Open Access
ARTICLE
Optimized Weighted Ensemble Using Dipper Throated Optimization Algorithm in Metamaterial Antenna
1 Department of Computer Sciences, College of Computer and Information Sciences, Princess Nourah bint Abdulrahman University, P.O. Box 84428, Riyadh, 11671, Saudi Arabia
2 Department of Communications and Electronics, Delta Higher Institute of Engineering and Technology, Mansoura, 35111, Egypt
3 Faculty of Artificial Intelligence, Delta University for Science and Technology, Mansoura, 35712, Egypt
4 General Information Technology Department, Ministry of Defense, The Executive Affairs, Excellence Services Directorate,
Riyadh, 11564, Saudi Arabia
5 Computer Engineering and Control Systems Department, Faculty of Engineering, Mansoura University, Mansoura, 35516, Egypt
6 Department of Computer Science, Faculty of Computer and Information Sciences, Ain Shams University, Cairo, 11566, Egypt
7 Department of Computer Science, College of Computing and Information Technology, Shaqra University, 11961, Saudi Arabia
* Corresponding Author: Faten Khalid Karim. Email:
Computers, Materials & Continua 2022, 73(3), 5771-5788. https://doi.org/10.32604/cmc.2022.032229
Received 11 May 2022; Accepted 12 June 2022; Issue published 28 July 2022
Abstract
Metamaterial Antennas are a type of antenna that uses metamaterial to enhance performance. The bandwidth restriction associated with small antennas can be solved using metamaterial antennas. Machine learning is gaining popularity as a way to improve solutions in a range of fields. Machine learning approaches are currently a big part of current research, and they’re likely to be huge in the future. The model utilized determines the accuracy of the prediction in large part. The goal of this paper is to develop an optimized ensemble model for forecasting the metamaterial antenna’s bandwidth and gain. The basic models employed in the developed ensemble are Support Vector Regression (SVR), K-Nearest Regression (KNR), Multi-Layer Perceptron (MLP), Decision Trees (DT), and Random Forest (RF). The percentages of contribution of these models in the ensemble model are weighted and optimized using the dipper throated optimization (DTO) algorithm. To choose the best features from the dataset, the binary (bDTO) algorithm is exploited. The proposed ensemble model is compared to the base models and results are recorded and analyzed statistically. In addition, two other ensembles are incorporated in the conducted experiments for comparison. These ensembles are average ensemble and K-nearest neighbors (KNN)-based ensemble. The comparison is performed in terms of eleven evaluation criteria. The evaluation results confirmed the superiority of the proposed model when compared with the basic models and the other ensemble models.Keywords
Cite This Article
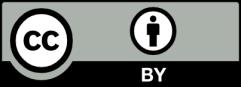