Open Access
ARTICLE
Image-Based Automatic Energy Meter Reading Using Deep Learning
1 Institute of Computer Sciences and Information Technology, Faculty of Management and Computer Sciences, The University of Agriculture, Peshawar, Pakistan
2 Department of Electrical and Computer Engineering, COMSATS University Islamabad, Attock Campus, Attock City, Pakistan
3 Kuwait College of Science and Technology, Kuwait City, Kuwait
4 School of Electrical & Electronic Engineering, USM Engineering Campus, Universiti Sains Malaysia, Penang, 14300, Malaysia
* Corresponding Author: Muhammad Imran. Email:
Computers, Materials & Continua 2023, 74(1), 203-216. https://doi.org/10.32604/cmc.2023.029834
Received 12 March 2022; Accepted 02 June 2022; Issue published 22 September 2022
Abstract
We propose to perform an image-based framework for electrical energy meter reading. Our aim is to extract the image region that depicts the digits and then recognize them to record the consumed units. Combining the readings of serial numbers and energy meter units, an automatic billing system using the Internet of Things and a graphical user interface is deployable in a real-time setup. However, such region extraction and character recognition become challenging due to image variations caused by several factors such as partial occlusion due to dust on the meter display, orientation and scale variations caused by camera positioning, and non-uniform illumination caused by shades. To this end, our work evaluates and compares the state-of-the art deep learning algorithm You Only Look Once (YOLO ) along with traditional handcrafted features for text extraction and recognition. Our image dataset contains 10,000 images of electrical energy meters and is further expanded by data augmentation such as in-plane rotation and scaling to make the deep learning algorithms robust to these image variations. For training and evaluation, the image dataset is annotated to produce the ground truth of all the images. Consequently, YOLO achieves superior performance over the traditional handcrafted features with an average recognition rate of 98% for all the digits. It proves to be robust against the mentioned image variations compared with the traditional handcrafted features. Our proposed method can be highly instrumental in reducing the time and effort involved in the current meter reading, where workers visit door to door, take images of meters and manually extract readings from these images.Keywords
Cite This Article
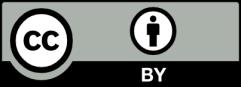