Open Access
ARTICLE
An Integrated and Comprehensive Fuzzy Multi-Criteria Model for Electronic Wallet Selection
Faculty of Business Administration, Posts and Telecommunications Institute of Technology (PTIT), Ho Chi Minh City, 700000, Vietnam
* Corresponding Author: Phuoc Van Nguyen. Email:
Computers, Materials & Continua 2023, 74(1), 2279-2301. https://doi.org/10.32604/cmc.2023.030019
Received 16 March 2022; Accepted 25 May 2022; Issue published 22 September 2022
Abstract
Electronic wallet (E-wallet), which combines software, hardware, and human interaction, is a modern platform for electronic payment. Financial services managers can create more effective strategies for advancing existing positions by evaluating the success of e-wallet services. The objective of this research is to create an integrated model for assessing E-wallet services using the Fuzzy Analytic Hierarchy Process (FAHP) in conjunction with a technique for extent analysis and the ARAS approach (Additive Ratio Assessment). In this study, E-wallet service providers are ranked and evaluated using the ARAS approach and the FAHP priority weights. In addition, a case study from Vietnam illustrates how the methodology is used. The data shows that perceived usefulness, perceived usability, perceived simplicity of use, and perceived threat have the least impact on how acceptable an E-wallet is. As a result, the suggested model improves the efficiency of E-wallet service operations and assists in overcoming the challenges associated with the evaluation of E-wallet services. The case finding demonstrates that the suggested model is a reliable and useful tool for making decisions when assessing E-wallet service providers functioning in a complex and changing environment.Keywords
Organizations achieve their business objectives more quickly when innovative technological facilities and procedures are used. Technology applications play a critical role in facilitating the processes of knowledge production, storage, sharing, and application in a globalizing and competitive market. Throughout these procedures, it is available to take advantage of the opportunities offered by the changing environments. Nonetheless, even just a technological infrastructure can ensure the effectiveness of a performance measurement system. The solution is derived from the strategic alignment of human and knowledge resources, technologies, culture of the organization, and procedures [1].
Electronic wallet (E-wallet) provides a function to make payments electronically, allowing users to exchange cash across transaction accounts, either traditional banking accounts or electronic money deposit accounts, as well as use extra payment mechanisms. When an E-wallet is built on mobile devices (such as smartphones or tablets), it is known as a mobile wallet [2,3]. As a result, mobile wallets simulate a physical wallet on a mobile device, where users can store various payment instruments (e.g., cards, transfers) to conduct mobile payments.
Mobile payments can support and stimulate the deployment of payment services, including person-to-person transfers, government-to-person transfers, online and offline purchases of goods and services (i.e., person-to-business transfer of funds), and the payment of bills and fees [4,5]. Consequently, digital payments and e-wallets are viewed as helpful to (unbanked) upper-middle-class and lower-class populations, as well as a tool during backup and recovery and emergency response personnel [4,6].
Additionally, the factors influencing the use of mobile payments and E-wallets have been researched. The authors of [7] present a comprehensive investigation of the key factors that drive consumers to use mobile payments. There are 10 primary technology, human, and environment variables identified: ease of use, perceived usefulness, perceived trust, perceived risk, social influence, perceived security, effort expectancy, attitude, performance expectancy, and facilitating condition. Furthermore, Kaur et al. [2] state that E-wallets are intended to provide speed, convenience of use, efficiency, efficacy, transparency, and accessibility. The authors of [3] state that the convenience of use provided by E-wallets should be the primary justification for using this system daily. According to the authors of [4], the popularity of mobile payments and E-wallets in developing countries is due to a lack of alternatives to cash, access to banking products, poorly developed infrastructure, and high costs for money transfer services.
Even though E-wallets have received much interest in developing markets, their adoption is still limited and uneven [2,3]. Variations in E-wallet use can be attributed to differences in internet access, literacy, access to financial services, and infrastructure [3]. According to the author [4] where mobile wallet systems were being established, they have primarily been used for person-to-person transactions, but they have also been used to acquire goods or services, as well as to make payments and levies. According to Iman [4] mobile wallets have enabled government-to-person transactions and have made a significant impact in backup and recovery and emergency services. Since a Covid-19 epidemic was proved [8], various governments, like Vietnam’s, effectively used E-wallet or other paytech systems to transfer funds in the form of subsidies to the bottom-of-the-pyramid unbanked population.
Vietnam, as a developing market, has seen some significant advancements in the context of digital payments throughout the last decade, which has become the fintech industry that has received the most attention and money from shareholders in Vietnam in recent years. As one of Southeast Asia’s greatest cash-reliant countries, Vietnam now has one of the top noncash payment acceptance highest in the region in 2020. The growing e-wallet industry has contributed significantly to the growth of Vietnamese mobile transactions, accounting for an increasing transaction value that is expected to exceed 48 billion U.S. dollars by 2025.
With the growth of the Vietnamese e-commerce business comes an increase in mobile payment methods. The COVID-19 pandemic has adopted electronic payment systems, particularly e-wallets, and the “wave of the future” by many of Vietnam’s increasingly connected consumers. According to the authors of [9], there were approximately 19.2 million mobile wallet users in the country in 2020, with the number of users expected to nearly triple between 2020 and 2025. As shown in a survey conducted in 2021, e-wallets were used by about 60% of respondents across Vietnam. This rate was significantly higher in larger cities such Vietnam Ha Noi and Ho Chi Minh City. According to the same survey, more than 60% of Vietnamese customers used at least two e-wallet apps.
In Vietnam’s booming financial sector, e-wallets have played a central role. Vietnam is a promising market for this digital service due to its young, connected population and rising economy. As a result, competition among an increasing number of e-wallet providers has accelerated the industry’s growth in just a few years. Polasik et al. [10] began with five e-wallet providers in 2015, and by 2020, Vietnamese consumers have over 30 E-wallets from which to choose.
