Open Access
ARTICLE
Multivariate Aggregated NOMA for Resource Aware Wireless Network Communication Security
1 ECE Department, Vidya Jyothi Institute of Technology, India
2 CSE Department, Neil Gogte Institute of Technology, India
3 School of Digital Science, Universiti Brunei Darussalam, JalanTungku Link, Gadong BE1410, Brunei Darussalam
4 Department of Information and Communication Technology, University of Agder (UiA), N-4898 Grimstad, Norway
5 Department of Computer Science, College of Computers and Information Technology, Taif University, P. O. Box 11099, Taif 21944, Saudi Arabia
6 Department of Information Technology, College of Computer and Information Sciences, Princess Nourahbint Abdulrahman University, P.O. Box 84428, Riyadh 11671, Saudi Arabia
7 Department of Information Technology, College of Computing and Informatics, Saudi Electronic University, 93499, Riyadh, Saudi Arabia
* Corresponding Author: Saddam Hussain. Email:
Computers, Materials & Continua 2023, 74(1), 1693-1708. https://doi.org/10.32604/cmc.2023.028129
Received 03 February 2022; Accepted 05 April 2022; Issue published 22 September 2022
Abstract
Nonorthogonal Multiple Access (NOMA) is incorporated into the wireless network systems to achieve better connectivity, spectral and energy effectiveness, higher data transfer rate, and also obtain the high quality of services (QoS). In order to improve throughput and minimum latency, a Multivariate Renkonen Regressive Weighted Preference Bootstrap Aggregation based Nonorthogonal Multiple Access (MRRWPBA-NOMA) technique is introduced for network communication. In the downlink transmission, each mobile device's resources and their characteristics like energy, bandwidth, and trust are measured. Followed by, the Weighted Preference Bootstrap Aggregation is applied to recognize the resource-efficient mobile devices for aware data transmission by constructing the different weak hypotheses i.e., Multivariate Renkonen Regression functions. Based on the classification, resource and trust-aware devices are selected for transmission. Simulation of the proposed MRRWPBA-NOMA technique and existing methods are carried out with different metrics such as data delivery ratio, throughput, latency, packet loss rate, and energy efficiency, signaling overhead. The simulation results assessment indicates that the proposed MRRWPBA-NOMA outperforms well than the conventional methods.Keywords
In the wireless mobile communication networks, outstanding challenges are involved to be met such as a great deal to obtain higher data delivery, spectrum utilization, lower latency, and better connection. Therefore, the NOMA technology has been newly put forward as a promising model to accomplish much better spectral efficiency compared to traditional orthogonal access techniques employed in wireless communication systems. A most important feature of NOMA is to allow many users to transmit and receive their data in the same time and frequency domain. A machine technique is applied to NOMA technology to achieve efficient communication with higher throughput and minimum latency.
A Solution Procedure for Joint Subcarrier Assignment, Time-Switching, and Power Allocation SP-J-SA-TS-PA (NOMA) scheme was developed in [1] for wireless information and power transmission to improve the coverage and throughput. But the scheme was not efficient to reduce the latency of wireless information. A code-domain NOMA and power-domain NOMA (CD/PD-NOMA) were developed in [2] for improving the secrecy of data transmission. But the resource-aware data communication in NOMA technology was not performed.
The fundamental theory of NOMA has been analyzed in [3] to improve the user data rate, system capacity, and reception complexity. But the security-aware data information was not performed in the multiple access technique. An online energy consumption minimization (OECM) method was designed in [4] to minimize the latency constraints. The nonorthogonal multiple access (NOMA) technique was also employed to solve the preamble collision where more devices access the same network. But the designed algorithm failed to analyze the multiple resources to improve the data delivery.
Two-tier heterogeneous networks with NOMA framework were introduced in [5] for improving the dynamic communication based on resource allocation. But the designed framework failed to perform privacy of data in dynamic communication. A secure non-orthogonal multiple access in joint energy-harvesting (EH) communications was introduced [6]. But while increasing the number of mobile users increases, the system security gets degraded. A joint optimization approach was developed in [7] for enhancing the system energy efficiency maximization. But the performance of the throughput maximization was not achieved.
