Open Access
ARTICLE
An Effective Classifier Model for Imbalanced Network Attack Data
Department of Information System Engineering, Mugla Sıtkı Koçman University, Mugla, 48000, Turkey
* Corresponding Author: Gürcan Çetin. Email:
Computers, Materials & Continua 2022, 73(3), 4519-4539. https://doi.org/10.32604/cmc.2022.031734
Received 25 April 2022; Accepted 07 June 2022; Issue published 28 July 2022
Abstract
Recently, machine learning algorithms have been used in the detection and classification of network attacks. The performance of the algorithms has been evaluated by using benchmark network intrusion datasets such as DARPA98, KDD’99, NSL-KDD, UNSW-NB15, and Caida DDoS. However, these datasets have two major challenges: imbalanced data and high-dimensional data. Obtaining high accuracy for all attack types in the dataset allows for high accuracy in imbalanced datasets. On the other hand, having a large number of features increases the runtime load on the algorithms. A novel model is proposed in this paper to overcome these two concerns. The number of features in the model, which has been tested at CICIDS2017, is initially optimized by using genetic algorithms. This optimum feature set has been used to classify network attacks with six well-known classifiers according to high f1-score and g-mean value in minimum time. Afterwards, a multi-layer perceptron based ensemble learning approach has been applied to improve the models’ overall performance. The experimental results show that the suggested model is acceptable for feature selection as well as classifying network attacks in an imbalanced dataset, with a high f1-score (0.91) and g-mean (0.99) value. Furthermore, it has outperformed base classifier models and voting procedures.Keywords
Cite This Article
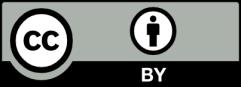
This work is licensed under a Creative Commons Attribution 4.0 International License , which permits unrestricted use, distribution, and reproduction in any medium, provided the original work is properly cited.