Open Access
ARTICLE
Emotion Recognition from Occluded Facial Images Using Deep Ensemble Model
1 Department of Computer Science, The Brains Institute, Peshawar, 25000, Pakistan
2 School of Natural and Computing Sciences, University of Aberdeen, Aberdeen, UK
3 Department of Neurosciences, KU Leuven Medical School, Leuven, 3000, Belgium
4 Security Engineering Laboratory, CCIS, Prince Sultan University, Riyadh, 12435, Saudi Arabia
5 Robotics and Internet of Things Lab, Prince Sultan University, Riyadh, 12435, Saudi Arabia
6 Department of Computer Science, Robert Gordon University, Aberdeen, UK
* Corresponding Author: Sadaqat ur Rehman. Email:
Computers, Materials & Continua 2022, 73(3), 4465-4487. https://doi.org/10.32604/cmc.2022.029101
Received 25 February 2022; Accepted 24 May 2022; Issue published 28 July 2022
Abstract
Facial expression recognition has been a hot topic for decades, but high intraclass variation makes it challenging. To overcome intraclass variation for visual recognition, we introduce a novel fusion methodology, in which the proposed model first extract features followed by feature fusion. Specifically, RestNet-50, VGG-19, and Inception-V3 is used to ensure feature learning followed by feature fusion. Finally, the three feature extraction models are utilized using Ensemble Learning techniques for final expression classification. The representation learnt by the proposed methodology is robust to occlusions and pose variations and offers promising accuracy. To evaluate the efficiency of the proposed model, we use two wild benchmark datasets Real-world Affective Faces Database (RAF-DB) and AffectNet for facial expression recognition. The proposed model classifies the emotions into seven different categories namely: happiness, anger, fear, disgust, sadness, surprise, and neutral. Furthermore, the performance of the proposed model is also compared with other algorithms focusing on the analysis of computational cost, convergence and accuracy based on a standard problem specific to classification applications.Keywords
Cite This Article
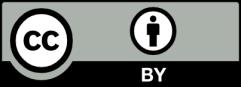