A trusted cooperative half-duplex relay has been introduced [8] for secure communication in a downlink NOMA system. But the latency-aware secure communication was not reduced. A novel relay-assisted non-orthogonal multiple access (NOMA) method was developed in [9] for multi-cell uplink cellular networks. The designed method significantly reduces the control overhead but the throughput of uplink cellular networks was not maximized.
A deep-learning-based technique was introduced in [10] for allowing many users to utilize the full bandwidth. The designed method increases the high bandwidth efficiency but the latency was not minimized.
The main contributions of the paper are summarized as follows:
• In order to achieve resource and secure data communication, an MRRWPBA-NOMA technique is introduced in the downlink transmission of the mobile network.
• The Weighted Preference Bootstrap Aggregation is applied in the MRRWPBA-NOMA technique to find the best mobile device for resource-efficient data transmission with help of several weak hypotheses. On the contrary conventional regression methods, the proposed regression function analyzes the resources and trust with the threshold based on the Renkonen similarity index. As a result, resource-aware and trusted devices are identified to enhance efficient data communication and minimize latency.
• Simulation of the proposed MRRWPBA-NOMA technique and existing methods are carried out and illustrated the better results for all the parameters.
Recently, several types of research have considered NOMA networks, and the existing literature can be reviewed according to resource and security models.
A maximal harvested energy (MHE) scheme and best cooperative path (BCP) approach was introduced in [11] to perform the relay selection with better energy harvesting. But the latency-aware relay selection was not performed. A cognitive NOMA network was introduced in [12] for enhancing the throughput. But the trust-aware NOMA network was not designed.
Power domain-NOMA (PD-NOMA) was introduced [13] for increasing the performance and better spectral utilization in downlink cooperative networks. But the NOMA-based cooperative networks were not designed. A multi-antenna cooperative NOMA approach was introduced in [14] to enhance the normal power performance. But the approach failed to minimize the computational complexity.
A joint design on beamforming vectors and artificial noise (AN) covariance matrix were introduced in [15] for the NOMA system to minimize the complexity. But the trust-based secure NOMA system was not designed to improve confidential data transmission. Physical layer security based on NOMA was presented in [16] to achieve a higher secrecy rate. But the analytical optimization of the power allocation factors was not considered.
A secure and accurate Channel State Information (CSI) was presented in [17] for improving privacy performance. The model increases the average secrecy throughput but it failed to protect the uplink feedback security. In order to significantly improved the performance of the NOMA system, EXIT charts analysis was presented in [18]. A centralized energy-efficiency maximizing power allocation scheme was developed in [19]. But, a higher throughput was not obtained. An optimized power role switching-NOMA (OPRS-NOMA) method was introduced in [20] for mobile users. But the reliable data transmission was not achieved.
Tab. 1 shows the summary of the above literature.
3 Multivariate Renkonen Regressive Weighted Preference Bootstrap Aggregation Based NOMA for Resource-Aware Secure Communication
Recently, the exponential growth of Smartphone and mobile users has direct to the increasing demand for high-speed data access. The data communication with non-orthogonal multiple access (NOMA) is an efficient solution that allows a non-orthogonal frequency resource allocation and handles many mobile users to a single channel. However, in wireless communication environments where a large number of mobile users exist and a huge volume of information is distributed simultaneously. In this situation, the energy and bandwidth allocation of the communication network is higher. This directs to reduce the life span of the entire network. In addition, wireless security for each transmission is very important during wireless transmissions. Based on motivation, MRRWPBA-NOMA called is developed.
System model: a system model of the MRRWPBA-NOMA is discussed. NOMA is a various many user access system with respect to other multiple access schemes, i.e., orthogonal multiple access. The basic idea of non-orthogonal access is to employ a receiver with a complex design in transmission for high spectral efficiency.
