Open Access
ARTICLE
Sika Deer Behavior Recognition Based on Machine Vision
1 Jilin Agricultural University, Changchun, 130118, China
2 Wuzhou University, Wuzhou, 543000, China
3 Jilin Province Agricultural Internet of Things Technology Collaborative Innovation Center, Changchun, 130118, China
4 Jilin Province Intelligent Environmental Engineering Research Center, Changchun, 130118, China
5 Department of Agricultural Economics and Animal Production, University of Limpopo, Sovenga, 0727, Polokwane, South Africa
6 Guangxi Key Laboratory of Machine Vision and Intelligent Control, Wuzhou, 543000, China
* Corresponding Author: Shijun Li. Email:
Computers, Materials & Continua 2022, 73(3), 4953-4969. https://doi.org/10.32604/cmc.2022.027457
Received 19 January 2022; Accepted 09 May 2022; Issue published 28 July 2022
Abstract
With the increasing intensive and large-scale development of the sika deer breeding industry, it is crucial to assess the health status of the sika deer by monitoring their behaviours. A machine vision–based method for the behaviour recognition of sika deer is proposed in this paper. Google Inception Net (GoogLeNet) is used to optimise the model in this paper. First, the number of layers and size of the model were reduced. Then, the 5 × 5 convolution was changed to two 3 × 3 convolutions, which reduced the parameters and increased the nonlinearity of the model. A 5 × 5 convolution kernel was used to replace the original convolution for extracting coarse-grained features and improving the model’s extraction ability. A multi-scale module was added to the model to enhance the multi-faceted feature extraction capability of the model. Simultaneously, the Squeeze-and-Excitation Networks (SE-Net) module was included to increase the channel’s attention and improve the model’s accuracy. The dataset’s images were rotated to reduce overfitting. For image rotation, the angle was multiplied by 30° to obtain the dataset enhanced by rotation operations of 30°, 60°, 90°, 120° and 150°. The experimental results showed that the recognition rate of this model in the behaviour of sika deer was 98.92%. Therefore, the model presented in this paper can be applied to the behaviour recognition of sika deer. The results will play an essential role in promoting animal behaviour recognition technology and animal health monitoring management.Keywords
Cite This Article
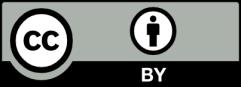