Open Access
ARTICLE
Environment Adaptive Deep Learning Classification System Based on One-shot Guidance
1 School of Telecommunication Engineering, Beijing Polytechnic, Beijing, 100176, China
2 Department of Mathematics, Wayne State University, Detroit, 48202, MI, USA
3 School of Computer Science and Technology, Tiangong University, Tianjin, 300387, China
* Corresponding Author: Jing Yu. Email:
Computers, Materials & Continua 2022, 73(3), 5185-5196. https://doi.org/10.32604/cmc.2022.027307
Received 14 January 2022; Accepted 14 March 2022; Issue published 28 July 2022
Abstract
When utilizing the deep learning models in some real applications, the distribution of the labels in the environment can be used to increase the accuracy. Generally, to compute this distribution, there should be the validation set that is labeled by the ground truths. On the other side, the dependency of ground truths limits the utilization of the distribution in various environments. In this paper, we carried out a novel system for the deep learning-based classification to solve this problem. Firstly, our system only uses one validation set with ground truths to compute some hyper parameters, which is named as one-shot guidance. Secondly, in an environment, our system builds the validation set and labels this by the prediction results, which does not need any guidance by the ground truths. Thirdly, the computed distribution of labels by the validation set selectively cooperates with the probability of labels by the output of models, which is to increase the accuracy of predict results on testing samples. We selected six popular deep learning models on three real datasets for the evaluation. The experimental results show that our system can achieve higher accuracy than state-of-art methods while reducing the dependency of labeled validation set.Keywords
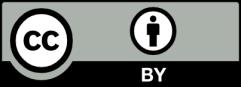