Fig. 1 shows the architecture of NOMA in Mobile Networks communication in which consists of one Macro Base Station (MBS) and multiple users share the common resources. The base stations are implemented by the non-orthogonal multiple access technology. As shown in Fig. 1, wireless-enabled NOMA network with a base station (MBS), and a set of mobile users or devices
Figure 1: NOMA in mobile networks communication
In the downlink transmission, the total transmit the energy of the users is formulated as given below,
From Eq. (1),
From Eq. (2),
From Eq. (3), ‘
From Eq. (4),
Bootstrap aggregation is a machine learning ensemble technique that helps to increase the accurateness of classification as well as regression. The ensemble technique uses weak hypotheses for learning the given input. The weak hypothesis is a base classifier that difficult to provide precise results. Therefore, the ensemble technique combines the weak hypotheses and applies the voting scheme for obtaining the final accurate prediction results. The schematic representation of the bootstrap ensemble aggregation is shown in Fig. 2.
Figure 2: Schematic representation of multivariate renkonen index regressive weighted preference bootstrap aggregation
Fig. 2 portrays an overall schematic representation of a Multivariate Renkonen index Regressive Weighted preference bootstrap aggregation with several weak hypotheses. The ensemble technique considers the training input
A Multivariate Renkonen index Regression tree is a machine learning technique that helps to analyze the resource such as energy and bandwidth and characteristics is the trust of the mobile devices for efficient and secure data communication from the base station. The Regression tree includes the root node, branch node, and leaf node. In the root node, the mobile device resource and characteristics are analyzed with the threshold is verified. Each branch node symbolizes the outcome of the assessment, and each leaf node indicates a class label (after verifying the threshold). Here the Multivariate represents the energy, bandwidth, and trust. The Renkonen index is used to measure the similarity between the devices and their characteristics.
From Eq. (5),
The weak hypothesis results have some training errors in the classification process. In order to make strong output, weak hypothesis results are combined into strong.
From Eq. (6), ‘
For each weak hypothesis, the error is measured based on the squared difference between the actual classification results and predicted classification results.
From Eq. (7),
From Eq. (8),
Algorithm 1 is described step by step process of resource-efficient and secure communication in NOMA-based communication. First, the initialization of the Number of mobile users or devices, data, initial energy is set. Then the Bootstrap Aggregation is applied for identifying the resource-efficient and trusted mobile devices. The ensemble technique first constructs the set of weak hypotheses to analyze the energy, bandwidth, and trust of the mobile devices. Based on the analysis, the resource and trusted mobile devices are identified and making strong output by estimating error. Then the weighted preferential voting scheme is applied to find hypotheses with higher weight. Finally, the majority votes of the hypotheses results are considered as outcomes. Hence, resource-efficient and trusted devices are selected to improve data communication and reduce the latency.
In this section, the effectiveness of the proposed MRRWPBA-NOMA and conventional methods are verified using the NS2.34 simulator. Tab. 2 shows the simulation parameters.
Simulation Results Analysis
Simulation of the MRRWPBA-NOMA and other two conventional methods SP-J-SA-TS-PA (NOMA) [1] and CD/PD-NOMA [2] are discussed in this section. The performance of various methods is discussed with the help of different parameters such as data delivery ratio, throughput, latency, packet loss rate, and energy efficiency.
Data delivery ratio: This is a significant performance metric that is measured based on data packets successfully received from base station to mobile device. This parameter is also used to estimate the confidential data communication from the base station to the mobile device. The overall delivery ratio is measured in percentage (%) as shown in Tab. 2. The ratio is mathematically formulated as given below,
Latency: The latency of a network is estimated as the amount of time it takes for a data packet to be transferred from its base station to the mobile device. The latency is estimated as given below,
Where,
Throughput: It is measured as the size of data received from one base station to mobile devices in a specified amount of time. It is measured in terms of bits per second (bps). The throughput is estimated as given below,
Packet loss rate: It is measured as the ratio of the number of data packets lost to the total number of data packets sent. The packet loss rate is measured in percentage (%). The loss rate is estimated as follows,
Energy efficiency: It is defined as the ratio of the output energy and input energy to perform communication. The energy efficiency is calculated using the below mathematical formula,
From (14),
Tab. 3 and Fig. 3 illustrate the relationship between the numbers of mobile devices versus data delivery ratio using three different schemes namely MRRWPBA-NOMA, SP-J-SA-TS-PA (NOMA) [1], and CD/PD-NOMA [2] are implemented. In the simulations, three different schemes are compared, namely our proposed scheme, and traditional scheme. The numbers of mobile devices or users are 100, 200,...700, respectively. As shown in Fig. 3, the simulation results of the three methods are repressed by three different colors namely red, orange and green respectively. The results demonstrate that the proposed MRRWPBA-NOMA algorithm is better than other algorithms when the same number of mobile devices is considered. The main reason is that the MRRWPBA-NOMA finds resource-efficient devices and higher trusted devices. The higher trusted devices are used to perform the communication to protect the data from unauthorized devices. This process enhances the higher data delivery from the base station to the mobile device in the wireless network.
