Open Access
ARTICLE
Attack Behavior Extraction Based on Heterogeneous Cyberthreat Intelligence and Graph Convolutional Networks
1 School of Cyber Science and Engineering, Sichuan University, Chengdu, 610065, China
2 College of Computer Science, Sichuan University, Chengdu, 610065, China
3 Jincheng College of Sichuan University, Chengdu, 610065, China
4 Institute for Infocomm Research, A*STAR Singapore, Singapore
* Corresponding Author: Junfeng Wang. Email:
Computers, Materials & Continua 2023, 74(1), 235-252. https://doi.org/10.32604/cmc.2023.029135
Received 26 February 2022; Accepted 08 June 2022; Issue published 22 September 2022
Abstract
The continuous improvement of the cyber threat intelligence sharing mechanism provides new ideas to deal with Advanced Persistent Threats (APT). Extracting attack behaviors, i.e., Tactics, Techniques, Procedures (TTP) from Cyber Threat Intelligence (CTI) can facilitate APT actors’ profiling for an immediate response. However, it is difficult for traditional manual methods to analyze attack behaviors from cyber threat intelligence due to its heterogeneous nature. Based on the Adversarial Tactics, Techniques and Common Knowledge (ATT&CK) of threat behavior description, this paper proposes a threat behavioral knowledge extraction framework that integrates Heterogeneous Text Network (HTN) and Graph Convolutional Network (GCN) to solve this issue. It leverages the hierarchical correlation relationships of attack techniques and tactics in the ATT&CK to construct a text network of heterogeneous cyber threat intelligence. With the help of the Bidirectional Encoder Representation from Transformers (BERT) pretraining model to analyze the contextual semantics of cyber threat intelligence, the task of threat behavior identification is transformed into a text classification task, which automatically extracts attack behavior in CTI, then identifies the malware and advanced threat actors. The experimental results show that F1 achieve 94.86% and 92.15% for the multi-label classification tasks of tactics and techniques. Extend the experiment to verify the method’s effectiveness in identifying the malware and threat actors in APT attacks. The F1 for malware and advanced threat actors identification task reached 98.45% and 99.48%, which are better than the benchmark model in the experiment and achieve state of the art. The model can effectively model threat intelligence text data and acquire knowledge and experience migration by correlating implied features with a priori knowledge to compensate for insufficient sample data and improve the classification performance and recognition ability of threat behavior in text.Keywords
Cite This Article
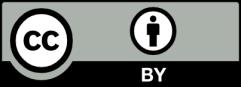
This work is licensed under a Creative Commons Attribution 4.0 International License , which permits unrestricted use, distribution, and reproduction in any medium, provided the original work is properly cited.