Open Access
ARTICLE
Apex Frame Spotting Using Attention Networks for Micro-Expression Recognition System
1 Department of Electrical, Electronic and Systems Engineering, Universiti Kebangsaan Malaysia, Bangi, 43600, Malaysia
2 Department of Electrical, Electronic and Systems Engineering, Universiti Kebangsaan Malaysia, Bangi, 43600, Malaysia
3 Faculty of Mathematics and Natural Science, Universitas Indonesia, Depok, 16424, Indonesia
4 Faculty of Humanities, Management and Science, Universiti Putra Malaysia Bintulu Campus, Bintulu, 97008, Malaysia
* Corresponding Author: Mohd Asyraf Zulkifley. Email:
Computers, Materials & Continua 2022, 73(3), 5331-5348. https://doi.org/10.32604/cmc.2022.028801
Received 11 February 2022; Accepted 18 May 2022; Issue published 28 July 2022
Abstract
Micro-expression is manifested through subtle and brief facial movements that relay the genuine person’s hidden emotion. In a sequence of videos, there is a frame that captures the maximum facial differences, which is called the apex frame. Therefore, apex frame spotting is a crucial sub-module in a micro-expression recognition system. However, this spotting task is very challenging due to the characteristics of micro-expression that occurs in a short duration with low-intensity muscle movements. Moreover, most of the existing automated works face difficulties in differentiating micro-expressions from other facial movements. Therefore, this paper presents a deep learning model with an attention mechanism to spot the micro-expression apex frame from optical flow images. The attention mechanism is embedded into the model so that more weights can be allocated to the regions that manifest the facial movements with higher intensity. The method proposed in this paper has been tested and verified on two spontaneous micro-expression databases, namely Spontaneous Micro-facial Movement (SAMM) andChinese Academy of Sciences Micro-expression (CASME) II databases. The proposed system performance is evaluated by using the Mean Absolute Error (MAE) metric that measures the distance between the predicted apex frame and the ground truth label. The best MAE of 14.90 was obtained when a combination of five convolutional layers, local response normalization, and attention mechanism is used to model the apex frame spotting. Even with limited datasets, the results have proven that the attention mechanism has better emphasized the regions where the facial movements likely to occur and hence, improves the spotting performance.Keywords
Cite This Article
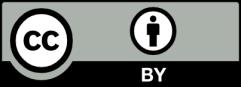