Figure 3: Performance of data delivery ratio vs. numbers of mobile devices
Tab. 4 and Fig. 4 illustrate the performance of latency versus the number of data packets taken in the ranges from 50, 100, 150, 200, 250, and 300. The graphical chart specifies that the latency of the three methods is represented by three different colors. Fig. 4 provides the seven iterations for all the methods. Among the three methods, the technique outperforms well in terms of achieving minimum latency. It is also evident that the NOMA method achieves minimum latency. In the first iteration, 50 data packets are considered to perform the simulation. Apply MRRWPBA-NOMA technique the latency of
Figure 4: Performance of latency vs. the number of data packets
In Tab. 5 and Fig. 5, the average number of iterations for three methods MRRWPBA-NOMA, [1,2] is illustrated. Each algorithm requires about 7 iterations on average to obtain the final results. In the above-given graphical plot, we have taken the throughput (bps) on the Y-axis versus the number of mobile devices ranging on the X-axis from 100 to 700 with 100 increment units. As can be understood, when the number of mobile devices increases and results in increased throughput. However, comparative analysis proves higher network throughput using the MRRWPBA-NOMA when compared to SP-J-SA-TS-PA (NOMA) [1] and CD/PD-NOMA [2]. In the first iteration, 100 mobile devices are considered to perform the simulation with 510 KB of data being downloaded from the base station. With the application of the MRRWPBA-NOMA technique, the mobile device 185 KB of the data is received from the base station. Whereas, the 170 KB and 160 KB sizes of the data are received by applying the conventional methods SP-J-SA-TS-PA (NOMA) [1] and CD/PD-NOMA [2]. For each method, seven various outcomes are observed with respect to a different number of mobile devices. Finally, the average of seven results of the proposed MRRWPBA-NOMA technique is compared to conventional methods. This is due to the application of higher bandwidth-efficient devices are selected by applying the Weighted Preference Bootstrap Aggregation. The ensemble technique uses the Multivariate Renkonen Regression function. The regression function analyzes the device characteristics and resources. The higher trust nodes reduce the packet loss and speed up the data transmission from the base station to the mobile device.
Figure 5: Performance of throughput vs. the number of mobile devices
Tab. 6 and Fig. 6 demonstrate the performance discussion between the numbers of mobile devices and packet loss rate using MRRWPBA-NOMA and two conventional schemes namely SP-J-SA-TS-PA (NOMA) [1], and CD/PD-NOMA [2]. In order to calculate the packet loss rate, the numbers of mobile devices or users are taken in the ranges from 100, 200, 700, respectively. The obtained evaluation results indicate that the MRRWPBA-NOMA provides better performance of minimizes the packet loss rate than the conventional NOMA schemes. This is due to the determination of the best device with higher trust. The higher trusted device minimizes the packet loss and increases the data transmission from the base station to the mobile device in the wireless network.
Figure 6: Performance of packet loss rate vs. the number of mobile devices
Tab. 7 and Fig. 7 illustrate the performance results of energy efficiency of the mobile devices taken in the ranges from 100 to 700. As shown in the observed results, the energy efficiency is decreased while increasing the number of mobile devices. This is due to the number of nodes consumed energy gets increased. Compared to all the three NOMA methods, the proposed MRRWPBA-NOMA outperforms well in terms of achieving higher energy efficiency. The mobile devices with higher residual energy are selected for efficient communication. From the obtained tabulated results and a graphical representation, MRRWPBA-NOMA provides superior performance of energy efficiency than the other methods.