This segment highlights some of the most successful digital enterprises in Vietnam and Southeast Asia, such as ViettelPay, which is owned and operated by the local Viettel Group, and ShopeePay, which is owned and operated by the Singaporean Sea Group (AirPay). On the other side, the Vietnamese mega app MoMo has maintained its position as the industry’s most dominant mobile wallet brand, holding more than 50% of the market share as of the year 2020. MoMo is currently concentrating on its expansion after purportedly raking in a total of 100 million U.S. dollars from its investment. As of the year 2021, the company has already amassed more than 25 million users as members. Moreover, several e-wallets have contributed a lot in attracting users owing to the increase in the role of electronic payment during the COVID-19 epidemic. This indicates that the dynamic competition is ongoing and that the Vietnamese e-payment sector is continuing to grow.
In terms of the reality that E-wallets are becoming an increasingly popular means of carrying out financial transactions, analyzing their performance is vital for financial services provider, entrepreneurs, customers, shareholders, policymakers, and those delivering services are all included. The owners of banks, those who determine the policy, and those who deliver services can all benefit from analyzing how well mobile banking services function. Because of this, the subject of performance evaluation might be approached as a multiple-criteria decision making (MCDM) problem alternative. The principal advantages of MCDM include the ability to combine quantitative and qualitative aspects in the evaluation process, the analysis of complex circumstances, and the active participation of decision makers in the decision making process [11]. In actuality, since human perception, judgment, intuition, and preferences remain unknown and difficult to measure, a concrete proof may not always be appropriate to introduce the decision-making process. The author in [12], Fuzzy Set Theory (FST), is a technique of analyzing ambiguous notions and mediates for describing vagueness [13,14]. It is imperceptible to the human reasoning process in that it uses insufficient understanding and complexity to make conclusions. It became specifically developed for the mathematical representation of ambiguity and uncertainty, and it provides a valuable tool for dealing with the lack of precision that is intrinsic to many problems. Many selection issues can be considerably simplified since information can be conveyed more easily using fuzzy sets. FST is applied to data classes whose boundaries are not precisely stated. As an outcome, the actual problems can be solved more simply. As a conclusion, linguistic variables play an important part in fuzzy logic applications [15]. In this regard, the work aims to create an integrated model for evaluating and ranking E-wallet service providers that integrates FAHP with an extent analysis approach and ARAS techniques. The AHP method, established by Saaty in 1980, is a well-known MCDM approach, while the FAHP method is a fuzzy environment generalization of the AHP. The ARAS method is used to prioritize the possibilities. The MCDM technique is simple but effective.
In addition, the suitable alternative should have the highest ratio to the optimal solution [16]. The FAHP technique is used in this study to determine the priority weights of the criteria. As a result, ARAS is used to evaluate the capability of E-wallet service providers. This research provided three contributions to the existing literature. To begin, there has been no research to our experience and understanding that integrates FAHP and ARAS techniques. Furthermore, the ARAS technique has had relatively few economic applications to date.
Finally, to the aimed to contribute, no previous research has been conducted in the area of evaluating E-wallet service providers. As a result, because it is based on pairwise comparisons, the FAHP approach is used in this study to estimate the weights of the major criteria and sub-criteria. The FAHP weights are then combined with the ARAS to examine and rate the E-wallet services provider. A case study of eight E-wallet service providers in Vietnam is also presented to examine the applicability of this developed application. As a result, the findings of this study can fintech companies gain a complete context of their E-wallet services and then evaluate the techniques for achievement. The section of this study is organized as follows to achieve the purpose: The next part discusses the E-wallet and adoption variables, while Section two describes the theoretical background of the FAHP and ARAS techniques. Section three then provides an instructive illustration. The study’s limitations are discussed in the final section. Moreover, the final section concludes with a summary and offers future study opportunities.
Nowadays, E-wallet is widely utilized by many individuals and has become a need to undertake financial transactions. The theoretical frameworks used to analyze factors that influence E-wallet intention have gradually evolved from the theory of planned behavior (TPB) [17], technological acceptance model (TAM) [18], to mobile technology acceptance model (MTAM) [19] and UTAUT [20]. Those research generally employed generic models or changed generic models by adding additional components to develop their own model for exploring the elements that drive behavioral intention. As a result, when extended to mobile environments, the findings do not give a relevant comparison. Accordingly, recent research on E-wallet adoption determinants are included in Tab. 1.
2.2 Benefit of E-Wallet Services
According to studies, fintech is gaining traction in places where the present financial system is unable to meet the need for financial services. In Southeast Asia, the unsatisfied demand for basic banking, payments, and money transfer services is most likely the driving force behind the rapid growth of paytech firms [28]. Paytech enterprises use technological development, changing demographics, regulatory reforms, socio-cultural, socioeconomic, and governmental policy changes, as well as their simpler organizational structures, to adopt new payment systems with better results than traditional banks [10].
E-wallets are electronic wallets that are built on a mobile device (e.g., a smartphone or tablet) that enable individuals to generate mobile banking such as interaccount transfers and online and offline purchases [2,3,29,30]. Some E-wallets also allow users to withdraw cash [3].
Numerous research have lately been conducted on e-wallets and mobile payments. The authors of [4] emphasize the importance of mobile payments for developing nations since they offer financial intermediation of banking services to individuals, ultimately promoting and supporting a range of service provisioning, particularly person-to-person transfers. Despite the fact that mobile payments have been around for a while, Kaur et al. [2] emphasize that E-wallets have introduced a new and more flexible manner of processing payments online. Mumtaza et al. [3] noted that E-wallets, due to their ease of use and favorable influence on noncash transactions, could represent the future of a cashless economy.
