Open Access
ARTICLE
Chaotic Krill Herd with Deep Transfer Learning-Based Biometric Iris Recognition System
1 Department of Computer Science, Faculty of Information Technology, Al-Hussein Bin Talal University Ma’an, 71111, Jordan
2 MIS Department, College of Business Administration, University of Business and Technology, Jeddah, 21448, Saudi Arabia
* Corresponding Author: Bassam A. Y. Alqaralleh. Email:
Computers, Materials & Continua 2022, 73(3), 5703-5715. https://doi.org/10.32604/cmc.2022.030399
Received 25 March 2022; Accepted 07 June 2022; Issue published 28 July 2022
Abstract
Biometric verification has become essential to authenticate the individuals in public and private places. Among several biometrics, iris has peculiar features and its working mechanism is complex in nature. The recent developments in Machine Learning and Deep Learning approaches enable the development of effective iris recognition models. With this motivation, the current study introduces a novel Chaotic Krill Herd with Deep Transfer Learning Based Biometric Iris Recognition System (CKHDTL-BIRS). The presented CKHDTL-BIRS model intends to recognize and classify iris images as a part of biometric verification. To achieve this, CKHDTL-BIRS model initially performs Median Filtering (MF)-based preprocessing and segmentation for iris localization. In addition, MobileNet model is also utilized to generate a set of useful feature vectors. Moreover, Stacked Sparse Autoencoder (SSAE) approach is applied for classification. At last, CKH algorithm is exploited for optimization of the parameters involved in SSAE technique. The proposed CKHDTL-BIRS model was experimentally validated using benchmark dataset and the outcomes were examined under several aspects. The comparison study results established the enhanced performance of CKHDTL-BIRS technique over recent approaches.Keywords
Cite This Article
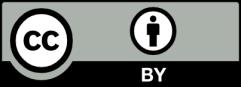
This work is licensed under a Creative Commons Attribution 4.0 International License , which permits unrestricted use, distribution, and reproduction in any medium, provided the original work is properly cited.