2022; Issue published 22 September 2022
Abstract
Single image super resolution (SISR) techniques produce images of high resolution (HR) as output from input images of low resolution (LR). Motivated by the effectiveness of deep learning methods, we provide a framework based on deep learning to achieve super resolution (SR) by utilizing deep singular-residual neural network (DSRNN) in training phase. Residuals are obtained from the difference between HR and LR images to generate LR-residual example pairs. Singular value decomposition (SVD) is applied to each LR-residual image pair to decompose into subbands of low and high frequency components. Later, DSRNN is trained on these subbands through input and output channels by optimizing the weights and biases of the network. With fewer layers in DSRNN, the influence of exploding gradients is reduced. This speeds up the learning process and also improves accuracy by using skip connections. The trained DSRNN parameters yield residuals to recover the HR subbands in the testing phase. Experimental analysis shows that the proposed method results in superior performance to existing methods in terms of subjective quality. Extensive testing results on popular benchmark datasets such as set5, set14, and urban100 for a scaling factor of 4 show the effectiveness of the proposed method across different qualitative evaluation metrics.Keywords
Cite This Article
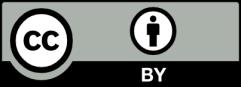
This work is licensed under a Creative Commons Attribution 4.0 International License , which permits unrestricted use, distribution, and reproduction in any medium, provided the original work is properly cited.