Open Access
ARTICLE
Seeker Optimization with Deep Learning Enabled Sentiment Analysis on Social Media
1 Department of Computer Science, College of Computing Al-Qunfidhah, Umm Al-Qura University, Saudi Arabia
2 Department of Computer Science, College of Science and Humanities, Slayel, Prince Sattam Bin AbdulAziz University, Saudi Arabia
3 Department of Computer Science, College of Computer Science and Information Systems, Najran Univesity, Najran, 61441, Saudi Arabia
4 Department of Mathematics, College of Science, Taif University, P.O. Box 11099, Taif, 21944, Saudi Arabia
5 Department of Mathematics, Faculty of Science, Sohag University, Sohag, Egypt
* Corresponding Author: Sayed Abdel-Khalek. Email:
Computers, Materials & Continua 2022, 73(3), 5985-5999. https://doi.org/10.32604/cmc.2022.031732
Received 25 April 2022; Accepted 09 June 2022; Issue published 28 July 2022
Abstract
World Wide Web enables its users to connect among themselves through social networks, forums, review sites, and blogs and these interactions produce huge volumes of data in various forms such as emotions, sentiments, views, etc. Sentiment Analysis (SA) is a text organization approach that is applied to categorize the sentiments under distinct classes such as positive, negative, and neutral. However, Sentiment Analysis is challenging to perform due to inadequate volume of labeled data in the domain of Natural Language Processing (NLP). Social networks produce interconnected and huge data which brings complexity in terms of expanding SA to an extensive array of applications. So, there is a need exists to develop a proper technique for both identification and classification of sentiments in social media. To get rid of these problems, Deep Learning methods and sentiment analysis are consolidated since the former is highly efficient owing to its automatic learning capability. The current study introduces a Seeker Optimization Algorithm with Deep Learning enabled SA and Classification (SOADL-SAC) for social media. The presented SOADL-SAC model involves the proper identification and classification of sentiments in social media. In order to attain this, SOADL-SAC model carries out data preprocessing to clean the input data. In addition, Glove technique is applied to generate the feature vectors. Moreover, Self-Head Multi-Attention based Gated Recurrent Unit (SHMA-GRU) model is exploited to recognize and classify the sentiments. Finally, Seeker Optimization Algorithm (SOA) is applied to fine-tune the hyperparameters involved in SHMA-GRU model which in turn enhances the classifier results. In order to validate the enhanced outcomes of the proposed SOADL-SAC model, various experiments were conducted on benchmark datasets. The experimental results inferred the better performance of SOADL-SAC model over recent state-of-the-art approaches.Keywords
Cite This Article
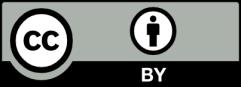