The accompanying study objectives are mentioned based on a review of the literature:
i.Numerous study studies on critical factors of E-wallet adoption are available in the prior literature [31–33]. However, only a few papers were able to calculate the influence of the identified important components on the success of E-wallet service uptake using any decision-making technique.
ii.Existing research has identified several success criteria and frameworks. Therefore, fewer publications could indicate the way to the connection between success factor influence E-wallet service providers and their ranking.
iii.A number of articles describing crucial aspects affecting E-wallet service provider adoption and framework are unverified or unconfirmed, throwing doubt on their significance for E-wallet service providers chosen in Vietnam.
iv.A few of the critical factors affecting E-wallet service provider acceptance and framework were investigated through the research. Simultaneously, none of them applied MCDM methodology to boost its practical application.
v.Only certain articles discuss the ranking of E-wallet service providers that have been obtained as a result of the deployment of the digital payment industry. Furthermore, many publications fall short of assessing their intensity through decision-making procedures.
The FAHP technique is combined with the ARAS technique employed to create a novel algorithm fuzzy MCDM model for evaluating E-wallet service providers. To do this, the FAHP technique is used to analyze fuzzy knowledge from expert assessments to determine prioritization weights. The ARAS technique is then used to show the preferred of the alternatives in terms of evaluation factors, resulting in the final rankings of E-wallet service providers. The techniques are thoroughly described in the following sections.
3.1 Fuzzy AHP (FAHP) Technique
There are various ways to determine the weights, such as AHP, SWARA, expert method, individual value method, entropy method, etc. Saaty (1980) designed AHP, one of the most widely used MCDM approaches, to address complex MCDM challenges such as qualitative decisions [34]. AHP studies hierarchical linkages between distinct choice levels without addressing interrelations among factors or alternatives [35]. Decision makers divide the decision process into sub-categories, such as objectives, factors, and options. As a result, when using a hierarchical structure, decision makers use Saaty’s 9-point scale to determine the significance of each component in a pairwise comparison. Furthermore, AHP is a subjective concept that collects information and priority weights of aspects through decision makers’ judgment [36,37]. The decision makers’ opinions and preferences are difficult to evaluate with precise data. In other words, the classical MCDM methodologies do not clarify human thought exactly [13]. Therefore, FST and AHP are integrated into the FAHP model to address ambiguities. To do this, FAHP translates linguistic judgments into Triangular Fuzzy Numbers (TFNs). These matrix multiplications are thus treated to determine the important weights of the elements and alternatives’ ranking [36]. [38] have first employed FAHP by altering the TFN of the FST [39]. Fuzzy sets and FAHP extent analysis method are as follows [40–42].
A fuzzy number is a subset of a fuzzy set
Consider two TFNs
The principal interpretation of Chang’s extent analysis (1992) is that the weights generated by this method do not represent the priority weights of choice criteria or alternatives [44–48]. Although various complaints for Chang’s extent analysis, the great majority of the applications use it. In addition, the extent analysis is utilized because of the ease with which it may be carried out to compute significant weights for this investigation.
Let
where all
Step 1: Define the value of fuzzy synthetic extend regarding the ith object as
To obtain
and to obtain,
and then use Eq. (8) to calculate the inverse of the vector such that:
Step 2: The probability of
and a can be stated similarly as follows:
where d is the latitude of the intersection’s top position D between
Step 3: The degree of probability of a convex fuzzy number being greater than k convex fuzzy number
Assume that:
For
where
Step 4: The normalized weight vectors are obtained using normalization as follows:
where W is a non-fuzzy number.
Figure 1: The intersection between
3.2 The Additive Ratio Assessment Technique
MCDM is generally adopted in scientific fields and economics, and it can make judgment more precise, consistent, and effective [16]. A MCDM technique is strongly related to the task of rating decision alternatives [50]. In this research, the ARAS approach is chosen to rank options. The ARAS approach is focused on basic relative comparisons. Decision criteria define the optimal alternative [51]. In other terms, the ARAS method offers a ratio of each alternative to the optimal option. In the ARAS approach, the value of a utility function is exactly proportional to the relative weights of the primary criteria and alternatives [16]. It suggests comparing the ratio of utility function ratings of examined alternatives to the utility function rating of the optimal alternative. For instance, if the optimal rating of the criterion is 10 points, the alternative with the highest score are 8 points. Evidently, the objective function level of the criterion is 0.8 and not 1.0. Among MCDM approaches, the ARAS technique is the most significant choice for objectively ranking [52]. According to [53], the ARAS method provides a variety of benefits: (i) the calculations are comprehensible, (ii) the concepts are rather logical, (iii) the method is uncomplicated, and (iv) the prioritization weights are determined by comparison. Therefore, the ARAS method is used in this research because it can effectively be applied to a wide variety of decision-making problems; it utilizes a simple mathematical algorithm; and its decision methodology is founded on a direct comparison to the best reasonable option.
In recent years, the ARAS approach has been implemented to the solving of difficult MCDM issues. Historical research based on the ARAS approach are included in Tab. 2.
The ARAS technique procedure consists of the following steps [16,11]:
Step 1: Generating the decision making matrix.
The decision-making matrix shown below is developed.
where m is the number of options, n represents the set of criteria defining each alternative,
Step 2: Normalizing decision-making matrix.
In the second step, the decision making matrix is normalized:
The following criteria, which desirable values are maxima, are normalized:
The following criteria, which desirable values are minima, are normalized:
Step 3: Defining the normalized weighted matrix.
Third, a normalized-weighted matrix is defined
Normalized-weighted values of all criteria are calculated as follows:
where
Step 4: Calculating the values of optimality function
The higher the value of the
Step 5: Deriving the final ranking.
Calculating the utility degree
where
4 Proposed Research Framework for E-Wallet Services Provider Adoption
There are numerous MCDM methods described in the literature. The objective of the problem, available information, decision cost, and decision makers’ qualifications all influence the choice of effective decision-making approaches. Two MCDM approaches are used in this study: ARAS and FAHP. Fig. 2 illustrates the proposed model’s process flow diagram.
There are three stages to the suggested model:
Stage 1: Decision making group working.
Stage 2: Using the FAHP, weigh determined criterion and subcriteria.
Stage 3: Using ARAS, rank the alternatives.
The subsection that follows demonstrates how to use the suggested model to evaluate E-wallet service providers in Vietnam.
