Open Access
ARTICLE
Water Wave Optimization with Deep Learning Driven Smart Grid Stability Prediction
1 Department of Electrical and Computer Engineering, International Islamic University Malaysia, Kuala Lumpur, 53100, Malaysia
2 Department of Computer and Self Development, Preparatory Year Deanship, Prince Sattam Bin Abdulaziz University, AlKharj, Saudi Arabia
3 Department of Electrical Engineering, College of Engineering, Princess Nourah Bint Abdulrahman University, P.O. Box 84428, Riyadh, 11671, Saudi Arabia
4 Department of Information Systems, College of Science & Art at Mahayil, King Khalid University, Saudi Arabia
5 Department of Computer Science and Bioinformatics, Singhania University, Pacheri Bari, Jhnujhunu, Rajasthan, India
6 Department of Computer Sciences, College of Computing and Information System, Umm Al-Qura University, Saudi Arabia
7 Department of Mechanical Engineering, Faculty of Engineering & Technology, Future University in Egypt, New Cairo, 11835, Egypt
* Corresponding Author: Anwer Mustafa Hilal. Email:
Computers, Materials & Continua 2022, 73(3), 6019-6035. https://doi.org/10.32604/cmc.2022.031425
Received 17 April 2022; Accepted 29 May 2022; Issue published 28 July 2022
Abstract
Smart Grid (SG) technologies enable the acquisition of huge volumes of high dimension and multi-class data related to electric power grid operations through the integration of advanced metering infrastructures, control systems, and communication technologies. In SGs, user demand data is gathered and examined over the present supply criteria whereas the expenses are then informed to the clients so that they can decide about electricity consumption. Since the entire procedure is valued on the basis of time, it is essential to perform adaptive estimation of the SG’s stability. Recent advancements in Machine Learning (ML) and Deep Learning (DL) models enable the designing of effective stability prediction models in SGs. In this background, the current study introduces a novel Water Wave Optimization with Optimal Deep Learning Driven Smart Grid Stability Prediction (WWOODL-SGSP) model. The aim of the presented WWOODL-SGSP model is to predict the stability level of SGs in a proficient manner. To attain this, the proposed WWOODL-SGSP model initially applies normalization process to scale the data to a uniform level. Then, WWO algorithm is applied to choose an optimal subset of features from the pre-processed data. Next, Deep Belief Network (DBN) model is followed to predict the stability level of SGs. Finally, Slime Mold Algorithm (SMA) is exploited to fine tune the hyperparameters involved in DBN model. In order to validate the enhanced performance of the proposed WWOODL-SGSP model, a wide range of experimental analyses was performed. The simulation results confirm the enhanced predictive results of WWOODL-SGSP model over other recent approaches.Keywords
Cite This Article
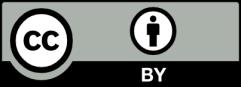
This work is licensed under a Creative Commons Attribution 4.0 International License , which permits unrestricted use, distribution, and reproduction in any medium, provided the original work is properly cited.