Figure 7: Performance of energy efficiency vs. the number of mobile devices
Currently, non-orthogonal responsiveness has been considered valuable in modern developments in the mobile network multiple access systems. In this work, we have combined machine learning and NOMA which are well-known techniques in wireless networks to achieve resource-aware and secure communication. This contribution is achieved by developing the MRRWPBA-NOMA technique. In the downlink transmission of the NOMA technology, the mobile device energy, bandwidth and trust are estimated. Then the ensemble Bootstrap Aggregation technique is employed to classify the resource-efficient mobile devices for well-organized data communication by considering the weak hypothesis. The regression analyzes the resources of the mobile device with the threshold. Finally, the majority votes of the mobile devices are identified. With resource-aware and trusted devices, the transmission is carried out to improve the data delivery and reduces latency. Simulation of three methods is carried out to show the performance of different NOMA schemes. The simulation results show that the proposed algorithm is more effective than the traditional NOMA in terms of higher throughput, data delivery, and minimum latency in a dynamic mobile communication environment.
Acknowledgement: The authors are grateful to the Taif University Researchers Supporting Project number (TURSP-2020/36), Taif University, Taif, Saudi Arabia and This research was funded by Princess Nourah bint Abdulrahman University Researchers Supporting Project Number (PNURSP2022R97), Princess Nourah bint Abdulrahman University, Riyadh, Saudi Arabia.
Funding Statement: The authors are grateful to the Taif University Researchers Supporting Project number (TURSP-2020/36), Taif University, Taif, Saudi Arabia.
Conflicts of Interest: The authors declare that they have no conflicts of interest to report regarding the present study.
References
1. M. W. Baidas, E. Alsusa and Y. Shi, “Resource allocation for SWIPT-enabled energy-harvesting downlink/uplink clustered NOMA networks,” Computer Networks, vol. 182, no. 2, pp. 1–14, 2020. [Google Scholar]
2. X. Yue, Y. Liu, Y. Yao, X. Li, R. Liu et al., “Secure communications in a unified non-orthogonal multiple access framework,” IEEE Transactions on Wireless Communications, vol. 19, no. 3, pp. 2163–2178, 2020. [Google Scholar]
3. M. Hussain and H. Rasheed, “Nonorthogonal multiple access for next-generation mobile networks: A technical aspect for research direction,” Wireless Communications and Mobile Computing, vol. 2020, no. 5, pp. 1–17, 2020. [Google Scholar]
4. C. Li, J. Tang, Y. Zhang, X. Yan and Y. Luo, “Energy-efficient computation offloading for nonorthogonal multiple access assisted mobile edge computing with energy harvesting devices,” Computer Networks, vol. 164, no. 3, pp. 1–12, 2019. [Google Scholar]
5. Z. Liu, G. Hou, Y. Yuan, K. Y. Chan, K. Ma et al., “Robust resource allocation in two-tier NOMA heterogeneous networks toward 5G,” Computer Networks, vol. 176, no. 5, pp. 1–11, 2020. [Google Scholar]
6. A. Salem, L. Musavian, E. A. Jorswieck and S. Aïssa, “Secrecy outage probability of energy-harvesting cooperative noma transmissions with relay selection,” IEEE Transactions on Green Communications and Networking, vol. 4, no. 4, pp. 1130–1148, 2020. [Google Scholar]
7. L. Zhang, G. Zhang, X. Zhao, Y. Li, C. Huang et al., “Resource allocation for energy efficient user association in user-centric ultra-dense networks integrating NOMA and beamforming,” AEU International Journal of Electronics and Communications, vol. 124, no. 4, pp. 1–38, 2020. [Google Scholar]
