Open Access
ARTICLE
Smart Home IoT Privacy and Security Preservation via Machine Learning Techniques
College of Computer Science and Engineering, University of Hafr Albatin, Saudi Arabia
* Corresponding Author: Mubarak Almutairi. Email:
Computers, Materials & Continua 2023, 74(1), 1959-1983. https://doi.org/10.32604/cmc.2023.031155
Received 11 April 2022; Accepted 10 June 2022; Issue published 22 September 2022
Abstract
The development and use of Internet of Things (IoT) devices have grown significantly in recent years. Advanced IoT device characteristics are mainly to blame for the wide range of applications that may now be achieved with IoT devices. Corporations have begun to embrace the IoT concept. Identifying true and suitable devices, security faults that might be used for bad reasons, and administration of such devices are only a few of the issues that IoT, a new concept in technological progress, provides. In some ways, IoT device traffic differs from regular device traffic. Devices with particular features can be classified into categories, irrespective of their function or performance. Ever-changing and complex environments, like a smart home, demand this classification scheme. A total of 41 IoT devices were employed in this investigation. To build a multiclass classification model, IoT devices contributed 13 network traffic parameters. To further preprocess the raw data received, preprocessing techniques like Normalization and Dataset Scaling were utilized. Feature engineering techniques can extract features from the text data. A total of 117,423 feature vectors are contained in the dataset after stratification, which are used to further improve the classification model. In this study, a variety of performance indicators were employed to show the performance of the logiboosted algorithms. Logi-XGB scored 80.2% accuracy following application of the logit-boosted algorithms to the dataset for classification, whereas Logi-GBC achieved 77.8% accuracy. Meanwhile, Logi-ABC attained 80.7% accuracy. Logi-CBC, on the other hand, received the highest Accuracy score of 85.6%. The accuracy of Logi-LGBM and Logi-HGBC was the same at 81.37% each. Our suggested Logi-CBC showed the highest accuracy on the dataset when compared to existing Logit-Boosted Algorithms used in earlier studies.Keywords
Cite This Article
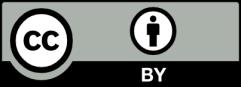