Open Access
ARTICLE
Trustworthy Explainable Recommendation Framework for Relevancy
Department of Computer Science, University of Engineering and Technology, Lahore, 54000, Pakistan
* Corresponding Author: Saba Sana. Email:
Computers, Materials & Continua 2022, 73(3), 5887-5909. https://doi.org/10.32604/cmc.2022.028046
Received 01 February 2022; Accepted 09 May 2022; Issue published 28 July 2022
Abstract
Explainable recommendation systems deal with the problem of ‘Why’. Besides providing the user with the recommendation, it is also explained why such an object is being recommended. It helps to improve trustworthiness, effectiveness, efficiency, persuasiveness, and user satisfaction towards the system. To recommend the relevant information with an explanation to the user is required. Existing systems provide the top-k recommendation options to the user based on ratings and reviews about the required object but unable to explain the matched-attribute-based recommendation to the user. A framework is proposed to fetch the most specific information that matches the user requirements based on Formal Concept Analysis (FCA). The ranking quality of the recommendation list for the proposed system is evaluated quantitatively with Normalized Discounted Cumulative Gain (NDCG)@k, which is better than the existing systems. Explanation is provided qualitatively by considering trustworthiness criterion i.e., among the seven explainability evaluation criteria, and its metric satisfies the results of proposed method. This framework can be enhanced to accommodate for more effectiveness and trustworthiness.Keywords
Cite This Article
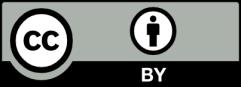