Open Access
ARTICLE
Integrated Evolving Spiking Neural Network and Feature Extraction Methods for Scoliosis Classification
1 Faculty of Engineering, Universiti Teknologi Malaysia, School of Computing, Skudai, 81310, Johor, Malaysia
2 Faculty of Computer and Mathematical Sciences, Universiti Teknologi MARA Cawangan Melaka Kampus Jasin, Merlimau, 77300, Melaka, Malaysia
3 Faculty of Computer and Mathematical Sciences, University Teknologi MARA, Shah Alam, 40450, Selangor, Malaysia
4 Fakulti Perubatan & Kesihatan Pertahanan, Universiti Pertahanan Nasional Malaysia (UPNM), Sungai Besi, 57000, Kuala Lumpur, Malaysia
* Corresponding Author: Nurbaity Sabri. Email:
Computers, Materials & Continua 2022, 73(3), 5559-5573. https://doi.org/10.32604/cmc.2022.029221
Received 28 February 2022; Accepted 20 May 2022; Issue published 28 July 2022
Abstract
Adolescent Idiopathic Scoliosis (AIS) is a deformity of the spine that affects teenagers. The current method for detecting AIS is based on radiographic images which may increase the risk of cancer growth due to radiation. Photogrammetry is another alternative used to identify AIS by distinguishing the curves of the spine from the surface of a human’s back. Currently, detecting the curve of the spine is manually performed, making it a time-consuming task. To overcome this issue, it is crucial to develop a better model that automatically detects the curve of the spine and classify the types of AIS. This research proposes a new integration of ESNN and Feature Extraction (FE) methods and explores the architecture of ESNN for the AIS classification model. This research identifies the optimal Feature Extraction (FE) methods to reduce computational complexity. The ability of ESNN to provide a fast result with a simplicity and performance capability makes this model suitable to be implemented in a clinical setting where a quick result is crucial. A comparison between the conventional classifier (Support Vector Machine (SVM), Multi-layer Perceptron (MLP) and Random Forest (RF)) with the proposed AIS model also be performed on a dataset collected by an orthopedic expert from Hospital Universiti Kebangsaan Malaysia (HUKM). This dataset consists of various photogrammetry images of the human back with different types of Malaysian AIS patients to solve the scoliosis problem. The process begins by pre-processing the images which includes resizing and converting the captured pictures to gray-scale images. This is then followed by feature extraction, normalization, and classification. The experimental results indicate that the integration of LBP and ESNN achieves higher accuracy compared to the performance of multiple baseline state-of-the-art Machine Learning for AIS classification. This demonstrates the capability of ESNN in classifying the types of AIS based on photogrammetry images.Keywords
Cite This Article
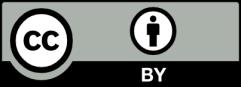