Figure 2: The integrated fuzzy model suggested
In the present investigation, eight E-wallet service providers in Vietnam were chosen as a case study. That is, to express the overall E-wallet, service providers in Vietnam have been established as the model’s alternative possibilities. These are Airpay, Grabpay, Moca, Momo, Payoo, Viettelpay, VNPay, and Zalopay.
5.1 Decision Making Group of Expert Opinions
This is an important point in any financial services provider evaluation problem. According to [53,60], in the case of complicated situations, to eliminate bias and reduce partiality, it is frequently preferable to use the perspectives of a group of experts rather than a single individual. Managers now frequently make choices in groups because the complexity and unpredictability of the constraints they must overcome sometimes exceed the capabilities of a single member. The efficacy of a group’s decision-making rests in part on its members’ cooperation in sharing information and thoroughly airing disagreements in assumptions and interpretation. Furthermore, having a say in a decision allows members to feel like decision-makers and validates their position on the judgment team [61]. A decision-making committee comprised of four specialists (customers) and two professionals with over 10 years of experience in the finance sector is formed to establish and analyze the factors influencing E-wallet adoption.
Many scholars have investigated E-wallets from the standpoints of TAM and UTAUT2. As a result, in this study, E-wallet consumer uptake will be attempted to explain based on user views of the technology. This study examines E-wallet service providers based on perceived utility, perceived simplicity of use, and perceived danger, in accordance with the E-wallet adoption factors. In this respect, Tab. 3 lists the criteria and subcriteria addressed in this study, as well as their definitions. Those criteria are compiled from the previous research indicated in Section 2 and are overseen by the decision-making panel. As a result, the model’s hierarchy includes three primary criteria and eight subcriteria.
First, a decision matrix of three primary factors and eight subfactors is generated to determine their relative importance. Next, Chang’s extent analysis converts the fuzzy values to accurate numbers. The accompanying tables will provide a fuzzy evaluation of the criteria. To fulfil this, the fuzzy synthetic extent values and the degree of synthetic extent value are computed. The weight vector is then normalized, as denoted by Eq. (15). Tab. 4 displays the resulting priority weight vector of factors after normalizing the weight vector.
As a result, the final prioritization weights of eight subcriteria SOI, SYQ, FAM, PER, PRR, SER, SEF, and FAC become 0.0223, 0.0539, 0.0632, 0.0688, 0.1429, 0.1467, 0.1876 and 0.3136 respectively. Thus, FAC, SEF, SER, and PRR are the most influential E-wallet adoption factors. Furthermore, SOI gets the lowest weight of 0.0223 for importance. The ARAS approach will use the priority weights.
5.3 ARAS Technique E-Wallet Services Provider Rankings
During this phase of the evaluation of E-wallet service providers, the decision-making matrix is constructed. As a case study, eight E-wallet service providers in Vietnam have been chosen. In addition, each E-wallet adoption element defining these options is determined. The problem is solved using the ARAS algorithm described in Section 3, and the results are provided in Tabs. 5–7.
Tabulated evaluations of the alternatives (Tab. 5), factor values, and priority weights are included in the initial decision-making matrix. As shown in Tab. 5, the adoption factors “Facilitating Conditions”, “Self-efficacy”, “Privacy Risk” and “Security Risk” have the highest priority weight. As described in Section 3, the initial decision-making matrix is then normalized. Tab. 6 shows the decision-making matrix that has been standardized. Tab. 7 depicts the subsequent construction of the weighted decision-making matrix. Each alternative’s optimality function
Given the outcomes of the solutions E-wallet service provider ranks as follows:
Consequently, the evaluation findings suggest that momo, with a utility degree (performance ratio) of 74.60%, is the top choice. According to Tab. 7, Viettelpay is the second-ranked E-wallet services provider with a utility degree of 57.34%. With 55.14% utility, Payoo is the third-ranked E-wallet services provider in Vietnam. Finally, Grabpay and Zalopay, with respective utility degrees of 49.34% and 43.40%, are chosen as the fourth and fifth E-wallet service provider. However, Vnpay, Moca, and Airpay with utility scores of 40.51%, 36.80%, and 35.23%.
5.4 The Sensitivity Analysis of Weight Information
A sensitivity analysis is used in this study to examine how the rank order of E-wallet service providers changes when the priority weights of the criteria are altered. To do this, the priority weights for two criteria are modified while the others remain constant. For example, the priority weight of the SOI is adjusted successively to SYO, SEF, FAC, and so on, while the others remain unchanged. Tab. 8 presents the situations that were considered. While the priority weights change, the utility value of alternatives changes. If the priority weights of SOI and SYQ are swapped in Scenario 1, the utility value of p4 falls from 0.7460 to 0.7450 and p6 rises from 0.5734 to 0.5764. As a result, except for Scenario 8, the preference ranking of p4 does not change. Furthermore, according to the sensitivity analysis results, p6 is considered the best alternative in all situations except Scenario 8 because it has the highest utility value following the priority weight exchange performed here. Fig. 3 illustrates a sensitivity study used to determine the ranking of E-wallet service providers.
Figure 3: Sensitivity analysis
The FAHP approach is used to establish the weights of the criterion in this study. The ARAS approach is used to analyze alternatives to rank them and choose the most E-wallet service provider. According to the findings of this study, the most important factor influencing E-wallet service provider adoption is the facilitating condition (FAC). The outcomes of this study are also similar with prior research [19,21,22,69]. This illustrates that the more customers believe they can utilize e-wallets and mobile devices available to them, the more probable it is that they will continue to use E-wallets. This also signifies that in Vietnam, the basic requirements for using an E-wallet are met. As a result, it is recommended that clients be supplied with proper information on E-wallets and their benefits.
The second factor influencing the adoption of E-wallet service providers is self-efficacy (SEF). According to [22], one of the most crucial variables influencing the client’s adoption of an E-wallet is self-efficacy. Furthermore, in [17] study, self-efficacy is a crucial E-wallet adoption factor. Consequently, financial services executives, entrepreneurs, and professionals should prioritize self-efficacy development.
