Open Access
ARTICLE
Optimal Logistics Activities Based Deep Learning Enabled Traffic Flow Prediction Model
1 Ports and Maritime Transportation Department, Faculty of Maritime Studies, King Abdulaziz University, Jeddah, 21589, Saudi Arabia
2 Information Technology Department, Faculty of Computing and Information Technology, King Abdulaziz University, Jeddah, 21589, Saudi Arabia
3 Department of Mathematics, Faculty of Science, Al-Azhar University, Naser City, 11884, Cairo, Egypt
4 Center of Excellence in Smart Environment Research, King Abdulaziz University, Jeddah, 21589, Saudi Arabia
5 Department of Computer Science, Faculty of Computing and Information Technology, King Abdulaziz University, Jeddah, 21589, Saudi Arabia
6 Information Systems Department, Faculty of Computing and Information Technology, King Abdulaziz University, Jeddah, 21589, Saudi Arabia
7 Information Systems Department, HECI School, Dar Alhekma University, Jeddah, Saudi Arabia
* Corresponding Author: Mahmoud Ragab. Email:
Computers, Materials & Continua 2022, 73(3), 5269-5282. https://doi.org/10.32604/cmc.2022.030694
Received 31 March 2022; Accepted 19 May 2022; Issue published 28 July 2022
Abstract
Traffic flow prediction becomes an essential process for intelligent transportation systems (ITS). Though traffic sensor devices are manually controllable, traffic flow data with distinct length, uneven sampling, and missing data finds challenging for effective exploitation. The traffic data has been considerably increased in recent times which cannot be handled by traditional mathematical models. The recent developments of statistic and deep learning (DL) models pave a way for the effectual design of traffic flow prediction (TFP) models. In this view, this study designs optimal attention-based deep learning with statistical analysis for TFP (OADLSA-TFP) model. The presented OADLSA-TFP model intends to effectually forecast the level of traffic in the environment. To attain this, the OADLSA-TFP model employs attention-based bidirectional long short-term memory (ABLSTM) model for predicting traffic flow. In order to enhance the performance of the ABLSTM model, the hyperparameter optimization process is performed using artificial fish swarm algorithm (AFSA). A wide-ranging experimental analysis is carried out on benchmark dataset and the obtained values reported the enhancements of the OADLSA-TFP model over the recent approaches mean square error (MSE), root mean square error (RMSE), and mean absolute percentage error (MAPE) of 120.342%, 10.970%, and 8.146% respectively.Keywords
Cite This Article
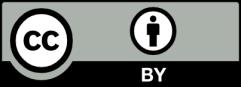