Open Access
ARTICLE
GRU-based Buzzer Ensemble for Abnormal Detection in Industrial Control Systems
1 Interdisciplinary Program of Information Security, Chonnam National University, Gwangju, 61186, Korea
2 Major in Computer Engineering, Chonnam National University, Yeosu, 59626, Korea
3 School of Business Administration, Chonnam National University, Gwangju, 61186, Korea
4 Department of Electronic Commerce, Chonnam National University, Yeosu, 59626, Korea
* Corresponding Author: Yong-Min Kim. Email:
Computers, Materials & Continua 2023, 74(1), 1749-1763. https://doi.org/10.32604/cmc.2023.026708
Received 02 January 2022; Accepted 23 February 2022; Issue published 22 September 2022
Abstract
Recently, Industrial Control Systems (ICSs) have been changing from a closed environment to an open environment because of the expansion of digital transformation, smart factories, and Industrial Internet of Things (IIoT). Since security accidents that occur in ICSs can cause national confusion and human casualties, research on detecting abnormalities by using normal operation data learning is being actively conducted. The single technique proposed by existing studies does not detect abnormalities well or provide satisfactory results. In this paper, we propose a GRU-based Buzzer Ensemble for Abnormal Detection (GBE-AD) model for detecting anomalies in industrial control systems to ensure rapid response and process availability. The newly proposed ensemble model of the buzzer method resolves False Negatives (FNs) by complementing the limited range that can be detected in a single model because of the internal models composing GBE-AD. Because the internal models remain suppressed for False Positives (FPs), GBE-AD provides better generalization. In addition, we generated mean prediction error data in GBE-AD and inferred abnormal processes using soft and hard clustering. We confirmed that the detection model's Time-series Aware Precision (TaP) suppressed FPs at 97.67%. The final performance was 94.04% in an experiment using an HIL-based Augmented ICS (HAI) Security Dataset (ver.21.03) among public datasets.Keywords
Cite This Article
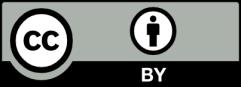
This work is licensed under a Creative Commons Attribution 4.0 International License , which permits unrestricted use, distribution, and reproduction in any medium, provided the original work is properly cited.