Security risk (SER) and privacy risk (PRR) are the third and fourth factors influencing E-wallet usage. These factors are perceived by clients as significant impediments to the adoption of E-wallet services. In other terms, the relatively high customers’ perceptions of the privacy and security risks associated with E-wallet services, the less likely they are to use this new channel. As a result, to guarantee that their financial transactions are secure and that the overall E-wallet service is operating, financial services providers must manage the risk issue [70]. Furthermore, studies on E-wallet use have examined privacy and security risks. This finding is as follows, identified by a previous studies, which revealed that privacy and security risks had a strong significant correlation with behavioral intentions [68,70–76].
Another factor influencing E-wallet adoption is performance risk (PER), which was identified as the sixth element in this study. This finding backs with Zhou’s [19] and Chen’s [70] findings that performance risk is a major barrier to E-wallet adoption. As a result, if a person perceives that an E-wallet service does not suit his or her needs (i.e., transfer speeds, transaction length, etc.), he or she is unlikely to use it. It is advised in this regard that E-wallet systems can be constructed in such a way that they can be enhanced in performance risk and utilized confidingly by diverse clients. To do this, bank practitioners must advocate for a reduction in performance risk with zeal. As a result, the more customers perceive the E-wallet service is underperforming, the less likely they are to use and adopt it.
The following factor influencing E-wallet adoption is familiarity (FAM), which is revealed as the eighth factor in E-wallet adoption. The premise that familiarity favorably affects perceived ease of use was confirmed in the [21] study. Customers will consider it as simple to use because they are familiar with E-wallet services. As a result of experience, familiarity enhances the likelihood of continuing to utilize E-wallet services. According to the findings, system quality is an issue (SYQ). According to other studies [21,76], system quality influences continued use. For example, the authors in [21] found that system quality had a significant impact on clients’ willingness to continue using E-wallets in Korea. As a result, system quality has an impact not only on adoption behavior but also on customer satisfaction. Customers will regard E-wallet services as valuable if they are dependable, flexible, accessible, accurate, and timely.
Finally, as an eighth component in E-wallet adoption, social impact (SOI) is discovered. According to [19], social influence has a major impact on user adoption. Furthermore, these findings are consistent with the findings of [27]. As a result, new advertising strategies, society, and social networks may impact Vietnamese clients, and these interactions may influence their decisions.
The case study further demonstrates that the proposed paradigm is straightforward and appropriate for dealing with complex E-wallet concerns. The combination of FAHP and ARAS demonstrated that Momo is the best E-wallet service provider. In addition, the suggested FAHP&ARAS model specifies the performance ratio of each E-wallet service option to the ideal E-wallet service alternative. Momo’s performance is about 74% of the performance of the best E-wallet services provider.
In today’s world, e-wallets are the main digital payment method. Thus, E-wallet customers consider it a necessity, not an option. Customers are also expected to improve the service quality of financial service providers. Non-banking providers have already invested in E-wallets, increasing their investments due to expanding user numbers. In Vietnam, around one-fifth of transactions are conducted via application, so expanding mobile transaction sets will enhance mobile share. Other E-wallet service providers failed to gain a foothold in the market and fell behind. Suppliers must increase perks and service quality to e-wallet consumers. E-wallet service providers who understand and meet customer expectations will have a competitive advantage.
Many business difficulties involve accurate modeling of conflicting viewpoints, multiple criteria, and the subjective nature of the judgment process. As a result, multicriteria evaluation helps the business field by determining priority weights, selecting the best alternative, and disclosing all options. ARAS as an MCDM method allows decision makers to identify the optima alternative by combining both qualitative and quantitative choice criteria in the evaluation process. For this reason, the ARAS technique is used to assess the expert estimations’ significance. That is, the suggested paradigm allows considering all decision-making team members’ perspectives. The ARAS method is then used to determine the best E-wallet services. The priority weights of factors affecting E-wallet adoption in Vietnam are assessed in this study. The data shows that facilitating conditions, self-efficacy, privacy risk, and security risk are the most essential elements of E-wallet adoption. It means that perceived simplicity of use is recognized as the most important dimension influencing E-wallet service acceptability and that it should be prioritized and improved. Momo is ranked as the finest E-wallet service provider in Vietnam. However, Vnpay is considered the worst E-wallet provider.
Acknowledgement: The author extends their appreciation to the Posts and Telecommunications Institute of Technology (PTIT) in Vietnam for funding this research work.
Funding Statement: The author wishes to express their gratitude to PTIT, Vietnam, for financial support for this research. (https://www.ptit.edu.vn/)
Conflicts of Interest: The author declares that they have no conflicts of interest to report regarding the present study.
