Open Access
ARTICLE
Augmenting IoT Intrusion Detection System Performance Using Deep Neural Network
1 Department of Computer Science, Islamia College Peshawar, Peshawar, 25120, Pakistan
2 Department of Computer Science, CECOS University of IT and Emerging Sciences, Peshawar, 25000, Pakistan
3 Department of Electrical Engineering, HITEC University Taxila, Taxila, 47080, Pakistan
4 Computer Science Department, College of Computer and Information Sciences, Imam Mohammad Ibn Saud Islamic University (IMSIU), Riyadh, 11432, Saudi Arabia
5 DAAI Research Group, Department of Computing and Data Science, School of Computing and Digital Technology, Birmingham City University, Birmingham, B4 7XG, UK
* Corresponding Author: Muhammad Shoaib. Email:
Computers, Materials & Continua 2023, 74(1), 1351-1374. https://doi.org/10.32604/cmc.2023.030831
Received 02 April 2022; Accepted 15 June 2022; Issue published 22 September 2022
Abstract
Due to their low power consumption and limited computing power, Internet of Things (IoT) devices are difficult to secure. Moreover, the rapid growth of IoT devices in homes increases the risk of cyber-attacks. Intrusion detection systems (IDS) are commonly employed to prevent cyberattacks. These systems detect incoming attacks and instantly notify users to allow for the implementation of appropriate countermeasures. Attempts have been made in the past to detect new attacks using machine learning and deep learning techniques, however, these efforts have been unsuccessful. In this paper, we propose two deep learning models to automatically detect various types of intrusion attacks in IoT networks. Specifically, we experimentally evaluate the use of two Convolutional Neural Networks (CNN) to detect nine distinct types of attacks listed in the NF-UNSW-NB15-v2 dataset. To accomplish this goal, the network stream data were initially converted to two-dimensional images, which were then used to train the neural network models. We also propose two baseline models to demonstrate the performance of the proposed models. Generally, both models achieve high accuracy in detecting the majority of these nine attacks.Keywords
Cite This Article
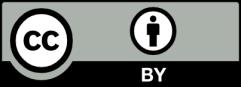
This work is licensed under a Creative Commons Attribution 4.0 International License , which permits unrestricted use, distribution, and reproduction in any medium, provided the original work is properly cited.