Open Access
ARTICLE
A Lightweight Model of VGG-U-Net for Remote Sensing Image Classification
1 College of Information Technology, Jilin Agricultural University, Changchun, 130118, China
2 Jilin Province Agricultural Internet of Things Technology Collaborative Innovation Center, Changchun, 130118, China
3 Jilin Province Intelligent Environmental Engineering Research Center, Changchun, 130118, China
4 Jilin Province Information Technology and Intelligent Agriculture Engineering Research Center, Changchun, 130118, China
5 College of Engineering and Technology, Jilin Agricultural University, Changchun, 130118, China
6 Department of Agricultural Economics and Animal Production, University of Limpopo, Sovenga, 0727, Polokwane, South Africa
7 College of Information Technology, Wuzhou University, Wuzhou, 543003, China
8 Guangxi Key Laboratory of Machine Vision and Intelligent Control, Wuzhou, 543003, China
* Corresponding Author: Li Shijun. Email:
Computers, Materials & Continua 2022, 73(3), 6195-6205. https://doi.org/10.32604/cmc.2022.026880
Received 06 January 2022; Accepted 24 March 2022; Issue published 28 July 2022
Abstract
Remote sensing image analysis is a basic and practical research hotspot in remote sensing science. Remote sensing images contain abundant ground object information and it can be used in urban planning, agricultural monitoring, ecological services, geological exploration and other aspects. In this paper, we propose a lightweight model combining vgg-16 and u-net network. By combining two convolutional neural networks, we classify scenes of remote sensing images. While ensuring the accuracy of the model, try to reduce the memory of the model. According to the experimental results of this paper, we have improved the accuracy of the model to 98%. The memory size of the model is 3.4 MB. At the same time, The classification and convergence speed of the model are greatly improved. We simultaneously take the remote sensing scene image of 64 × 64 as input into the designed model. As the accuracy of the model is 97%, it is proved that the model designed in this paper is also suitable for remote sensing images with few target feature points and low accuracy. Therefore, the model has a good application prospect in the classification of remote sensing images with few target feature points and low pixels.Keywords
Cite This Article
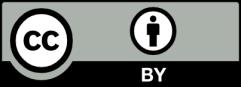
This work is licensed under a Creative Commons Attribution 4.0 International License , which permits unrestricted use, distribution, and reproduction in any medium, provided the original work is properly cited.