References
1. D. Canzano and M. Grimaldi, “An integrated framework to implement a knowledge management programme: The role of technological tools and techniques,” International Journal of Intelligent Enterprise, vol. 1, no. 3–4, pp. 233–247, 2012. [Google Scholar]
2. P. Kaur, A. Dhir, R. Bodhi, T. Singh and M. Almotairi, “Why do people use and recommend m-wallets?” Journal of Retailing and Consumer Services, vol. 56, pp. 1–11, 2020. [Google Scholar]
3. Q. M. H. Mumtaza, S. I. Nabillah, S. Amaliya, Y. Rosabella and J. A. Hammad, “Worldwide mobile wallet: A futuristic cashless system,” Bulletin of Social Informatics Theory and Application, vol. 4, no. 2, pp. 70–75, 2020. [Google Scholar]
4. N. Iman, “Is mobile payment still relevant in the fintech era?” Electronic Commerce Research and Applications, vol. 30, pp. 72–82, 2018. [Google Scholar]
5. C. Unger, D. Murthy, A. Acker, I. Arora and A. Chang, “Examining the evolution of mobile social payments in venmo,” in ACM Int. Conf. Proc. Series, New York, USA, pp. 101–110, 2020. [Google Scholar]
6. Surtikanti and R. H. Mustofa, “Utilization of electronic money,” in IOP Conf. Series: Materials Science and Engineering, Bristol, UK, vol. 662, no. 2, 2019. [Google Scholar]
7. M. Karsen, Y. U. Chandra and H. Juwitasary, “Technological factors of mobile payment: A systematic literature review,” Procedia Computer Science, vol. 157, pp. 489–498, 2019. [Google Scholar]
8. C. Cantú and B. Ulloa, “The dawn of fintech in Latin America: Landscape, prospects and challenges,” BIS Papers, vol. 112, no. 11, 1–44, 2020. [Google Scholar]
9. P. Nguyen and H. Tran, “Behavioural intention to accept mobile wallet: An empirical study of a developing country perspective,” International Journal of Information Systems and Supply Chain Management, vol. 12, no. 5, pp. 93–107, 2019. [Google Scholar]
10. M. Polasik, A. Huterska, R. Iftikhar and Š. Mikula, “The impact of payment services directive 2 on the PayTech sector development in Europe,” Journal of Economic Behavior and Organization, vol. 178, pp. 385–401, 2020, https://doi.org/10.1016/j.jebo.2020.07.010. [Google Scholar]
11. S. Sušinskas, E. K. Zavadskas and Z. Turskis, “Multiple criteria assessment of pile-columns alternatives,” Baltic Journal of Road and Bridge Engineering, vol. 6, no. 3, pp. 145–152, 2011. [Google Scholar]
12. L. A. Zadeh, “Fuzzy sets,” Information and Control, vol. 8, no. 3, pp. 338–353, 1965. [Google Scholar]
13. W. C. Chou and Y. P. Cheng, “A hybrid fuzzy MCDM approach for evaluating website quality of professional accounting firms,” Expert Systems with Applications, vol. 39, no. 3, pp. 2783–2793, 2012. [Google Scholar]
14. L. Y. Chen and T. C. Wang, “Optimizing partners’ choice in IS/IT outsourcing projects: The strategic decision of fuzzy VIKOR,” International Journal of Production Economics, vol. 120, no. 1, pp. 233–242, 2009. [Google Scholar]
15. C. Kahraman, U. Cebeci and D. Ruan, “Multi-attribute comparison of catering service companies using fuzzy AHP: The case of Turkey,” International Journal of Production Economics, vol. 87, no. 2, pp. 171–184, 2004. [Google Scholar]
16. E. K. Zavadskas and Z. Turskis, “A new additive ratio assessment (ARAS) method in multicriteria decision-making,” Technological and Economic Development of Economy, vol. 16, no. 2, pp. 159–172, 2010. [Google Scholar]
17. P. Luarn and H. H. Lin, “Toward an understanding of the behavioral intention to use mobile banking,” Computers in Human Behavior, vol. 21, no. 6, pp. 873–891, 2005. [Google Scholar]
18. J. Lu, C. S. Yu, C. Liu and J. E. Yao, “Technology acceptance model for wireless internet,” Internet Research, vol. 13, no. 3, pp. 206–222, 2003. [Google Scholar]
19. T. Zhou, Y. Lu and B. Wang, “Integrating TTF and UTAUT to explain mobile banking user adoption,” Computers in Human Behavior, vol. 26, no. 4, pp. 760–767, 2010. [Google Scholar]
20. A. de la Concha, S. Martinez-Jaramillo and C. Carmona, “Multiplex financial networks: Revealing the level of interconnectedness in the banking system,” In: C. C., C. H., K. M., and M. M. (EdsStudies in Computational Intelligence, Cham: Springer, vol. 689, pp. 1135–1148, 2018. [Google Scholar]
21. J. C. Gu, S. C. Lee and Y. H. Suh, “Determinants of behavioral intention to mobile banking,” Expert Systems with Applications, vol. 36, no. 9, pp. 11605–11616, 2009. [Google Scholar]
22. J. Püschel, J. A. Mazzon and J. M. C. Hernandez, “Mobile banking: Proposition of an integrated adoption intention framework,” International Journal of Bank Marketing, vol. 28, no. 5, pp. 389–409, 2010. [Google Scholar]
23. H. E. Riquelme and R. E. Rios, “The moderating effect of gender in the adoption of mobile banking,” International Journal of Bank Marketing, vol. 28, no. 5, pp. 328–341, 2010. [Google Scholar]
24. H. F. Lin, “An empirical investigation of mobile banking adoption: The effect of innovation attributes and knowledge-based trust,” International Journal of Information Management, vol. 31, no. 3, pp. 252–260, 2011. [Google Scholar]
25. D. Zheng, J. Chen, L. Huang and C. Zhang, “E-government adoption in public administration organizations: Integrating institutional theory perspective and resource-based view,” European Journal of Information Systems, vol. 22, no. 2, pp. 221–234, 2013. [Google Scholar]
26. P. Hanafizadeh, M. Behboudi, A. Abedini Koshksaray and M. Jalilvand Shirkhani Tabar, “Mobile-banking adoption by Iranian bank clients,” Telematics and Informatics, vol. 31, no. 1, pp. 62–78, 2014. [Google Scholar]
