Open Access
ARTICLE
State-of-Charge Estimation of Lithium-Ion Battery for Electric Vehicles Using Deep Neural Network
1 Department of Electrical and Electronics Engineering, Dayananda Sagar College of Engineering, Bengaluru, 560078, Karnataka, India
2 Department of Electrical and Electronics Engineering, National Institute of Technology, Tiruchirapalli, 620015, Tamil Nadu, India
3 Department of Electrical and Electronics Engineering, M S Ramaiah Institute of Technology, Bengaluru, 560054, Karnataka, India
4 Department of Electrical and Electronics Engineering, M. Kumarasamy College of Engineering, Karur, 639113, Tamil Nadu, India
5 Electrical Engineering Department, College of Engineering, King Khalid University, Abha, 61421, Saudi Arabia
6 Computers and Communications Department, College of Engineering, Delta University for Science and Technology, Gamasa, 35712, Egypt
7 Faculty of Technology, The Gateway, De Montfort University, Leicester, LE1 9BH, United Kingdom
8 Department of Mathematics, College of Arts and Sciences, Prince Sattam bin Abdulaziz University, Wadi Aldawaser, 11991, Saudi Arabia
* Corresponding Author: Kottakkaran Sooppy Nisar. Email:
Computers, Materials & Continua 2022, 73(3), 6289-6306. https://doi.org/10.32604/cmc.2022.030490
Received 27 March 2022; Accepted 28 April 2022; Issue published 28 July 2022
Abstract
It is critical to have precise data about Lithium-ion batteries, such as the State-of-Charge (SoC), to maintain a safe and consistent functioning of battery packs in energy storage systems of electric vehicles. Numerous strategies for estimating battery SoC, such as by including the coulomb counting and Kalman filter, have been established. As a result of the differences in parameter values between each cell, when these methods are applied to high-capacity battery packs, it has difficulties sustaining the prediction accuracy of overall cells. As a result of aging, the variation in the parameters of each cell is higher as more time is spent in operation. It is suggested in this study to establish an SoC estimate model for a Lithium-ion battery by employing an enhanced Deep Neural Network (DNN) approach. This is because the proposed DNN has a substantial hidden layer, which can accurately predict the SoC of an unknown driving cycle during training, making it ideal for SoC estimation. To evaluate the nonlinearities between voltage and current at various SoCs and temperatures, the proposed DNN is applied. Using current and voltage data measured at various temperatures throughout discharge/charge cycles is necessary for training and testing purposes. When the method has been thoroughly trained with the data collected, it is used for additional cells cycle tests to predict their SoC. The simulation has been conducted for two different Li-ion battery datasets. According to the experimental data, the suggested DNN-based SoC estimate approach produces a low mean absolute error and root-mean-square-error values, say less than 5% errors.Keywords
Cite This Article
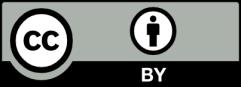
This work is licensed under a Creative Commons Attribution 4.0 International License , which permits unrestricted use, distribution, and reproduction in any medium, provided the original work is properly cited.