Open Access
ARTICLE
KGSR-GG: A Noval Scheme for Dynamic Recommendation
1 Xi’an Research Institute of High-Tech, Xi’an, Shaanxi, 710025, China
2 Nanyang Technological University, Singapore, 639798, Singapore
* Corresponding Author: Kai-Yuan Cheng. Email:
Computers, Materials & Continua 2022, 73(3), 5509-5524. https://doi.org/10.32604/cmc.2022.030150
Received 19 March 2022; Accepted 08 June 2022; Issue published 28 July 2022
Abstract
Recommendation algorithms regard user-item interaction as a sequence to capture the user’s short-term preferences, but conventional algorithms cannot capture information of constantly-changing user interest in complex contexts. In these years, combining the knowledge graph with sequential recommendation has gained momentum. The advantages of knowledge graph-based recommendation systems are that more semantic associations can improve the accuracy of recommendations, rich association facts can increase the diversity of recommendations, and complex relational paths can hence the interpretability of recommendations. But the information in the knowledge graph, such as entities and relations, often fails to be fully utilized and high-order connectivity is unattainable in graph modelling in knowledge graph-based sequential recommender systems. To address the above problems, a knowledge graph-based sequential recommendation algorithm that combines the gated recurrent unit and the graph neural network (KGSR-GG) is proposed in the present work. Specifically, entity disambiguation in the knowledge graph is performed on the preprocessing layer; on the embedding layer, the TransR embedding technique is employed to process the user information, item information and the entities and relations in the knowledge graph; on the aggregation layer, the information is aggregated by graph convolutional neural networks and residual connections; and at last, on the sequence layer, a bi-directional gated recurrent unit (Bi-GRU) is utilized to model the user’s sequential preferences. The research results showed that this new algorithm performed better than existing sequential recommendation algorithms on the MovieLens-1M and Book-Crossing datasets, as measured by five evaluation indicators.Keywords
Cite This Article
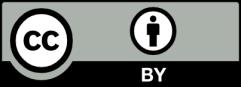
This work is licensed under a Creative Commons Attribution 4.0 International License , which permits unrestricted use, distribution, and reproduction in any medium, provided the original work is properly cited.