27. R. Bidar, M. B. Fard, Y. B. Salman, M. A. Tunga and H. I. Cheng, “Factors affecting the adoption of mobile banking: Sample of Turkey,” in Int. Conf. on Advanced Communication Technology, ICACT, PyeongChang, Korea, pp. 1278–1282, 2014, https://doi.org/10.1109/ICACT.2014.6779165. [Google Scholar]
28. J. Frost, “The economic forces driving fintech adoption across countries,” The Technological Revolution in Financial Services: How Banks, FinTechs, and Customers Win Together, vol. 838, pp. 70–89, 2020, https://doi.org/10.2139/ssrn.3515326. [Google Scholar]
29. Z. Bezovski, “The future of the mobile payment as electronic payment system,” European Journal of Business and Management, vol. 8, no. 8, pp. 127–132, 2016. [Google Scholar]
30. Financial Stability Board, “Financial stability implications from fintech,” no. June. Financial Stability Board, 2017. [Google Scholar]
31. N. Tura, J. Hanski, T. Ahola, M. Ståhle, S. Piiparinen et al., “Unlocking circular business: A framework of barriers and drivers,” Journal of Cleaner Production, vol. 212, no. 90, pp. 98, 2019. [Google Scholar]
32. A. De Jesus and S. Mendonça, “Lost in transition? Drivers and barriers in the ecoinnovation road to the circular economy,” Ecological Economics, vol. 145, pp. 75–89, 2018. [Google Scholar]
33. K. Govindan and M. Hasanagic, “A systematic review on drivers, barriers, and practices towards circular economy: A supply chain perspective,” International Journal of Production Research, vol. 56, pp. 278–311, 2018, https://doi.org/10.1080/00207543.2017.1402141. [Google Scholar]
34. F. Ecer, “A hybrid banking websites quality evaluation model using AHP and COPRAS-G: A Turkey case,” Technological and Economic Development of Economy, vol. 20, no. 4, pp. 758–782, 2014. [Google Scholar]
35. F. A. Nouri, S. K. Esbouei and J. Antucheviciene, “A hybrid MCDM approach based on fuzzy ANP and fuzzy TOPSIS for technology selection,” Informatica (Netherlands), vol. 26, no. 3, pp. 369–388, 2015. [Google Scholar]
36. A. Calabrese, R. Costa and T. Menichini, “Using fuzzy AHP to manage intellectual capital assets: An application to the ICT service industry,” Expert Systems with Applications, vol. 40, no. 9, pp. 3747–3755, 2013. [Google Scholar]
37. M. C. Das, B. Sarkar and S. Ray, “A framework to measure relative performance of Indian technical institutions using integrated fuzzy AHP and COPRAS methodology,” Socio-Economic Planning Sciences, vol. 46, no. 3, pp. 230–241, 2012. [Google Scholar]
38. P. J. M. van Laarhoven and W. Pedrycz, “A fuzzy extension of Saaty’s priority theory,” Fuzzy Sets and Systems, vol. 11, no. 1–3, pp. 229–241, 1983. [Google Scholar]
39. E. Bulut, O. Duru, T. Keçeci and S. Yoshida, “Use of consistency index, expert prioritization and direct numerical inputs for generic fuzzy-AHP modeling: A process model for shipping asset management,” Expert Systems with Applications, vol. 39, no. 2, pp. 1911–1923, 2012. [Google Scholar]
40. M. Tavana, E. Momeni, N. Rezaeiniya, S. M. Mirhedayatian and H. Rezaeiniya, “A novel hybrid social media platform selection model using fuzzy ANP and COPRAS-G,” Expert Systems with Applications, vol. 40, no. 14, pp. 5694–5702, 2013. [Google Scholar]
41. M. H. Aghdaie, S. H. Zolfani and E. K. Zavadskas, “Market segment evaluation and selection based on application of fuzzy AHP and COPRAS-G methods,” Journal of Business Economics and Management, vol. 14, no. 1, pp. 213–233, 2013. [Google Scholar]
42. F. Ecer, “Performance evaluation of internet banking branches via a hybrid MCDM model under fuzzy environment,” Economic Computation and Economic Cybernetics Studies and Research, vol. 49, no. 2, pp. 1–19, 2015. [Google Scholar]
43. A. Calabrese, R. Costa, N. Levialdi and T. Menichini, “A fuzzy analytic hierarchy process method to support materiality assessment in sustainability reporting,” Journal of Cleaner Production, vol. 121, pp. 248–264, 2016, https://doi.org/10.1016/j.jclepro.2015.12.005. [Google Scholar]
44. K. J. Zhu, Y. Jing and D. Y. Chang, “Discussion on extent analysis method and applications of fuzzy AHP,” European Journal of Operational Research, vol. 116, no. 2, pp. 450–456, 1999. [Google Scholar]
45. L. C. Leung and D. Cao, “On consistency and ranking of alternatives in fuzzy AHP,” European Journal of Operational Research, vol. 124, no. 1, pp. 102–113, 2000. [Google Scholar]
46. L. Mikhailov, “Deriving priorities from fuzzy pairwise comparison judgements,” Fuzzy Sets and Systems, vol. 134, no. 3, pp. 365–385, 2003. [Google Scholar]
47. Y. M. Wang, Y. Luo and Z. Hua, “On the extent analysis method for fuzzy AHP and its applications,” European Journal of Operational Research, vol. 186, no. 2, pp. 735–747, 2008. [Google Scholar]
48. Y. M. Wang and K. S. Chin, “Fuzzy analytic hierarchy process: A logarithmic fuzzy preference programming methodology,” International Journal of Approximate Reasoning, vol. 52, no. 4, pp. 541–553, 2011. [Google Scholar]
49. D. Y. Chang, “Applications of the extent analysis method on fuzzy AHP,” European Journal of Operational Research, vol. 95, no. 3, pp. 649–655, 1996. [Google Scholar]
50. S. Dadelo, Z. Turskis, E. K. Zavadskas and R. Dadeliene, “Multiple criteria assessment of elite security personal on the basis of ARAS and expert methods,” Economic Computation and Economic Cybernetics Studies and Research, vol. 4, no. 4, pp. 65–87, 2012. [Google Scholar]
51. V. Kutut, E. K. Zavadskas and M. Lazauskas, “Assessment of priority options for preservation of historic city centre buildings using MCDM (ARAS),” Procedia Engineering, vol. 57, pp. 657–661, 2013, https://doi.org/10.1016/j.proeng.2013.04.083. [Google Scholar]
