Open Access
ARTICLE
Motion Enhanced Model Based on High-Level Spatial Features
1 College of Computer Science and Engineering, Inner Mongolia University, Hohhot, 010021, China
2 Department of Information and Communications, PaiChai University, Daejeon, 35345, Korea
* Corresponding Author: Ming Ma. Email:
Computers, Materials & Continua 2022, 73(3), 5911-5924. https://doi.org/10.32604/cmc.2022.031664
Received 23 April 2022; Accepted 29 May 2022; Issue published 28 July 2022
Abstract
Action recognition has become a current research hotspot in computer vision. Compared to other deep learning methods, Two-stream convolutional network structure achieves better performance in action recognition, which divides the network into spatial and temporal streams, using video frame images as well as dense optical streams in the network, respectively, to obtain the category labels. However, the two-stream network has some drawbacks, i.e., using dense optical flow as the input of the temporal stream, which is computationally expensive and extremely time-consuming for the current extraction algorithm and cannot meet the requirements of real-time tasks. In this paper, instead of the dense optical flow, the Motion Vectors (MVs) are used and extracted from the compressed domain as temporal features, which greatly reduces the extraction time. However, the motion pattern that MVs contain is coarser, which leads to low accuracy. In this paper, we propose two strategies to improve the accuracy: firstly, an accumulated strategy is used to enhance the motion information and continuity of MVs; secondly, knowledge distillation is used to fuse the spatial information into the temporal stream so that more information (e.g., motion details, colors, etc.) is obtainable. Experimental results show that the accuracy of MV can be greatly improved by the strategies proposed in this paper and the final recognition for human actions accuracy is guaranteed without using optical flow.Keywords
Cite This Article
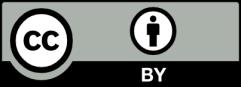
This work is licensed under a Creative Commons Attribution 4.0 International License , which permits unrestricted use, distribution, and reproduction in any medium, provided the original work is properly cited.