8. A. Arafa, W. Shin, M. Vaezi and H. V. Poor, “Secure relaying in non-orthogonal multiple access: Trusted and untrusted scenarios,” IEEE Transactions on Information Forensics and Security, vol. 15, pp. 210–222, 2020. [Google Scholar]
9. W. Shin, H. Yang, M. Vaezi, J. Lee and H. V. Poor, “Relay-aided noma in uplink cellular networks,” IEEE Signal Processing Letters, vol. 24, no. 12, pp. 1842–1846, 2017. [Google Scholar]
10. K. N. Doan, M. Vaezi, W. Shin, H. V. Poor, H. Shin et al., “Power allocation in cache-aided noma systems: Optimization and deep reinforcement learning approaches,” IEEE Transactions on Communications, vol. 2019, pp. 1–30, 2019. [Google Scholar]
11. T. Nguyen, D. Do, C. X. Mavromoustakis and G. Mastorakis, “Opportunistic user selection schemes for energy harvesting-aware cooperative NOMA,” Physical Communication, vol. 44, pp. 1–21, 2019. [Google Scholar]
12. F. Li, H. Jiang, R. Fan and P. Tan, “Cognitive non-orthogonal multiple access with energy harvesting: An optimal resource allocation approach,” IEEE Transactions on Vehicular Technology, vol. 68, no. 7, pp. 7080–7095, 2019. [Google Scholar]
13. M. Liaqat, K. A. Noordin, T. A. Latefand and K. Dimyati, “Power-domain non orthogonal multiple access (PD-NOMA) in cooperative networks: An overview,” Wireless Networks, vol. 26, no. 1, pp. 181–203, 2020. [Google Scholar]
14. D. Hwang, J. Yang, S. S. Namand and H. Song, “Optimal multi-antenna transmission for the cooperative non-orthogonal multiple-access system,” Applied Science, vol. 11, no. 5, pp. 1–11, 2021. [Google Scholar]
15. J. Zhou, Y. Sun, Q. Cao, S. Li, Z. Sun et al., “Power Minimization for secure multi-user miso noma system with energy harvesting,” IEEE Transactions on Vehicular Technology, vol. 69, no. 9, pp. 10046–10058, 2020. [Google Scholar]
16. H. Yua and I. Lee, “Physical layer security based on NOMA and AJ for MISOSE channels with an untrusted relay,” Future Generation Computer Systems, vol. 102, no. 8, pp. 611–618, 2020. [Google Scholar]
17. W. Jiang, K. Huang, S. Xiao and X. Xu, “Secure transmission for a heterogeneous cellular network with limited feedback,” Science China Information Sciences, vol. 63, no. 12, pp. 1–14, 2020. [Google Scholar]
18. Z. Elsaraf, A. Ahmed, F. A. Khanand and Q. Z. Ahmed, “Cooperative non-orthogonal multiple access for wireless communication networks by exploiting the exit chart analysis,” EURASIP Journal on Wireless Communications and Networking, vol. 2021, no. 1, pp. 1–14, 2021. [Google Scholar]
19. M. W. Baidas, “Distributed energy-efficiency maximization in energy-harvesting uplink NOMA relay ad-hoc networks: Game-theoretic modeling and analysis,” Physical Communication, vol. 43, no. 10, pp. 1–14, 2020. [Google Scholar]
20. I. Azam, M. B. Shahab and S. Y. Shin, “Role switching and power allocation technique for mobile users in non-orthogonal multiple access,” Physical Communication, vol. 43, no. 2, pp. 1–9, 2020. [Google Scholar]
21. H. Xiaoli, S. Yu, X. Yu, M. Owais, W. Yang et al., “Resource allocation for throughput maximization in cognitive radio network with NOMA,” Computers, Materials & Continua, vol. 67, no. 3, pp. 3283–3292, 2021. [Google Scholar]
22. M. Abd-Elnaby, “Capacity and fairness maximization-based resource allocation for downlink NOMA networks,” Computers, Materials & Continua, vol. 69, no. 1, pp. 521–537, 2021. [Google Scholar]
Cite This Article
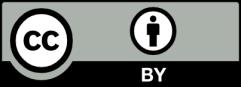
This work is licensed under a Creative Commons Attribution 4.0 International License , which permits unrestricted use, distribution, and reproduction in any medium, provided the original work is properly cited.