52. J. Sliogeriene, Z. Turskis and D. Streimikiene, “Analysis and choice of energy generation technologies: The multiple criteria assessment on the case study of Lithuania,” Energy Procedia, vol. 32, pp. 11–20, 2013, https://doi.org/10.1016/j.egypro.2013.05.003. [Google Scholar]
53. M. Zamani, A. Rabbani, A. Yazdani-Chamzini and Z. Turskis, “An integrated model for extending brand based on fuzzy ARAS and ANP methods,” Journal of Business Economics and Management, vol. 15, no. 3, pp. 403–423, 2014. [Google Scholar]
54. T. Bakshi and B. Sarkar, “MCA based performance evaluation of project selection,” International Journal of Software Engineering & Applications, vol. 2, no. 2, pp. 14–22, 2011. [Google Scholar]
55. D. Štreimikienė and A. Baležentis, “Integrated sustainability index: The case study of Lithuania,” Intellectual Economics, vol. 7, no. 3, pp. 289–303, 2013. [Google Scholar]
56. R. Sheikh and A. Majid, “Ranking financial institutions based on of trust in online banking using ARAS and ANP method,” International Research Journal of Applied and Basic Sciences, vol. 6, no. 4, pp. 415–423, 2013. [Google Scholar]
57. P. Chatterjee and S. Chakraborty, “Gear material selection using complex proportional assessment and additive ratio assessment-based approaches: A comparative study,” International Journal of Materials Science and Engineering, vol. 1, no. 2, pp. 104–111, 2013. [Google Scholar]
58. M. Medineckiene, E. K. Zavadskas, F. Björk and Z. Turskis, “Multi-criteria decision-making system for sustainable building assessment/certification,” Archives of Civil and Mechanical Engineering, vol. 15, no. 1, pp. 11–18, 2015. [Google Scholar]
59. F. Ecer, “ARAS yöntemi kullanılarak kurumsal kaynak planlaması yazılımı seçimi,” Uluslararası Alanya İşletme Fakültesi Dergisi, vol. 8, no. 1, pp. 89–98, 2016. [Google Scholar]
60. R. U. Bilsel, G. Büyüközkan and D. Ruan, “A fuzzy preference-ranking model for a quality evaluation of hospital Web sites,” International Journal of Intelligent Systems, vol. 21, no. 11, pp. 1181–1197, 2006. [Google Scholar]
61. M. A. Korsgaard, D. M. Schweiger and H. J. Sapienza, “Building commitment, attachment, and trust in strategic decision-making teams: The role of procedural justice,” Academy of Management Journal, vol. 38, no. 1, pp. 60–84, 1995. [Google Scholar]
62. V. Venkatesh and H. Bala, “Adoption and impacts of interorganizational business process standards: Role of partnering synergy,” Information Systems Research, vol. 23, no. 4, pp. 1131–1157, 2012. [Google Scholar]
63. F. D. Davis, “Perceived usefulness, perceived ease of use, and user acceptance of information technology,” MIS Quarterly: Management Information Systems, vol. 13, no. 3, pp. 319–339, 1989. [Google Scholar]
64. V. Venkatesh, “Determinants of perceived ease of use: Integrating control, intrinsic motivation, and emotion into the technology acceptance model,” Information Systems Research, vol. 11, no. 4, pp. 342–365, 2000. [Google Scholar]
65. R. J. Hill, M. Fishbein and I. Ajzen, Belief, Attitude, Intention and Behavior: An Introduction to Theory and Research, vol. 6, no. 2. Washington: Addison-Wesley, 1977. [Google Scholar]
66. D. WH and M. ER, “The DeLone and McLean model of information systems success: A ten-year update,” Journal of Management Information Systems, vol. 19, no. 4, pp. 9–30, 2003. [Google Scholar]
67. S. T. J. -D. Lovelock, J. Hare, A. Woodward and A. Priestley, “Forecast: The business value of artificial intelligence, worldwide, 2017–2025,” Gartner, Research note G00348137, pp. 1–22, 2018. [Google Scholar]
68. U. Akturan and N. Tezcan, “Mobile banking adoption of the youth market: Perceptions and intentions,” Marketing Intelligence and Planning, vol. 30, no. 4, pp. 444–459, 2012. [Google Scholar]
69. G. Kim, B. Shin and H. G. Lee, “Understanding dynamics between initial trust and usage intentions of mobile banking,” Information Systems Journal, vol. 19, no. 3, pp. 283–311, 2009. [Google Scholar]
70. C. S. Chen, “Perceived risk, usage frequency of mobile banking services,” Managing Service Quality, vol. 23, no. 5, pp. 410–436, 2013. [Google Scholar]
71. K. S. Lee, H. S. Lee and S. Y. Kim, “Factors influencing the adoption behavior of mobile banking: A South Korean perspective,” The Journal of Internet Banking and Commerce, vol. 2007, no. 2, pp. 1–9, 2015. [Google Scholar]
72. T. Laukkanen, “Internet vs. mobile banking: Comparing customer value perceptions,” Business Process Management Journal, vol. 13, no. 6, pp. 788–797, 2007. [Google Scholar]
73. P. Cruz, L. B. F. Neto, P. Muñoz-Gallego and T. Laukkanen, “Mobile banking rollout in emerging markets: Evidence from Brazil,” International Journal of Bank Marketing, vol. 28, no. 5, pp. 342–371, 2010. [Google Scholar]
74. N. Koenig-Lewis, A. Palmer and A. Moll, “Predicting young consumers’ take up of mobile banking services,” International Journal of Bank Marketing, vol. 28, no. 5, pp. 410–432, 2010. [Google Scholar]
75. L. Wessels and J. Drennan, “An investigation of consumer acceptance of M-banking,” International Journal of Bank Marketing, vol. 28, no. 7, pp. 547–568, 2010. [Google Scholar]
76. S. Negash, “Mobile banking adoption by under-banked communities in the United States: Adapting mobile banking features from low-income countries,” in Proc.-2011 10th Int. Conf. on Mobile Business, ICMB 2011, Como, Italy, pp. 205–209, 2011. [Google Scholar]
Cite This Article
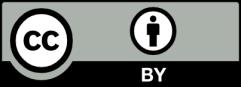
This work is licensed under a Creative Commons Attribution 4.0 International License , which permits unrestricted use, distribution, and reproduction in any medium, provided the original work is properly cited.