Open Access
ARTICLE
Residual Autoencoder Deep Neural Network for Electrical Capacitance Tomography
1 Department of Computer Science in Jamoum, Umm Al-Qura University, Makkah, 25371, Saudi Arabia
2 Computers and Systems Engineering Department, Mansoura University, Mansoura, 35516, Egypt
3 Laboratoire D’Informatique et des Technologies de L’Information D’Oran (LITIO), University of Oran, 31000, Oran, Algeria
* Corresponding Author: Wael Deabes. Email:
Computers, Materials & Continua 2022, 73(3), 6307-6326. https://doi.org/10.32604/cmc.2022.030420
Received 25 March 2022; Accepted 15 June 2022; Issue published 28 July 2022
Abstract
Great achievements have been made during the last decades in the field of Electrical Capacitance Tomography (ECT) image reconstruction. However, there is still a need to make these image reconstruction results faster and of better quality. Recently, Deep Learning (DL) is flourishing and is adopted in many fields. The DL is very good at dealing with complex nonlinear functions and it is built using several series of Artificial Neural Networks (ANNs). An ECT image reconstruction model using DNN is proposed in this paper. The proposed model mainly uses Residual Autoencoder called (ECT_ResAE). A large-scale dataset of 320 k instances have been generated to train and test the proposed ECT_ResAE model. Each instance contains two vectors; a distinct permittivity distribution and its corresponding capacitance measurements. The capacitance vector has been modulated to generate a 66×66 image, and represented to the ECT_ResAE as an input. The scalability and practicability of the ECT_ResAE network are tested using noisy data, new samples, and experimental data. The experimental results show that the proposed ECT_ResAE image reconstruction model provides accurate reconstructed images. It achieved an average image Correlation Coefficient (CC) of more than 99% and an average Relative Image Error (IE) around 8.5%.Keywords
Cite This Article
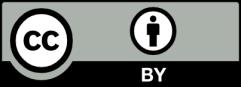