Open Access
ARTICLE
Hyperparameter Tuned Deep Learning Enabled Cyberbullying Classification in Social Media
1 Department of Computer Science, College of Sciences and Humanities-Aflaj, Prince Sattam bin Abdulaziz University, Saudi Arabia
2 Department of Electrical Engineering, College of Engineering, Princess Nourah Bint Abdulrahman University, P.O. Box 84428, Riyadh, 11671, Saudi Arabia
3 Department of Information Systems, College of Computing and Information System, Umm Al-Qura University, Saudi Arabia
4 Department of Computer Science, College of Science & Art at Mahayil, King Khalid University, Muhayel Aseer, Saudi Arabia
5 Faculty of Computers and Information, Computer Science Department, Menoufia University, Egypt
6 Research Centre, Future University in Egypt, New Cairo, 11845, Egypt
7 Department of Computer and Self Development, Preparatory Year Deanship, Prince Sattam bin Abdulaziz University, Al-Kharj, Saudi Arabia
8 Department of Information System, College of Computer Engineering and Sciences, Prince Sattam bin Abdulaziz University, Al Kharj, Saudi Arabia
* Corresponding Author: Mesfer Al Duhayyim. Email:
Computers, Materials & Continua 2022, 73(3), 5011-5024. https://doi.org/10.32604/cmc.2022.031096
Received 10 April 2022; Accepted 20 May 2022; Issue published 28 July 2022
Abstract
Cyberbullying (CB) is a challenging issue in social media and it becomes important to effectively identify the occurrence of CB. The recently developed deep learning (DL) models pave the way to design CB classifier models with maximum performance. At the same time, optimal hyperparameter tuning process plays a vital role to enhance overall results. This study introduces a Teacher Learning Genetic Optimization with Deep Learning Enabled Cyberbullying Classification (TLGODL-CBC) model in Social Media. The proposed TLGODL-CBC model intends to identify the existence and non-existence of CB in social media context. Initially, the input data is cleaned and pre-processed to make it compatible for further processing. Followed by, independent recurrent autoencoder (IRAE) model is utilized for the recognition and classification of CBs. Finally, the TLGO algorithm is used to optimally adjust the parameters related to the IRAE model and shows the novelty of the work. To assuring the improved outcomes of the TLGODL-CBC approach, a wide range of simulations are executed and the outcomes are investigated under several aspects. The simulation outcomes make sure the improvements of the TLGODL-CBC model over recent approaches.Keywords
Cite This Article
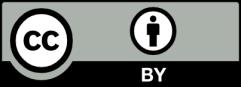
This work is licensed under a Creative Commons Attribution 4.0 International License , which permits unrestricted use, distribution, and reproduction in any medium, provided the original work is properly cited.