Open Access
ARTICLE
Feature Selection with Stacked Autoencoder Based Intrusion Detection in Drones Environment
1 Department of Electrical Engineering, College of Engineering, Princess Nourah Bint Abdulrahman University, P.O. Box 84428, Riyadh, 11671, Saudi Arabia
2 Department of Information Systems, College of Computing and Information System, Umm Al-Qura University, Saudi Arabia
3 Department of Information Systems, College of Science & Art at Mahayil, King Khalid University, Saudi Arabia
4 Department of Computer Science, Faculty of Computers and Information Technology, Future University in Egypt, New Cairo, 11835, Egypt
5 Department of Computer and Self Development, Preparatory Year Deanship, Prince Sattam Bin Abdulaziz University, AlKharj, Saudi Arabia
* Corresponding Author: Manar Ahmed Hamza. Email:
Computers, Materials & Continua 2022, 73(3), 5441-5458. https://doi.org/10.32604/cmc.2022.031887
Received 29 April 2022; Accepted 02 June 2022; Issue published 28 July 2022
Abstract
The Internet of Drones (IoD) offers synchronized access to organized airspace for Unmanned Aerial Vehicles (known as drones). The availability of inexpensive sensors, processors, and wireless communication makes it possible in real time applications. As several applications comprise IoD in real time environment, significant interest has been received by research communications. Since IoD operates in wireless environment, it is needed to design effective intrusion detection system (IDS) to resolve security issues in the IoD environment. This article introduces a metaheuristics feature selection with optimal stacked autoencoder based intrusion detection (MFSOSAE-ID) in the IoD environment. The major intention of the MFSOSAE-ID technique is to identify the occurrence of intrusions in the IoD environment. To do so, the proposed MFSOSAE-ID technique firstly pre-processes the input data into a compatible format. In addition, the presented MFSOSAE-ID technique designs a moth flame optimization based feature selection (MFOFS) technique to elect appropriate features. Moreover, firefly algorithm (FFA) with stacked autoencoder (SAE) model is employed for the recognition and classification of intrusions in which the SAE parameters are optimally tuned with utilize of FFA. The performance validation of the MFSOSAE-ID model was tested utilizing benchmark dataset and the outcomes implied the promising performance of the MFSOSAE-ID model over other techniques with maximum accuracy of 99.72%.Keywords
Cite This Article
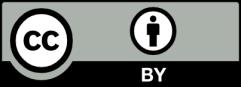
This work is licensed under a Creative Commons Attribution 4.0 International License , which permits unrestricted use, distribution, and reproduction in any medium, provided the original work is properly cited.